Bayesian Non-Parametric Ordinal Regression Under a Monotonicity Constraint
arxiv(2023)
摘要
Compared to the nominal scale, the ordinal scale for a categorical out-come variable has the property of making a monotonicity assumption for the co-variate effects meaningful. This assumption is encoded in the commonly used pro-portional odds model, but there it is combined with other parametric assumptions such as linearity and additivity. Herein, the considered models are non-parametric and the only condition imposed is that the effects of the covariates on the outcome categories are stochastically monotone according to the ordinal scale. We are not aware of the existence of other comparable multivariable models that would be suitable for inference purposes. We generalize our previously proposed Bayesian monotonic multivariable regression model to ordinal outcomes, and propose an estimation procedure based on reversible jump Markov chain Monte Carlo. The model is based on a marked point process construction, which allows it to approx-imate arbitrary monotonic regression function shapes, and has a built-in covariate selection property. We study the performance of the proposed approach through extensive simulation studies, and demonstrate its practical application in two real data examples.
更多查看译文
关键词
monotonic regression,non-parametric Bayesian regression,ordinal regression,proportional odds model
AI 理解论文
溯源树
样例
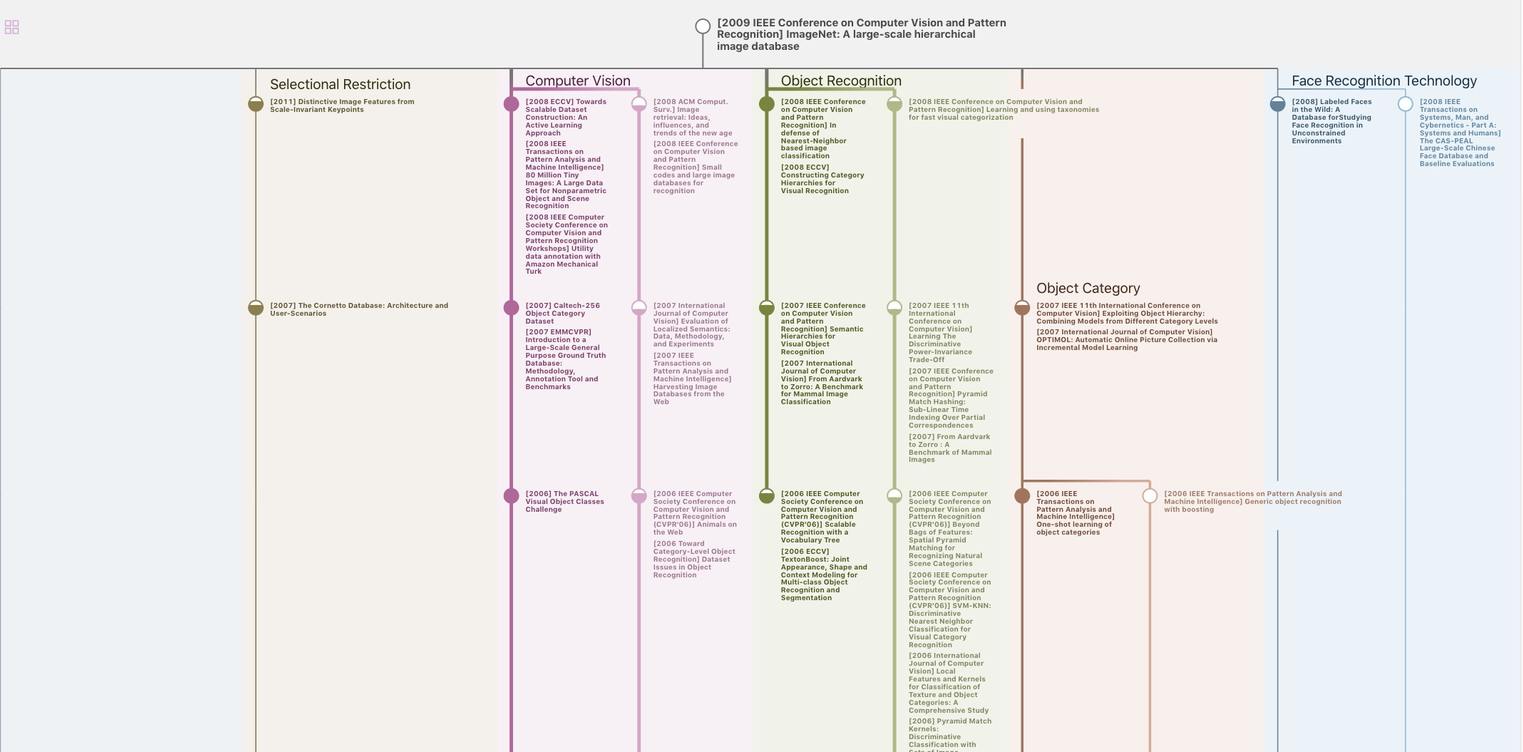
生成溯源树,研究论文发展脉络
Chat Paper
正在生成论文摘要