Multiagent Low-Dimensional Linear Bandits
arxiv(2023)
摘要
We study a multiagent stochastic linear bandit with side information, parameterized by an unknown vector 0(*) ? R-d. The side information consists of a finite collection of low-dimensional subspaces, one of which contains 0(*). In our setting, agents can collaborate to reduce regret by sending recommendations across a communication graph connecting them. We present a novel decentralized algorithm, where agents communicate subspace indices with each other and each agent plays a projected variant of LinUCB on the corresponding (low dimensional) subspace. By distributing the search for the optimal subspace across users and learning of the unknown vector by each agent in the corresponding low-dimensional subspace, we show that the per-agent finite-time regret is much smaller than the case when agents do not communicate. We finally complement these results through simulations.
更多查看译文
关键词
Decentralized learning,gossip,linear bandits,networks,regret minimization
AI 理解论文
溯源树
样例
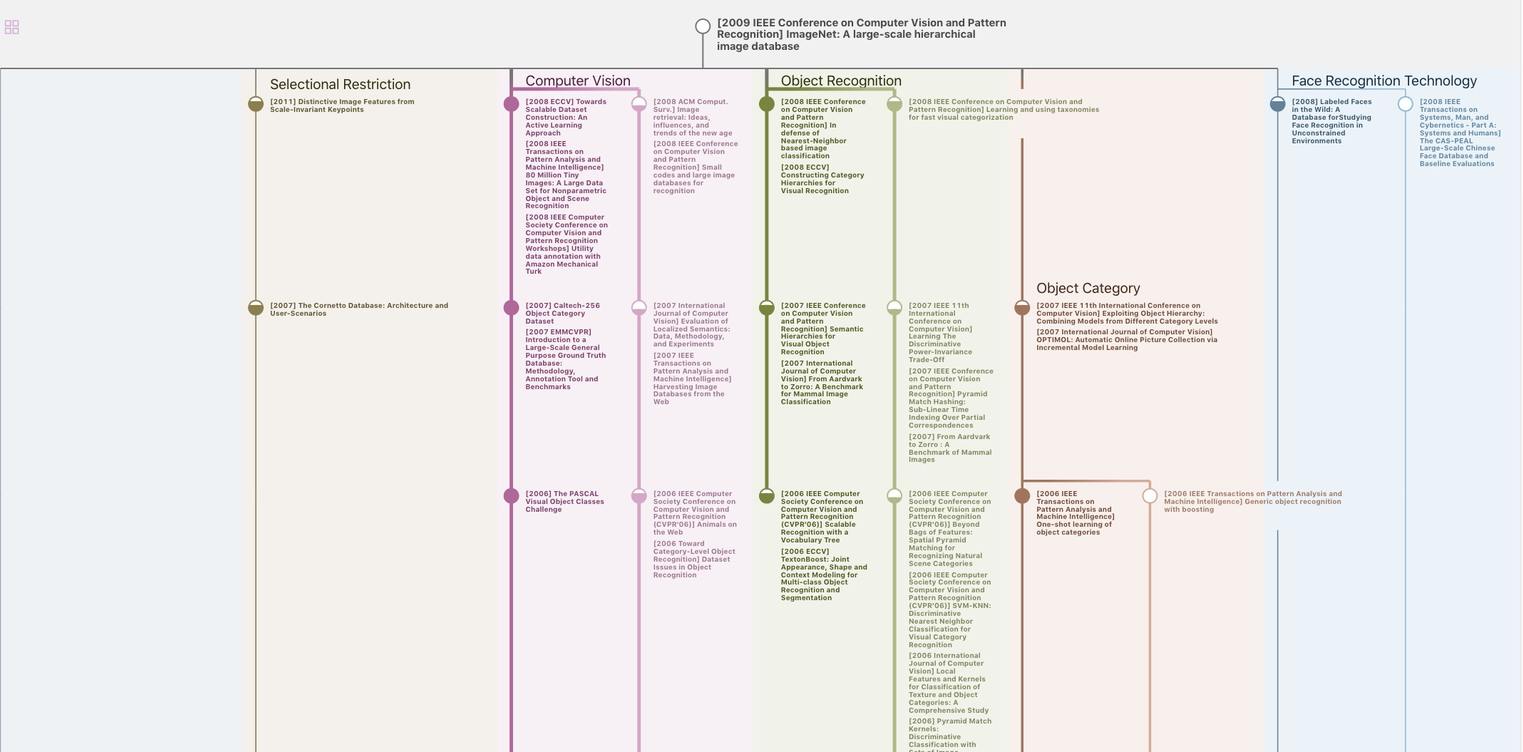
生成溯源树,研究论文发展脉络
Chat Paper
正在生成论文摘要