Evaluating Fairness using Permutation Tests
KDD '20: The 26th ACM SIGKDD Conference on Knowledge Discovery and Data Mining Virtual Event CA USA July, 2020(2020)
摘要
Machine learning models are central to people's lives and impact society in ways as fundamental as determining how people access information. The gravity of these models imparts a responsibility to model developers to ensure that they are treating users in a fair and equitable manner. Before deploying a model into production, it is crucial to examine the extent to which its predictions demonstrate biases. This paper deals with the detection of bias exhibited by a machine learning model through statistical hypothesis testing. We propose a permutation testing methodology that performs a hypothesis test that a model is fair across two groups with respect to any given metric. There are increasingly many notions of fairness that can speak to different aspects of model fairness. Our aim is to provide a flexible framework that empowers practitioners to identify significant biases in any metric they wish to study. We provide a formal testing mechanism as well as extensive experiments to show how this method works in practice.
更多查看译文
关键词
Permutation tests, Fairness, Asymptotics
AI 理解论文
溯源树
样例
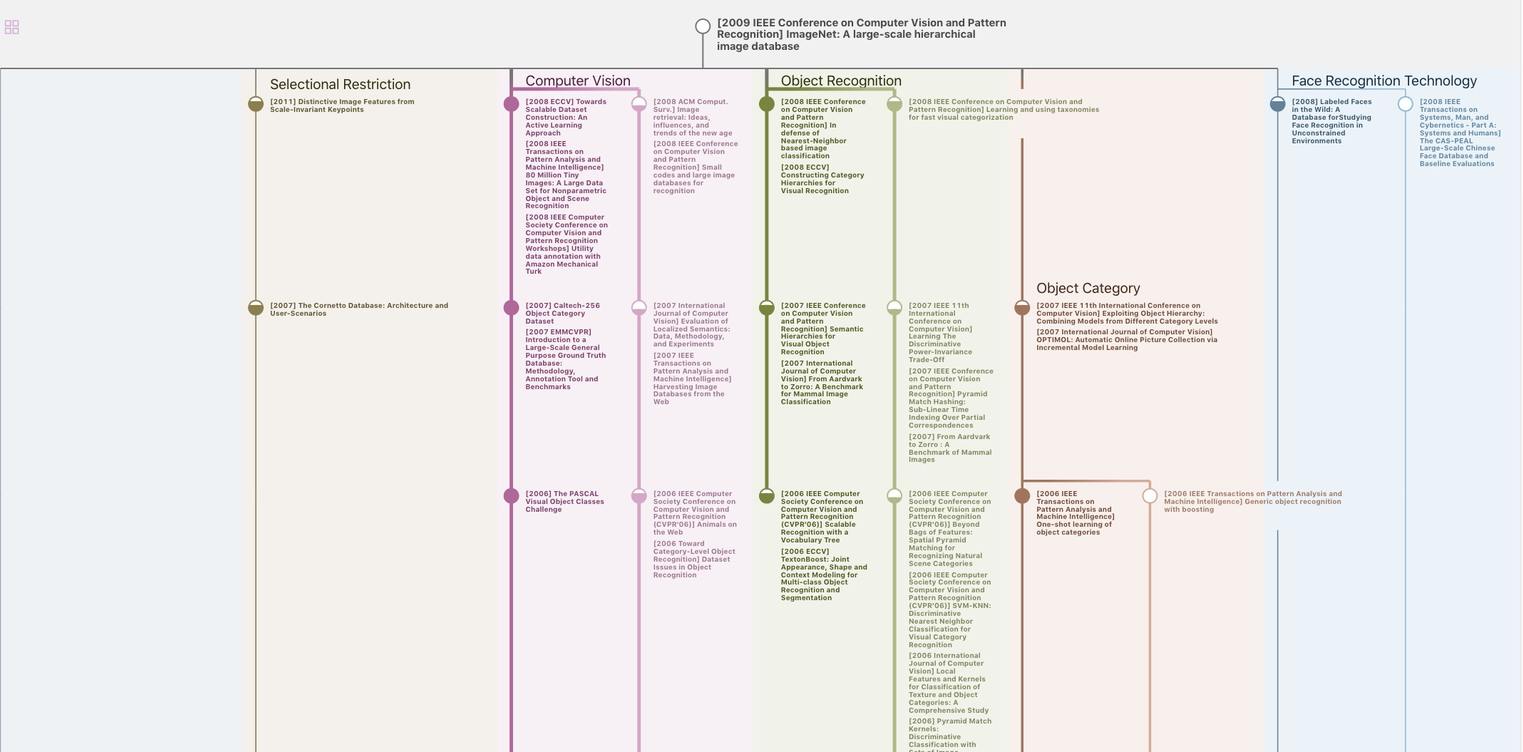
生成溯源树,研究论文发展脉络
Chat Paper
正在生成论文摘要