SMIM: A unified framework of survival sensitivity analysis using multiple imputation and martingale.
Biometrics(2023)
摘要
Censored survival data are common in clinical trial studies. We propose a unified framework for sensitivity analysis to censoring at random in survival data using multiple imputation and martingale, called SMIM. The proposed framework adopts the δ-adjusted and control-based models, indexed by the sensitivity parameter, entailing censoring at random and a wide collection of censoring not at random assumptions. Also, it targets a broad class of treatment effect estimands defined as functionals of treatment-specific survival functions, taking into account missing data due to censoring. Multiple imputation facilitates the use of simple full-sample estimation; however, the standard Rubin's combining rule may overestimate the variance for inference in the sensitivity analysis framework. We decompose the multiple imputation estimator into a martingale series based on the sequential construction of the estimator and propose the wild bootstrap inference by resampling the martingale series. The new bootstrap inference has a theoretical guarantee for consistency and is computationally efficient compared to the nonparametric bootstrap counterpart. We evaluate the finite-sample performance of the proposed SMIM through simulation and an application on an HIV clinical trial.
更多查看译文
关键词
delta adjustment,jump-to-reference,restrictive mean survival time,restrictive mean time loss,wild-bootstrap
AI 理解论文
溯源树
样例
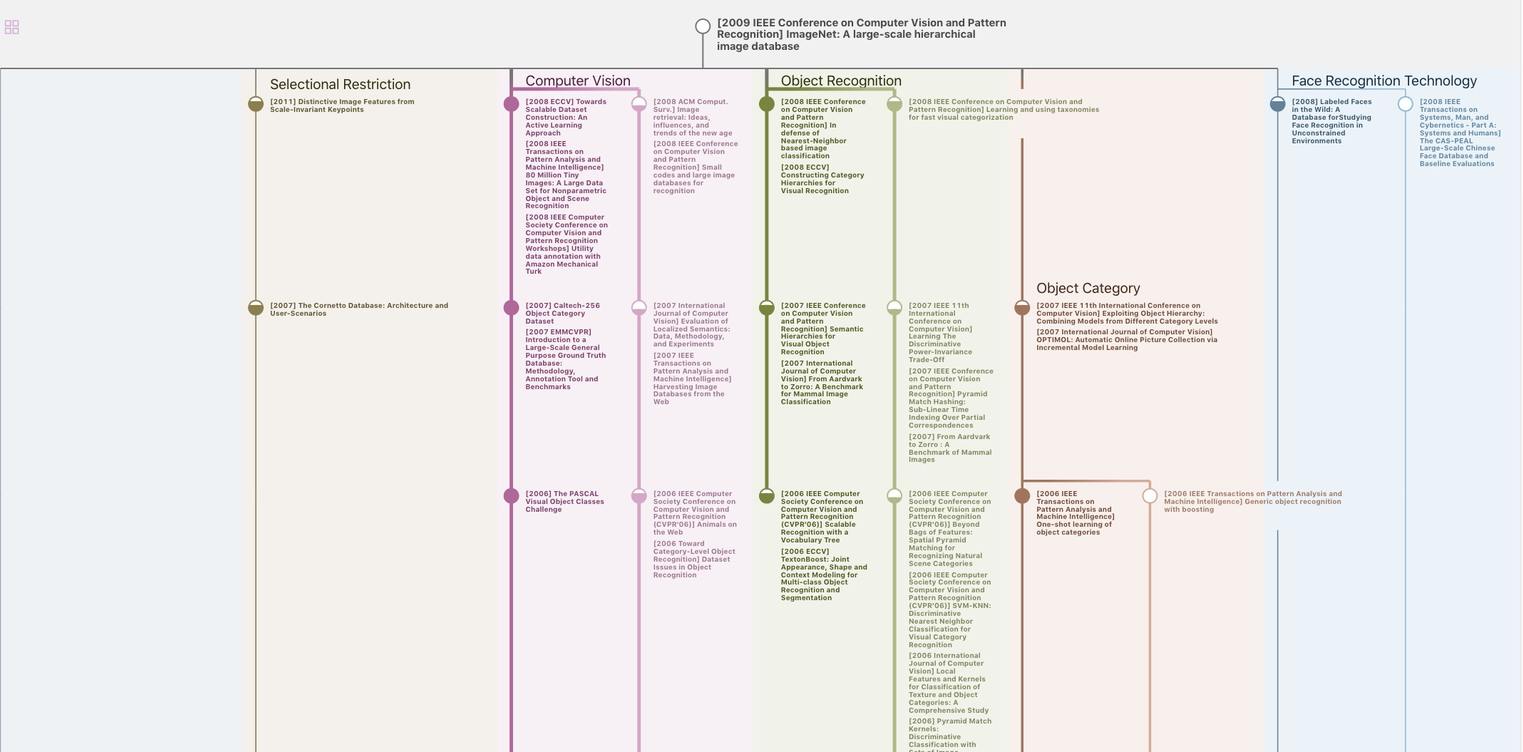
生成溯源树,研究论文发展脉络
Chat Paper
正在生成论文摘要