Reinforcement learning with analogue memristor arrays
NATURE ELECTRONICS(2019)
摘要
Reinforcement learning algorithms that use deep neural networks are a promising approach for the development of machines that can acquire knowledge and solve problems without human input or supervision. At present, however, these algorithms are implemented in software running on relatively standard complementary metal–oxide–semiconductor digital platforms, where performance will be constrained by the limits of Moore’s law and von Neumann architecture. Here, we report an experimental demonstration of reinforcement learning on a three-layer 1-transistor 1-memristor (1T1R) network using a modified learning algorithm tailored for our hybrid analogue–digital platform. To illustrate the capabilities of our approach in robust in situ training without the need for a model, we performed two classic control problems: the cart–pole and mountain car simulations. We also show that, compared with conventional digital systems in real-world reinforcement learning tasks, our hybrid analogue–digital computing system has the potential to achieve a significant boost in speed and energy efficiency.
更多查看译文
关键词
Computer science,Electronic devices,Information technology,Learning algorithms,Network models,Electrical Engineering
AI 理解论文
溯源树
样例
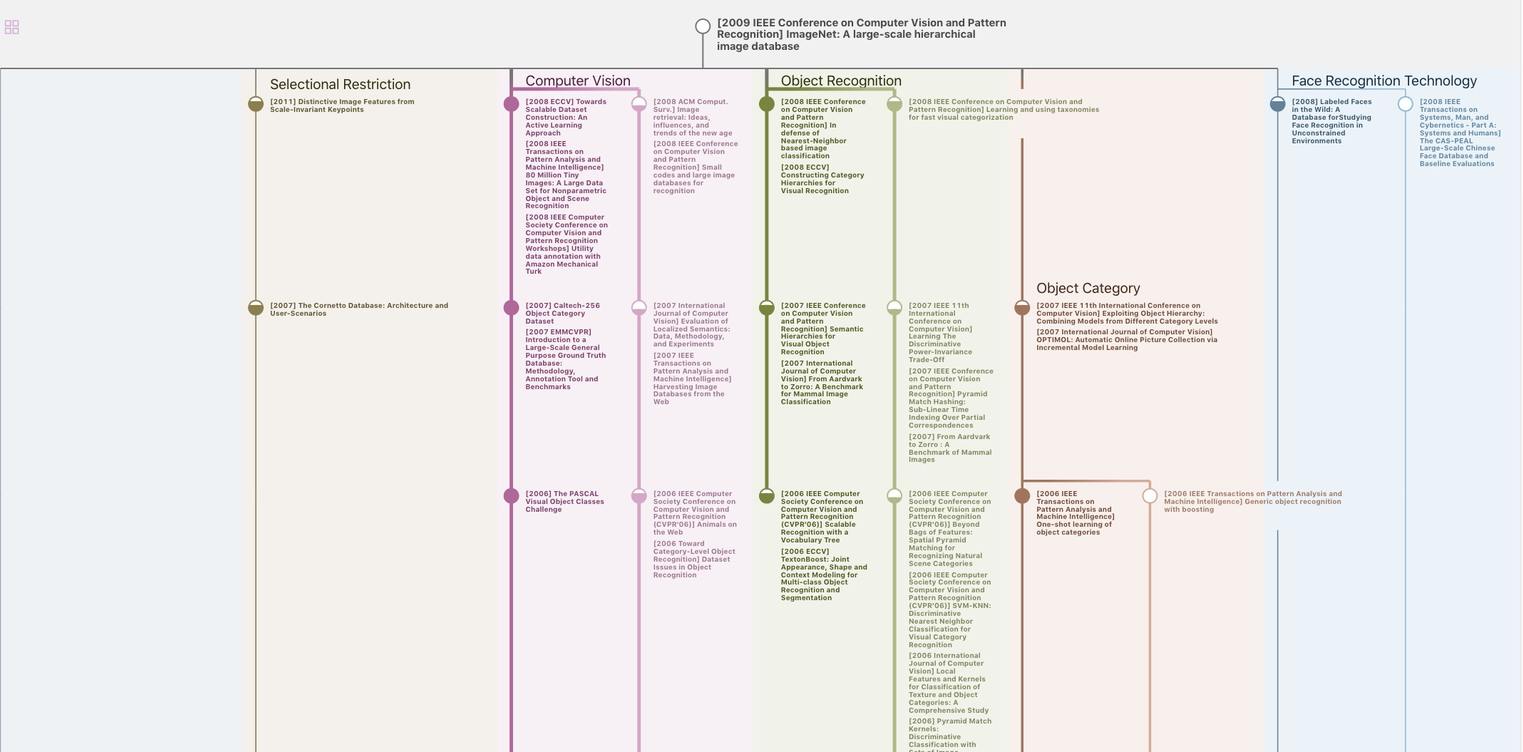
生成溯源树,研究论文发展脉络
Chat Paper
正在生成论文摘要