Weakly Supervised Temporal Action Localization with Segment-Level Labels
PATTERN RECOGNITION AND COMPUTER VISION, PT I(2020)
摘要
Temporal action localization presents a trade-off between test performance and annotation-time cost. Fully supervised methods achieve good performance with time-consuming boundary annotations. Weakly supervised methods with cheaper video-level category label annotations result in worse performance. In this paper, we introduce a new segment-level supervision setting: segments are labeled when annotators observe actions happening here. We incorporate this segment-level supervision along with a novel localization module in the training. Specifically, we devise a partial segment loss regarded as a loss sampling to learn integral action parts from labeled segments. Since the labeled segments are only parts of actions, the model tends to overfit along with the training process. To tackle this problem, we first obtain a similarity matrix from discriminative features guided by a sphere loss. Then, a propagation loss is devised based on the matrix to act as a regularization term, allowing implicit unlabeled segments propagation during training. Experiments validate that our method can outperform the video-level supervision methods with almost same the annotation time.
更多查看译文
关键词
Temporal action localization,Weak supervision,Regularization
AI 理解论文
溯源树
样例
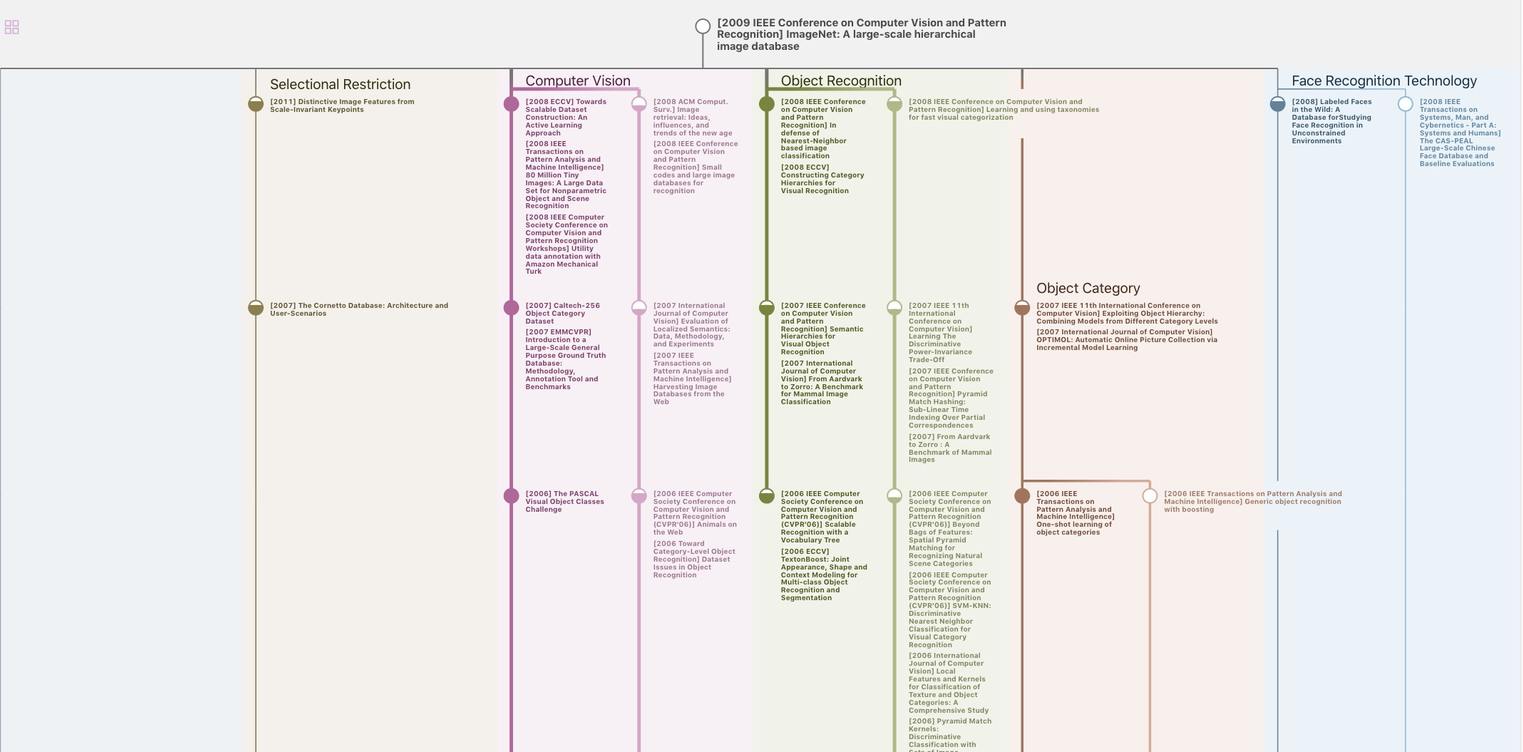
生成溯源树,研究论文发展脉络
Chat Paper
正在生成论文摘要