Computational inference of genome-wide protein-DNA interactions using high-throughput genomic data
user-5efd71244c775ed682ed8a03(2015)
摘要
Transcriptional regulation has been studied intensively in recent decades. One important aspect of this regulation is the interaction between regulatory proteins, such as transcription factors (TF) and nucleosomes, and the genome. Different highthroughput techniques have been invented to map these interactions genome-wide, including ChIP-based methods (ChIP-chip, ChIP-seq, etc.), nuclease digestion methods (DNase-seq, MNase-seq, etc.), and others. However, a single experimental technique often only provides partial and noisy information about the whole picture of protein-DNA interactions. Therefore, the overarching goal of this dissertation is to provide computational developments for jointly modeling different experimental datasets to achieve a holistic inference on the protein-DNA interaction landscape. We first present a computational framework that can incorporate the protein binding information in MNase-seq data into a thermodynamic model of protein-DNA interaction. We use a correlation-based objective function to model the MNaseseq data and a Markov chain Monte Carlo method to maximize the function. Our results show that the inferred protein-DNA interaction landscape is concordant with the MNase-seq data and provides a mechanistic explanation for the experimentally collected MNase-seq fragments. Our framework is flexible and can easily incorporate other data sources. To demonstrate this flexibility, we use prior distributions to integrate experimentally measured protein concentrations. We also study the ability of DNase-seq data to position nucleosomes. Traditioniv
更多查看译文
AI 理解论文
溯源树
样例
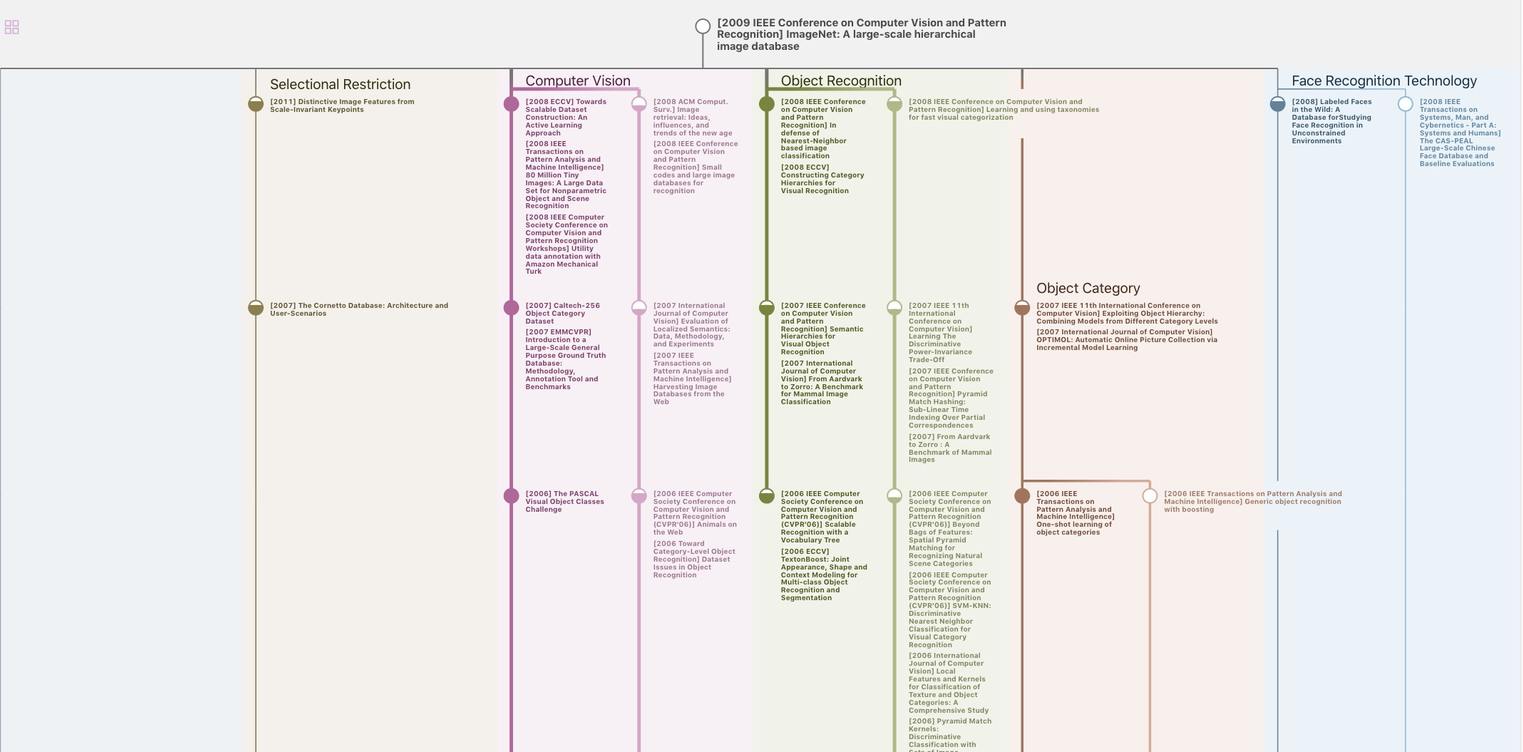
生成溯源树,研究论文发展脉络
Chat Paper
正在生成论文摘要