Fairness in Machine Learning: Against False Positive Rate Equality as a Measure of Fairness
JOURNAL OF MORAL PHILOSOPHY(2022)
摘要
As machine learning informs increasingly consequential decisions, different metrics have been proposed for measuring algorithmic bias or unfairness. Two popular "fairness measures" are calibration and equality of false positive rate. Each measure seems intuitively important, but notably, it is usually impossible to satisfy both measures. For this reason, a large literature in machine learning speaks of a "fairness tradeoff" between these two measures. This framing assumes that both measures are, in fact, capturing something important. To date, philosophers have seldom examined this crucial assumption, and examined to what extent each measure actually tracks a normatively important property. This makes this inevitable statistical conflict between calibration and false positive rate equality - an important topic for ethics. In this paper, I give an ethical framework for thinking about these measures and argue that, contrary to initial appearances, false positive rate equality is in fact morally irrelevant and does not measure fairness.
更多查看译文
关键词
fairness, algorithmic bias, statistical discrimination
AI 理解论文
溯源树
样例
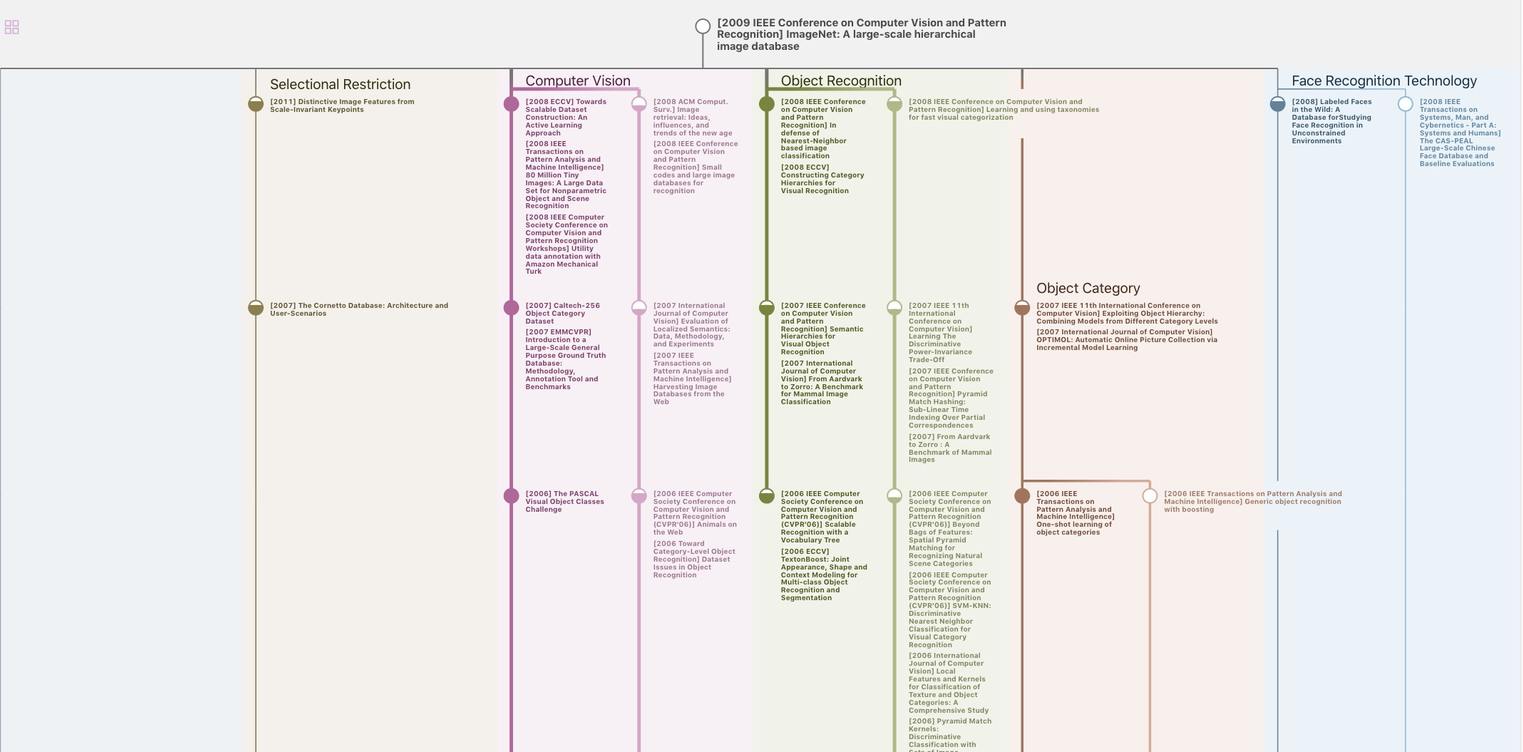
生成溯源树,研究论文发展脉络
Chat Paper
正在生成论文摘要