Towards Fair Truth Discovery from Biased Crowdsourced Answers
KDD '20: The 26th ACM SIGKDD Conference on Knowledge Discovery and Data Mining Virtual Event CA USA July, 2020(2020)
摘要
Crowdsourcing systems have gained considerable interest and adoption in recent years. One important research problem for crowdsourcing systems is truth discovery, which aims to aggregate noisy answers contributed by the workers to obtain the correct answer (truth) of each task. However, since the collected answers are highly prone to the workers' biases, aggregating these biased answers without proper treatment will unavoidably lead to discriminatory truth discovery results for particular race, gender and political groups. To address this challenge, in this paper, first, we define a new fairness notion named θ-disparity for truth discovery. Intuitively, θ-disparity bounds the difference in the probabilities that the truth of both protected and unprotected groups being predicted to be positive. Second, we design three fairness enhancing methods, namely Pre-TD, FairTD, and Post-TD, for truth discovery. Pre-TD is a pre-processing method that removes the bias in workers' answers before truth discovery. FairTD is an in-processing method that incorporates fairness into the truth discovery process. And Post-TD is a post-processing method that applies additional treatment on the discovered truth to make it satisfy θ-disparity. We perform an extensive set of experiments on both synthetic and real-world crowdsourcing datasets. Our results demonstrate that among the three fairness enhancing methods, FairTD produces the best accuracy with θ-disparity. In some settings, the accuracy of FairTD is even better than truth discovery without fairness, as it removes some low-quality answers as side effects.
更多查看译文
关键词
Truth discovery, algorithmic fairness, crowdsourcing systems
AI 理解论文
溯源树
样例
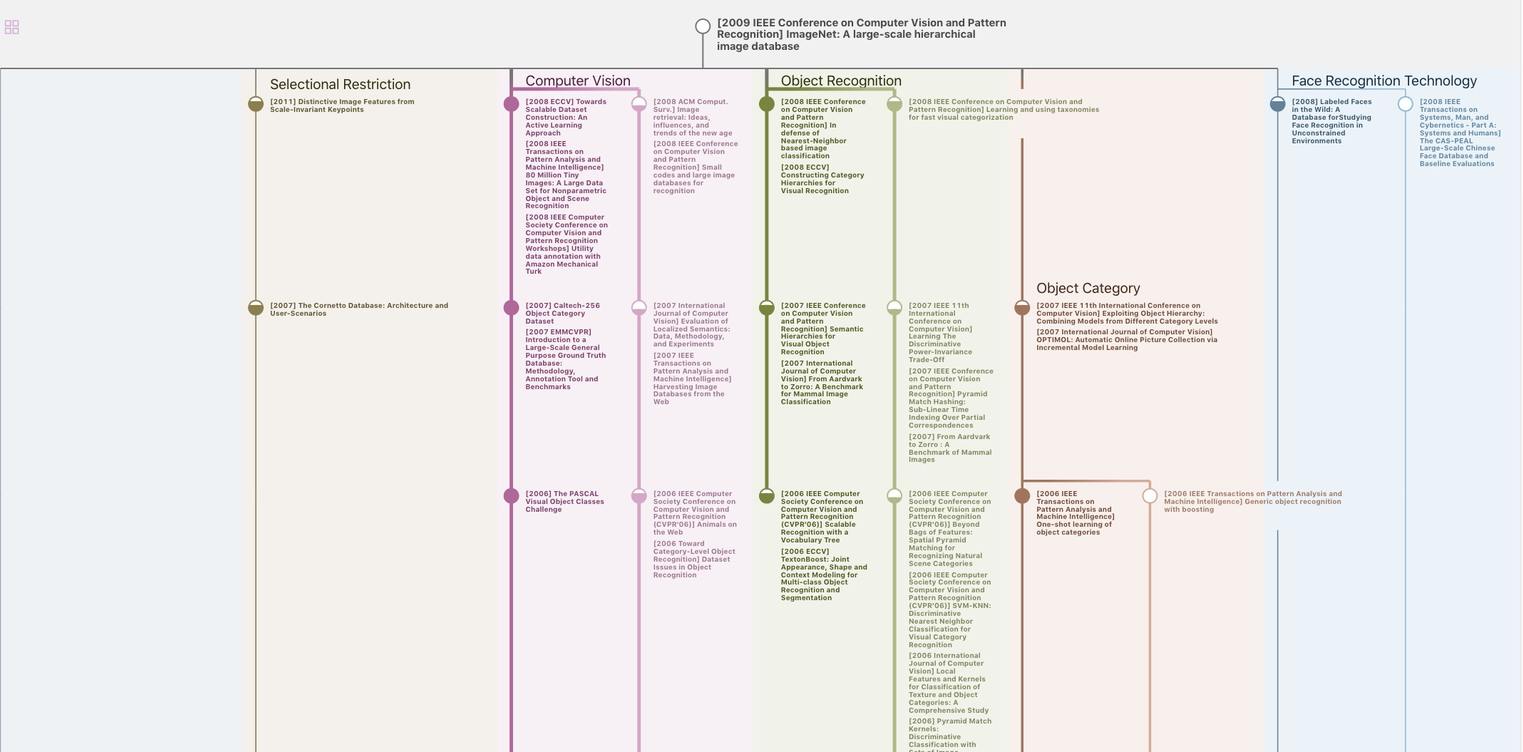
生成溯源树,研究论文发展脉络
Chat Paper
正在生成论文摘要