Contrastive Learning for Debiased Candidate Generation in Large-Scale Recommender Systems
Knowledge Discovery and Data Mining(2021)
摘要
ABSTRACTDeep candidate generation (DCG) that narrows down the collection of relevant items from billions to hundreds via representation learning has become prevalent in industrial recommender systems. Standard approaches approximate maximum likelihood estimation (MLE) through sampling for better scalability and address the problem of DCG in a way similar to language modeling. However, live recommender systems face severe exposure bias and have a vocabulary several orders of magnitude larger than that of natural language, implying that MLE will preserve and even exacerbate the exposure bias in the long run in order to faithfully fit the observed samples. In this paper, we theoretically prove that a popular choice of contrastive loss is equivalent to reducing the exposure bias via inverse propensity weighting, which provides a new perspective for understanding the effectiveness of contrastive learning. Based on the theoretical discovery, we design CLRec, a contrastive learning method to improve DCG in terms of fairness, effectiveness and efficiency in recommender systems with extremely large candidate size. We further improve upon CLRec and propose Multi-CLRec, for accurate multi-intention aware bias reduction. Our methods have been successfully deployed in Taobao, where at least four-month online A/B tests and offline analyses demonstrate its substantial improvements, including a dramatic reduction in the Matthew effect.
更多查看译文
关键词
Recommender systems, candidate generation, bias reduction, inverse propensityweighting, contrastive learning, negative sampling
AI 理解论文
溯源树
样例
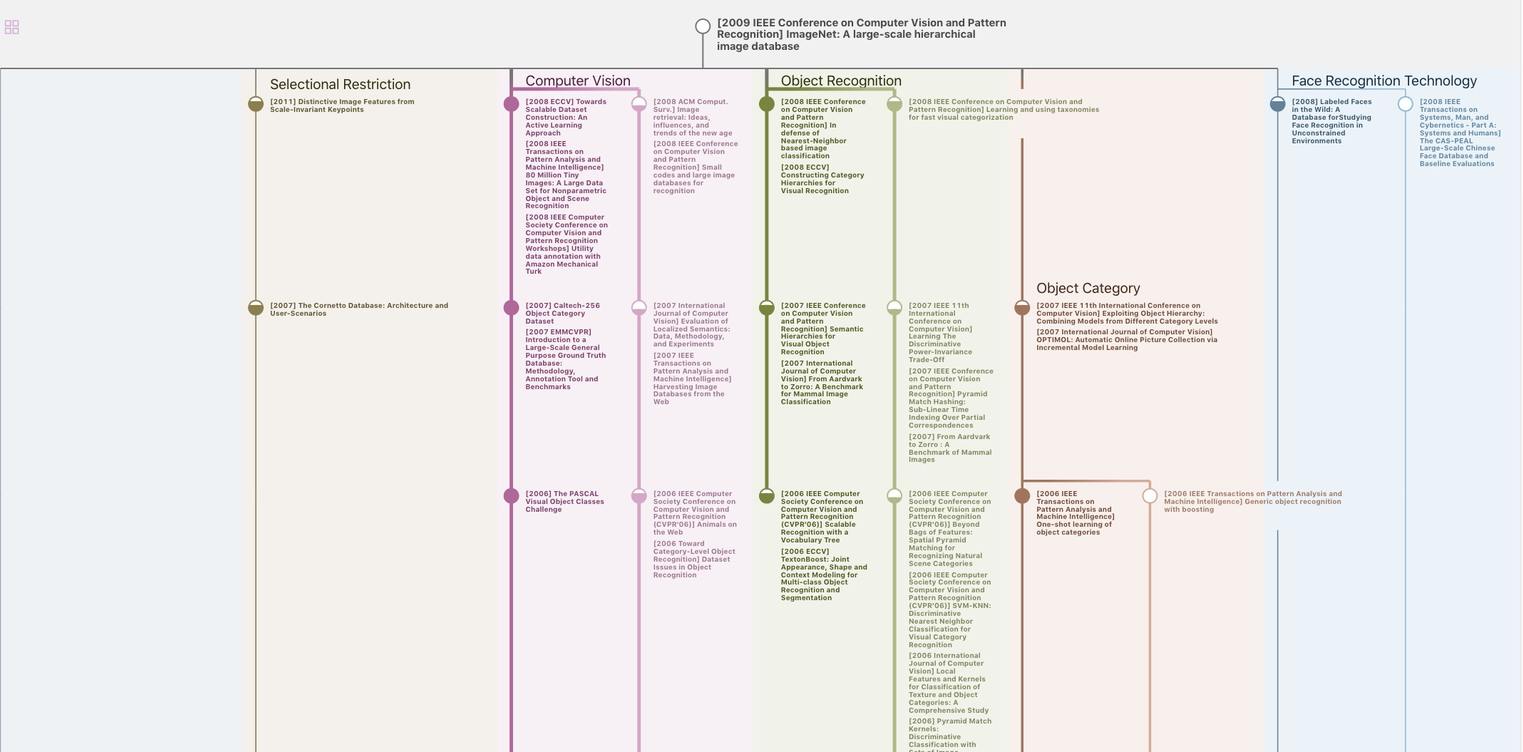
生成溯源树,研究论文发展脉络
Chat Paper
正在生成论文摘要