Generic Coreset for Scalable Learning of Monotonic Kernels: Logistic Regression, Sigmoid and more.
International Conference on Machine Learning(2022)
摘要
Coreset (or core-set) is a small weighted subset $Q$ of an input set $P$ with respect to a given monotonic function $f:\mathbb{R}\to\mathbb{R}$ that provably approximates its fitting loss $\sum_{p\in P}f(p\cdot x)$ to any given $x\in\mathbb{R}^d$. Using $Q$ we can obtain an approximation of $x^*$ that minimizes this loss, by running existing optimization algorithms on $Q$. In this work we provide: (i) A lower bound which proves that there are sets with no coresets smaller than $n=|P|$ for general monotonic loss functions. (ii) A proof that, with an additional common regularization term and under a natural assumption that holds e.g. for logistic regression and the sigmoid activation functions, a small coreset exists for any input $P$. (iii) A generic coreset construction algorithm that computes such a small coreset $Q$ in $O(nd+n\log n)$ time, and (iv) Experimental results with open-source code which demonstrate that our coresets are effective and are much smaller in practice than predicted in theory.
更多查看译文
AI 理解论文
溯源树
样例
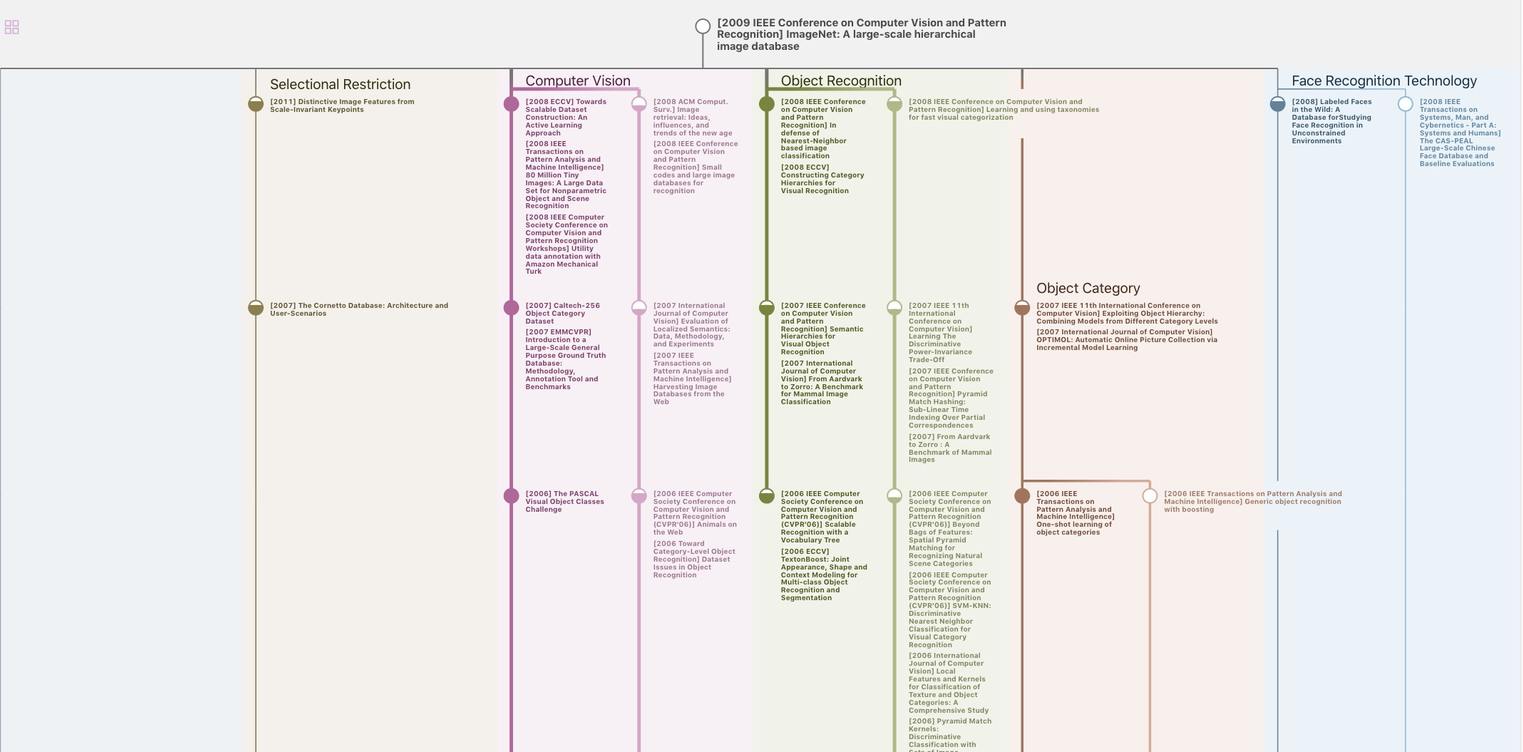
生成溯源树,研究论文发展脉络
Chat Paper
正在生成论文摘要