Ground truth free denoising by optimal transport
NUMERICAL ALGEBRA CONTROL AND OPTIMIZATION(2024)
摘要
This paper proposes a new training strategy for a denoiser re-moving (additive) independent noise, with only as readily available data as possible and no further assumptions on the data nor noise. While every real -world measurement contains some noise, it seems that this problem remains unsolved for settings where clean data samples are lacking. We propose a push -forward operator formulation of an ideal denoiser and a corresponding GAN setup for training a denoiser ground truth free. The GAN trains solely on sam-ples of noisy data and noise. In a series of denoising experiments in 1D and 2D, we demonstrate our training strategy's performance, which significantly improves the state-of-the-art of unsupervised denoising. Moreover, for some non-Gaussian noise, the method compares favorably even to naive supervised denoising.
更多查看译文
关键词
Deep learning,denoising,inverse problem,optimal transport,ground-truth free,adversarial training
AI 理解论文
溯源树
样例
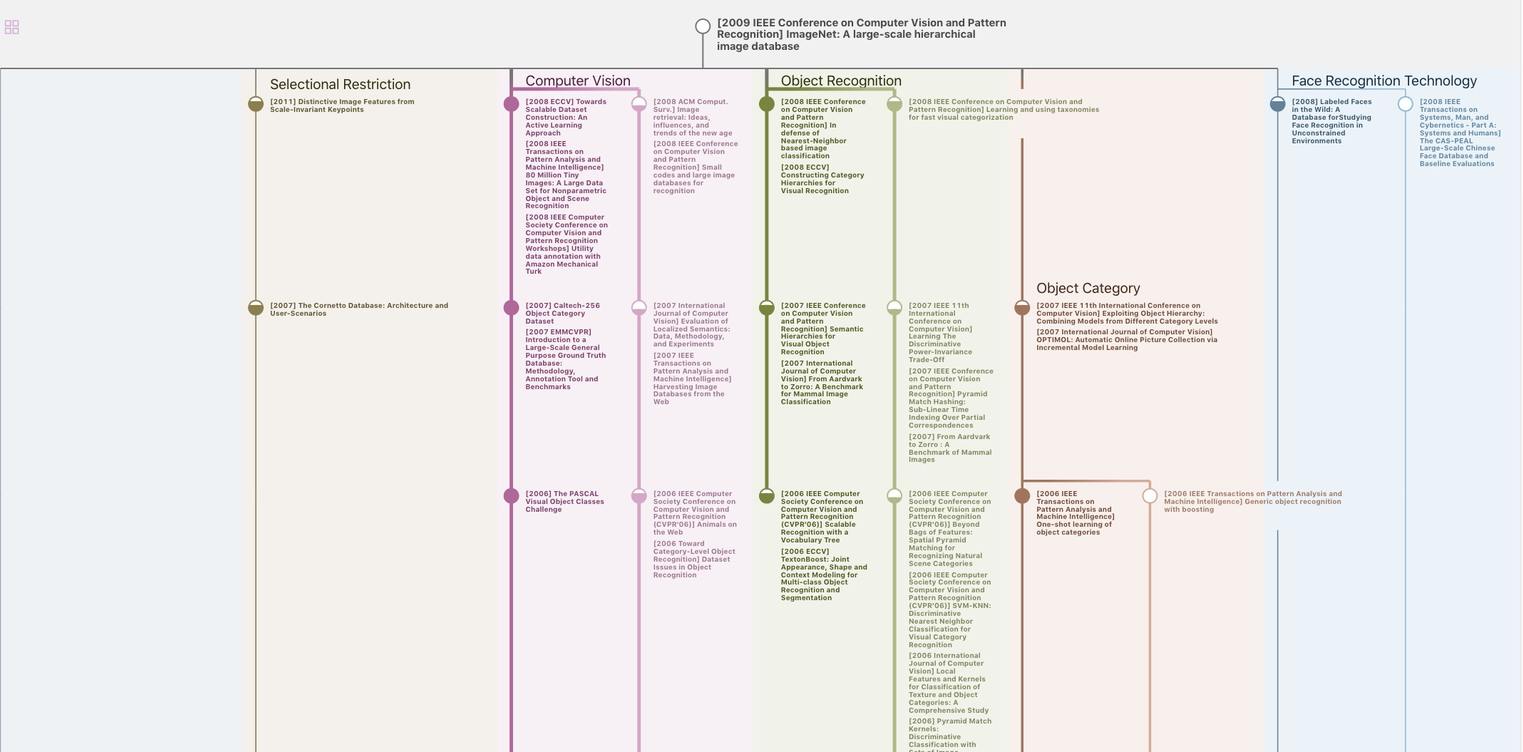
生成溯源树,研究论文发展脉络
Chat Paper
正在生成论文摘要