Personalized Query Suggestions
SIGIR '20: The 43rd International ACM SIGIR conference on research and development in Information Retrieval Virtual Event China July, 2020(2020)
摘要
With the exponential growth of information on the internet, users have been relying on search engines for finding the precise documents. However, user queries are often short. The inherent ambiguity of short queries imposes great challenges for search engines to understand user intent. Query suggestion is one key technique for search engines to augment user queries so that they can better understand user intent. In the past, query suggestions have been relying on either term-frequency--based methods with little semantic understanding of the query, or word-embedding--based methods with little personalization efforts. Here, we present a sequence-to-sequence-model--based query suggestion framework that is capable of modeling structured, personalized features and unstructured query texts naturally. This capability opens up the opportunity to better understand query semantics and user intent at the same time. As the largest professional network, LinkedIn has the advantage of utilizing a rich amount of accurate member profile information to personalize query suggestions. We applied this framework in the LinkedIn production traffic and showed that personalized query suggestions significantly improved member search experience as measured by key business metrics at LinkedIn.
更多查看译文
关键词
Query suggestion, Sequence-to-sequence model, Personalization, Deep learning model deployment
AI 理解论文
溯源树
样例
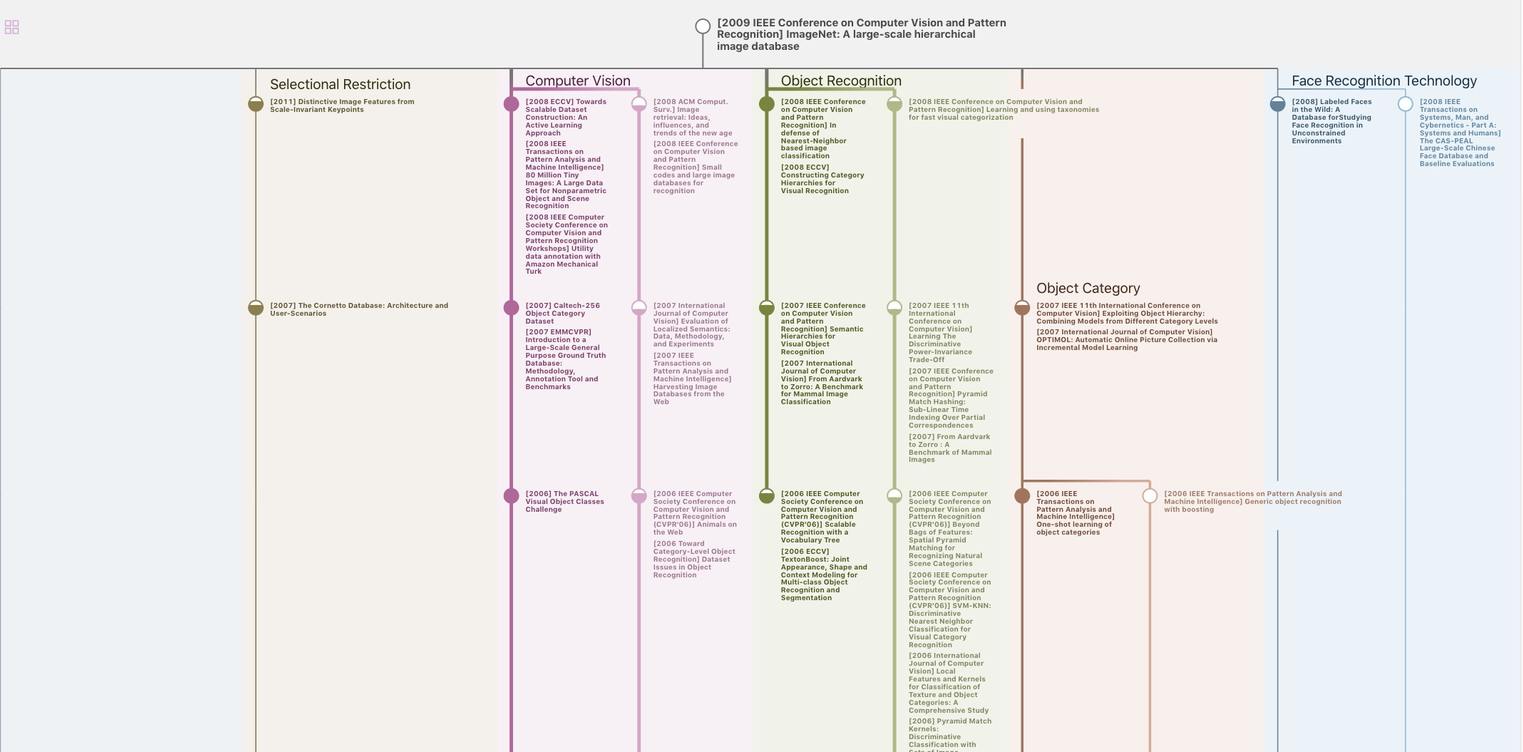
生成溯源树,研究论文发展脉络
Chat Paper
正在生成论文摘要