Progressive Cluster Purification For Unsupervised Feature Learning
2020 25TH INTERNATIONAL CONFERENCE ON PATTERN RECOGNITION (ICPR)(2020)
摘要
In unsupervised feature learning, sample specificity based methods ignore the inter-class information, which deteriorates the discriminative capability of representation models. Clustering based methods are error-prone to explore the complete class boundary information due to the inevitable class inconsistent samples in each cluster. In this work, we propose a novel clustering based method, which, by iteratively excluding class inconsistent samples during progressive cluster formation, alleviates the impact of noise samples in a simple-yet-effective manner. Our approach, referred to as Progressive Cluster Purification (PCP), implements progressive clustering by gradually reducing the number of clusters during training, while the sizes of clusters continuously expand consistently with the growth of model representation capability. With a well-designed cluster purification mechanism, it further purifies clusters by filtering noise samples which facilitate the subsequent feature learning by utilizing the refined clusters as pseudo-labels. Experiments on commonly used benchmarks demonstrate that the proposed PCP improves baseline method with significant margins. Our code will be available at https://github.com/zhangyifei0115/PCP.
更多查看译文
关键词
progressive cluster purification,learning
AI 理解论文
溯源树
样例
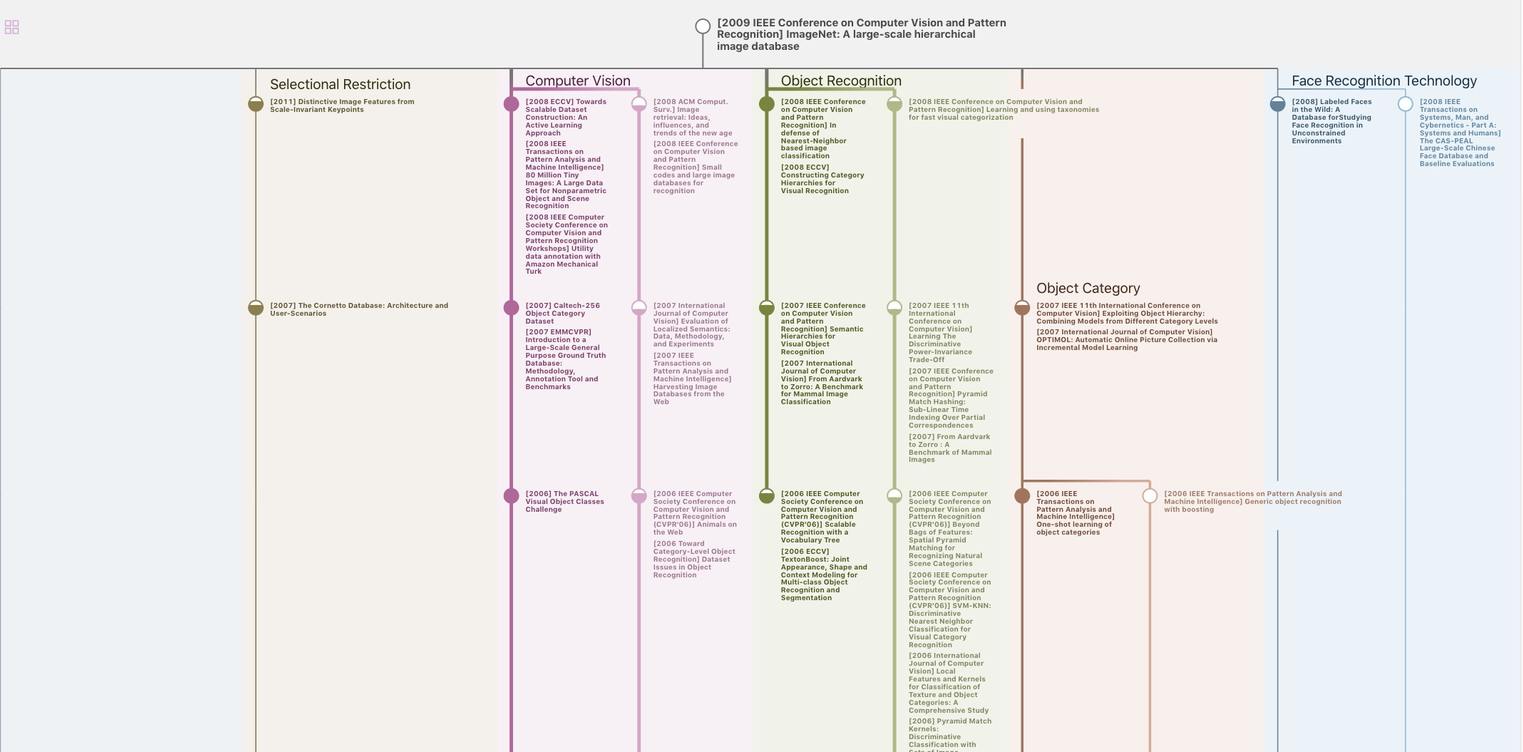
生成溯源树,研究论文发展脉络
Chat Paper
正在生成论文摘要