Meta-Semi: A Meta-learning Approach for Semi-supervised Learning
arxiv(2021)
摘要
Deep learning based semi-supervised learning (SSL) algorithms have led to promising results in recent years. However, they tend to introduce multiple tunable hyper-parameters, making them less practical in real SSL scenarios where the labeled data is scarce for extensive hyper-parameter search. In this paper, we propose a novel meta-learning based SSL algorithm (Meta-Semi) that requires tuning only one additional hyper-parameter, compared with a standard supervised deep learning algorithm, to achieve competitive performance under various conditions of SSL. We start by defining a meta optimization problem that minimizes the loss on labeled data through dynamically reweighting the loss on unlabeled samples, which are associated with soft pseudo labels during training. As the meta problem is computationally intensive to solve directly, we propose an efficient algorithm to dynamically obtain the approximate solutions. We show theoretically that Meta-Semi converges to the stationary point of the loss function on labeled data under mild conditions. Empirically, Meta-Semi outperforms state-of-the-art SSL algorithms significantly on the challenging semi-supervised CIFAR-100 and STL-10 tasks, and achieves competitive performance on CIFAR-10 and SVHN.
更多查看译文
关键词
deep learning,semi-supervised learning,computer vision
AI 理解论文
溯源树
样例
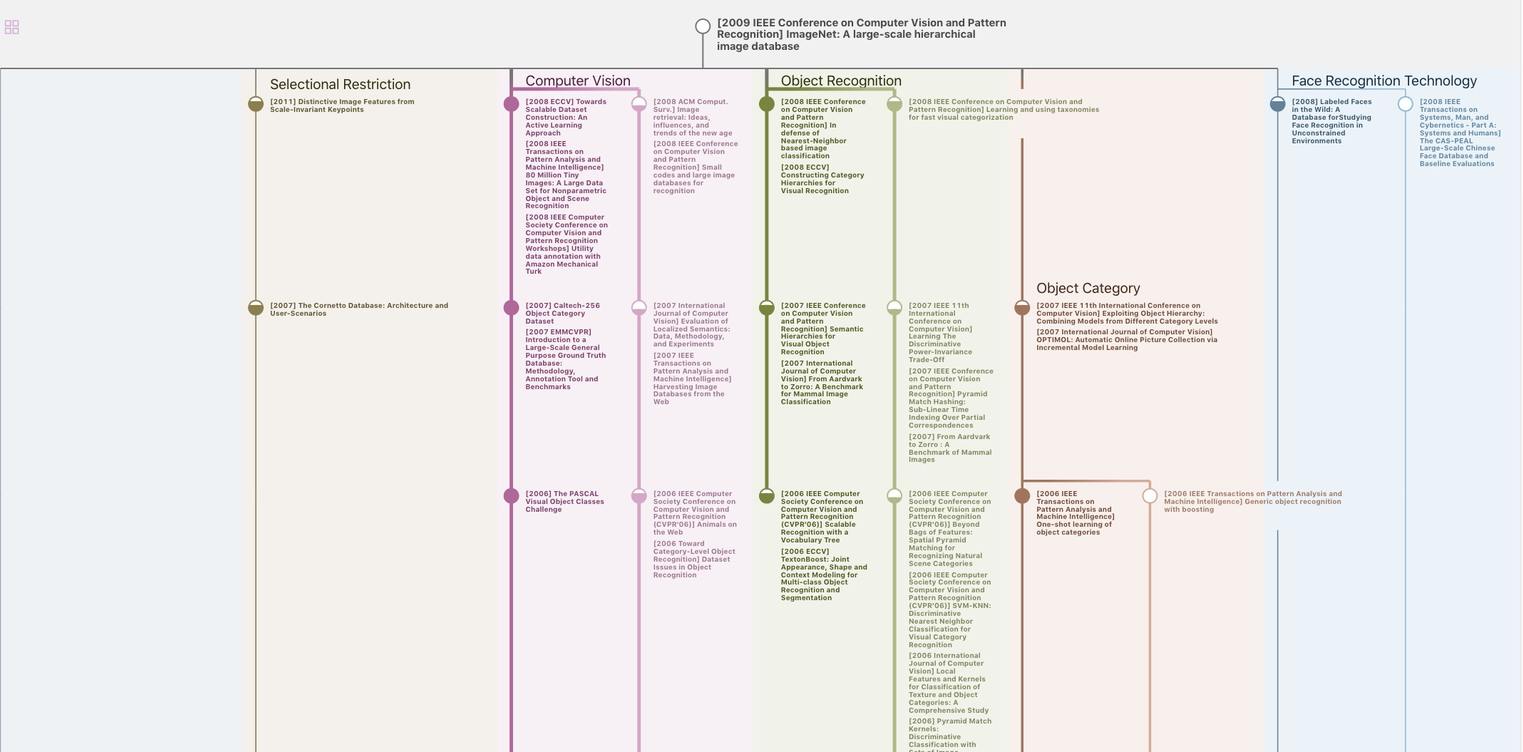
生成溯源树,研究论文发展脉络
Chat Paper
正在生成论文摘要