ODE-CNN: Omnidirectional Depth Extension Networks
arxiv(2020)
摘要
Omnidirectional 360{\deg} camera proliferates rapidly for autonomous robots since it significantly enhances the perception ability by widening the field of view(FoV). However, corresponding 360{\deg} depth sensors, which are also critical for the perception system, are still difficult or expensive to have. In this paper, we propose a low-cost 3D sensing system that combines an omnidirectional camera with a calibrated projective depth camera, where the depth from the limited FoV can be automatically extended to the rest of the recorded omnidirectional image. To accurately recover the missing depths, we design an omnidirectional depth extension convolutional neural network(ODE-CNN), in which a spherical feature transform layer(SFTL) is embedded at the end of feature encoding layers, and a deformable convolutional spatial propagation network(D-CSPN) is appended at the end of feature decoding layers. The former resamples the neighborhood of each pixel in the omnidirectional coordination to the projective coordination, which reduces the difficulty of feature learning, and the later automatically finds a proper context to well align the structures in the estimated depths via CNN w.r.t. the reference image, which significantly improves the visual quality. Finally, we demonstrate the effectiveness of proposed ODE-CNN over the popular 360D dataset and show that ODE-CNN significantly outperforms (relatively 33% reduction in-depth error) other state-of-the-art (SoTA) methods.
更多查看译文
关键词
omnidirectional depth extension networks,omnidirectional 360° camera,autonomous robots,perception ability,FoV,depth sensors,perception system,low-cost 3D sensing system,omnidirectional camera,calibrated projective depth camera,recorded omnidirectional image,omnidirectional depth extension convolutional neural network,spherical feature,feature encoding layers,deformable convolutional spatial propagation network,feature decoding layers,omnidirectional coordination,projective coordination,feature learning,estimated depths,proposed ODE-CNN,popular 360D dataset,depth error
AI 理解论文
溯源树
样例
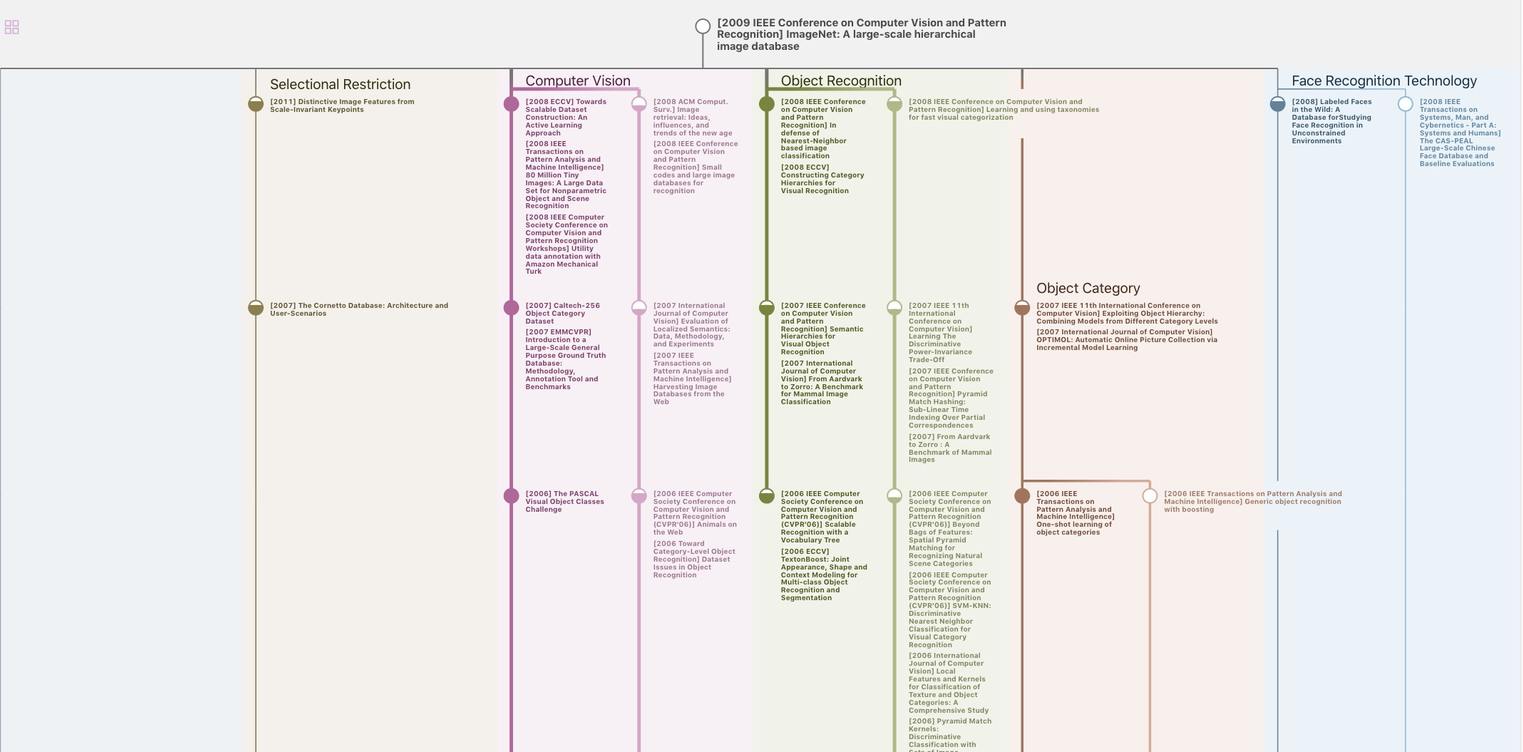
生成溯源树,研究论文发展脉络
Chat Paper
正在生成论文摘要