Explaining Fast Improvement in Online Policy Optimization
arxiv(2020)
摘要
Online policy optimization (OPO) views policy optimization for sequential decision making as an online learning problem. In this framework, the algorithm designer defines a sequence of online loss functions such that the regret rate in online learning implies the policy convergence rate and the minimal loss witnessed by the policy class determines the policy performance bias. This reduction technique has been successfully applied to solving various policy optimization problems, including imitation learning, structured prediction, and system identification. Interestingly, the policy improvement speed observed in practice is usually much faster than existing theory suggests. In this work, we provide an explanation of this fast policy improvement phenomenon. Let $\epsilon$ denote the policy class bias and assume the online loss functions are convex, smooth, and non-negative. We prove that, after $N$ rounds of OPO with stochastic feedback, the policy converges in $\tilde{O}(1/N + \sqrt{\epsilon/N})$ in both expectation and high probability. In other words, we show that adopting a sufficiently expressive policy class in OPO has two benefits: both the convergence rate increases and the performance bias decreases, as the policy class becomes reasonably rich. This new theoretical insight is further verified in an online imitation learning experiment.
更多查看译文
AI 理解论文
溯源树
样例
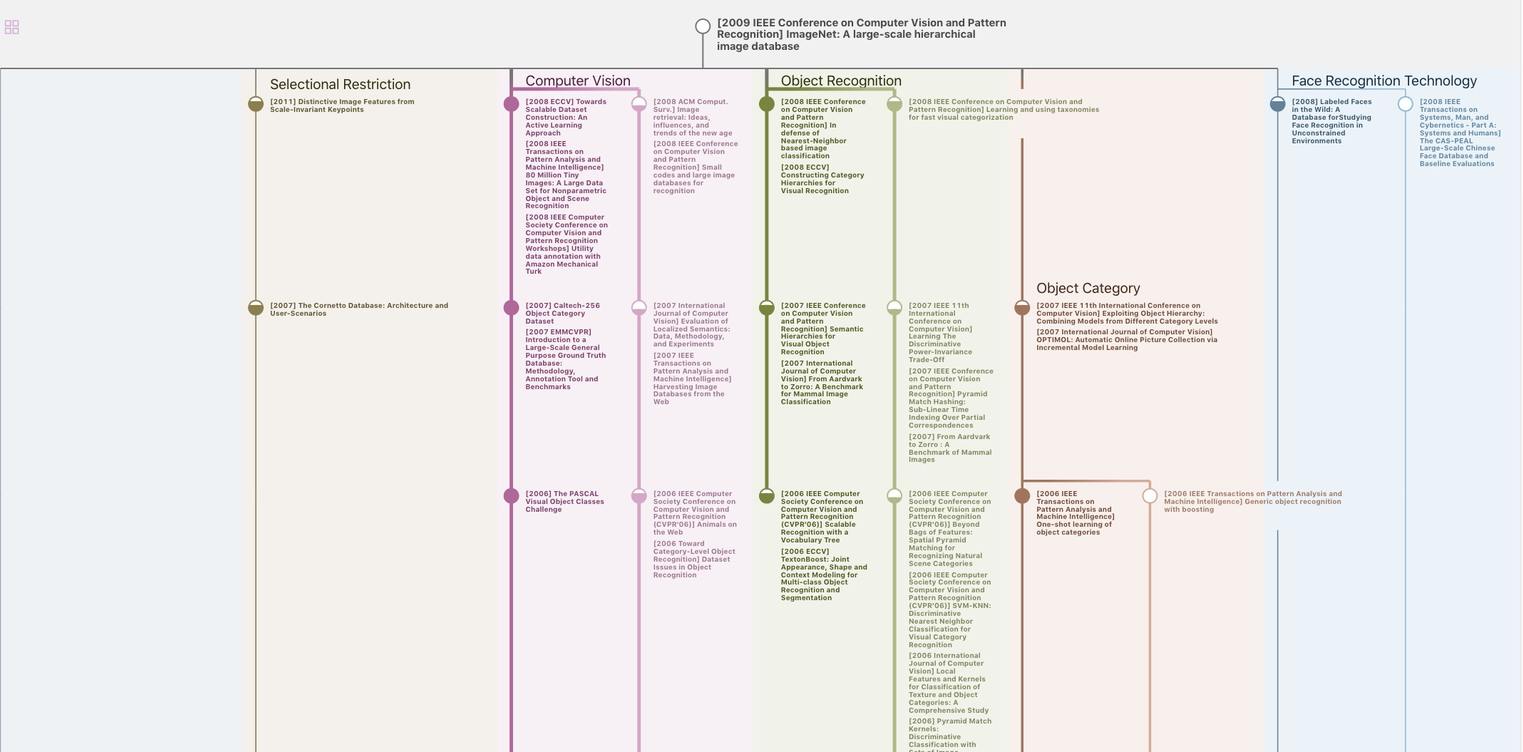
生成溯源树,研究论文发展脉络
Chat Paper
正在生成论文摘要