Joint estimation of low-rank components and connectivity graph in high-dimensional graph signals: Application to brain imaging
SIGNAL PROCESSING(2021)
摘要
Given high-dimensional, graph-smooth and grossly-corrupted signals, this paper presents the algorithm to simultaneously estimate the intrinsic low-rank components and the underlying graph from high dimensional noisy graph signal. We assume that the perturbation on low-rank components is sparse and signals are smooth on unknown underlying graph. The proposed algorithm learns the low-rank components by exploiting estimate of the graph, and refines the graph estimation with learned low-rank components. We propose two solutions to this problem: One applies alternating optimization to solve the subproblems. The other solves the problem directly. Furthermore, we analyze the impact of inexact graph on low-rank components estimation to justify our approach. We conduct extensive experiments on the proposed algorithm with synthetic data and real brain imaging data, Magnetoencephalography (MEG) and compare it with state-of-the-art methods. In particular, our algorithm is applied to estimate the low rank components for classifying MEG signals evoked when a subject views a face or non-face image. We observe that our proposed algorithm is competitive in estimating low-rank components, adequately capturing intrinsic task-related information in lower-dimensional representation. This leads to improved classification performance. Furthermore, we notice that our estimated graph indicates brain active regions for the visual activity that are consistent with neuroscientific findings. (c) 2020 Elsevier B.V. All rights reserved.
更多查看译文
关键词
Graph Signal Processing,Low-rank Components Estimation,Dimensionality Reduction,Graph Learning,Brain Imaging,Classification
AI 理解论文
溯源树
样例
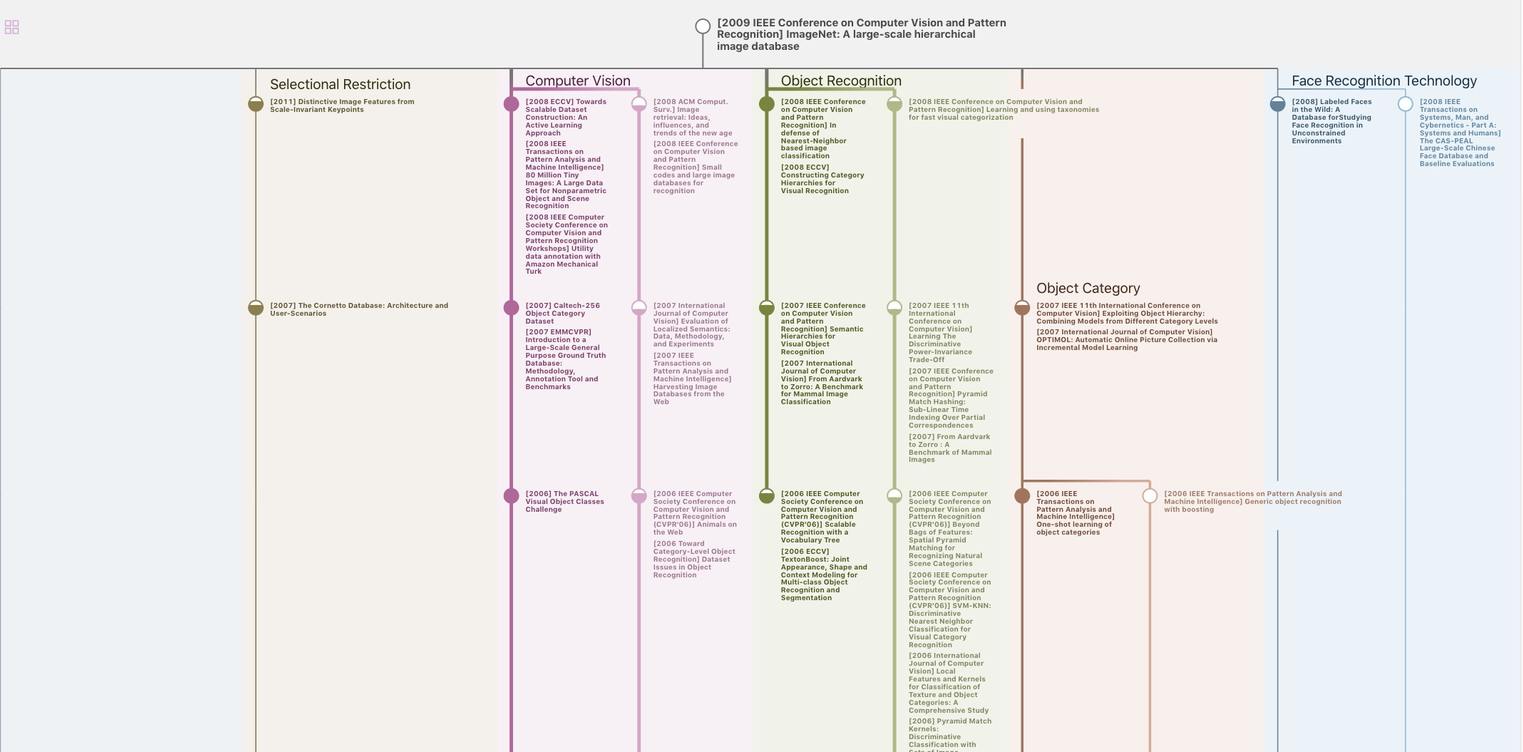
生成溯源树,研究论文发展脉络
Chat Paper
正在生成论文摘要