Feature-Induced Manifold Disambiguation for Multi-View Partial Multi-label Learning
KDD '20: The 26th ACM SIGKDD Conference on Knowledge Discovery and Data Mining Virtual Event CA USA July, 2020(2020)
摘要
In conventional multi-label learning framework, each example is assumed to be represented by a single feature vector and associated with multiple valid labels simultaneously. Nonetheless, real-world objects usually exhibit complicated properties which can have multi-view feature representation as well as false positive labeling. Accordingly, the problem of multi-view partial multi-label learning (MVPML) is studied in this paper, where each example is assumed to be presented by multiple feature vectors while associated with multiple candidate labels which are only partially valid. To learn from MVPML examples, a novel approach named FIMAN is proposed which makes use of multi-view feature representation to tackle the noisy labeling information. Firstly, an aggregate manifold structure over training examples is generated by adaptively fusing affinity information conveyed by feature vectors of different views. Then, candidate labels of each training example are disambiguated by preserving the feature-induced manifold structure in label space. Finally, the resulting predictive models are learned by fitting modeling outputs with the disambiguated labels. Extensive experiments on a number of real-world data sets show that FIMAN achieves highly competitive performance against state-of-the-art approaches in solving the MVPML problem.
更多查看译文
关键词
Multi-label learning, partial label learning, multi-view, disambiguation
AI 理解论文
溯源树
样例
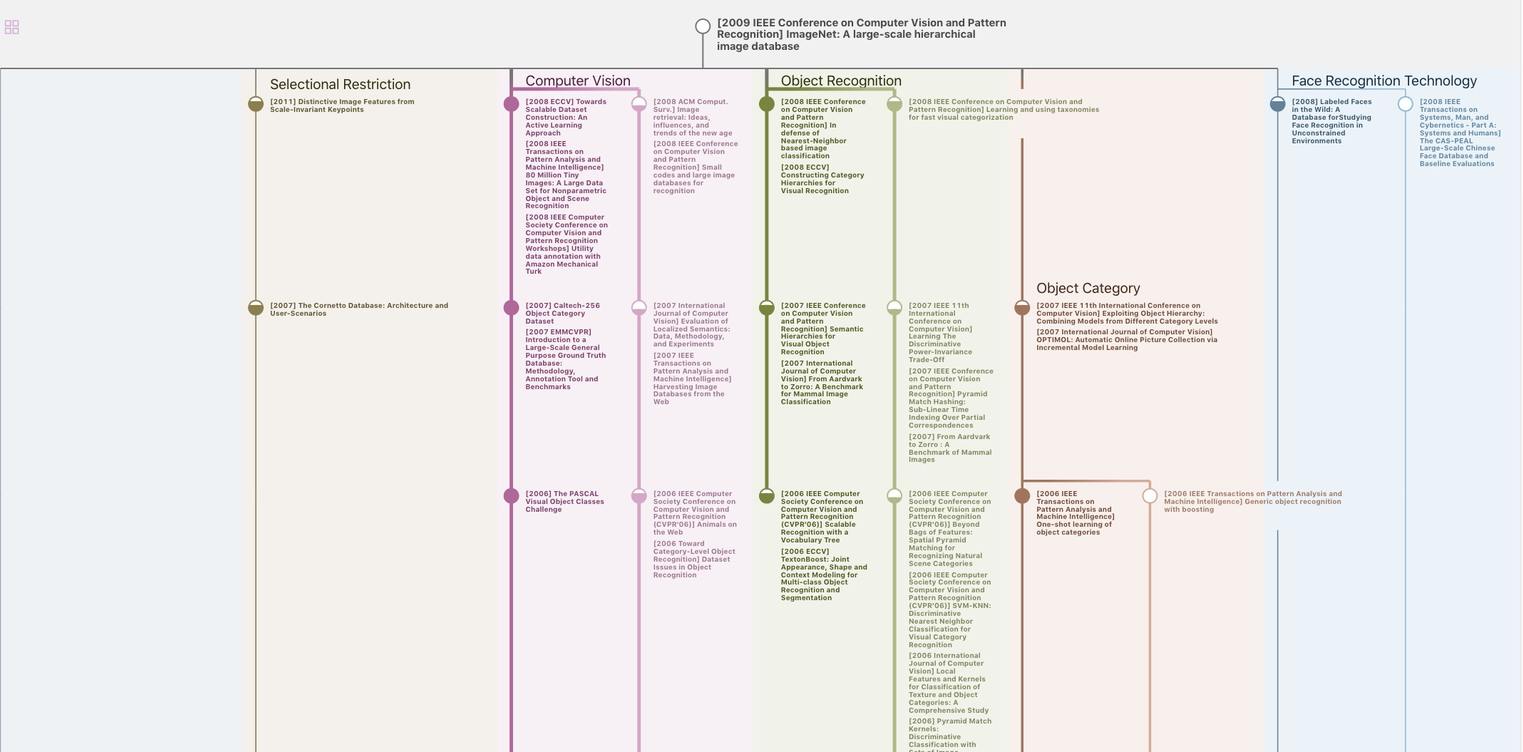
生成溯源树,研究论文发展脉络
Chat Paper
正在生成论文摘要