Prediction and Profiling of Audience Competition for Online Television Series
KDD '20: The 26th ACM SIGKDD Conference on Knowledge Discovery and Data Mining Virtual Event CA USA July, 2020(2020)
摘要
Understanding the target audience for popular television series is valuable for online video platform to manage advertising sales, purchase video copyrights, and compete with other video service platforms. Existing studies in this domain generally focus on using data mining and machine learning techniques to recommend television series to individual users or predict the popularity of television series. Knowing only the popularity of television series may, however, limit our ability to answer more in-depth questions and develop more intelligent applications. In this paper, we develop a data-driven framework to model and predict audience competition patterns for popular online television series. Specifically, we first construct a sequence of dynamic competition networks of television series by mining the detailed viewership records. Then, we design the Dynamic Deep Network Factorization (DDNF), a hybrid modeling framework for predicting the future competition networks. Our framework adopts the deep neural network (DNN) and the knowledge-base (KB) embedding to incorporate static features, and integrates the Long Short-Term Memory (LSTM) network to learn dynamic features of the television series. Finally, extensive experiments on real-world data sets validate the effectiveness of our approach compared with state-of-the-art baselines in predicting the audience competition for existing and new television series.
更多查看译文
关键词
Audience Competition, Network Prediction, Network Factorization, Online Television Series
AI 理解论文
溯源树
样例
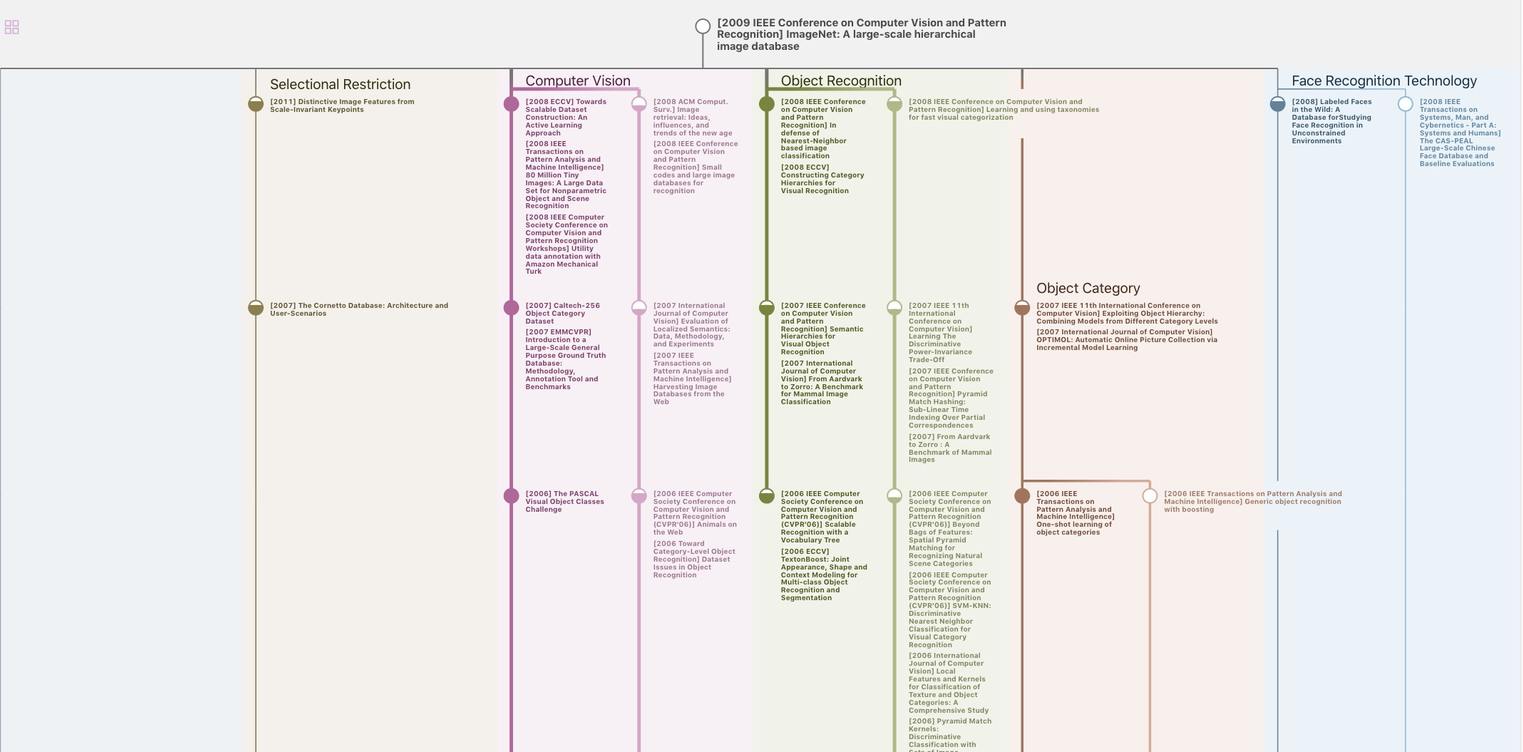
生成溯源树,研究论文发展脉络
Chat Paper
正在生成论文摘要