Bidirectional loss function for Label Enhancement and distribution learning
Knowledge-Based Systems(2021)
摘要
Label distribution learning (LDL) is an interpretable and general learning paradigm that has been applied in many real-world applications. In contrast to the simple logical vector in single-label learning (SLL) and multi-label learning (MLL), LDL assigns labels with a description degree to each instance. In practice, two challenges exist in LDL, namely, how to address the dimensional gap problem during the learning process of LDL and how to exactly recover label distributions from existing logical labels, i.e., Label Enhancement (LE). For most existing LDL and LE algorithms, the fact that the dimension of the input matrix is much higher than that of the output one is always ignored and it typically leads to the dimensional reduction owing to the unidirectional projection. The valuable information hidden in the feature space is lost during the mapping process. To this end, this study considers bidirectional projections function which can be applied in LE and LDL problems simultaneously. More specifically, this novel loss function not only considers the mapping errors generated from the projection of the input space into the output one but also accounts for the reconstruction errors generated from the projection of the output space back to the input one. This loss function aims to potentially reconstruct the input data from the output data. Therefore, it is expected to obtain more accurate results. Experiments on several real-world datasets are carried out to demonstrate the superiority of the proposed method for both LE and LDL. Specifically, BD-LE achieves optimal performance in 85.71% cases and renders sub-optimal in 13.09% cases. BD-LDL ranks 1st in 90.48% cases across six evaluation measurements. Compared with the baseline algorithms, the bidirectional projection methods can outperform the best baselines over 7.38% and 9.98% on average for LE and LDL, respectively.
更多查看译文
关键词
Label distribution learning,Label Enhancement,Bi-directional loss
AI 理解论文
溯源树
样例
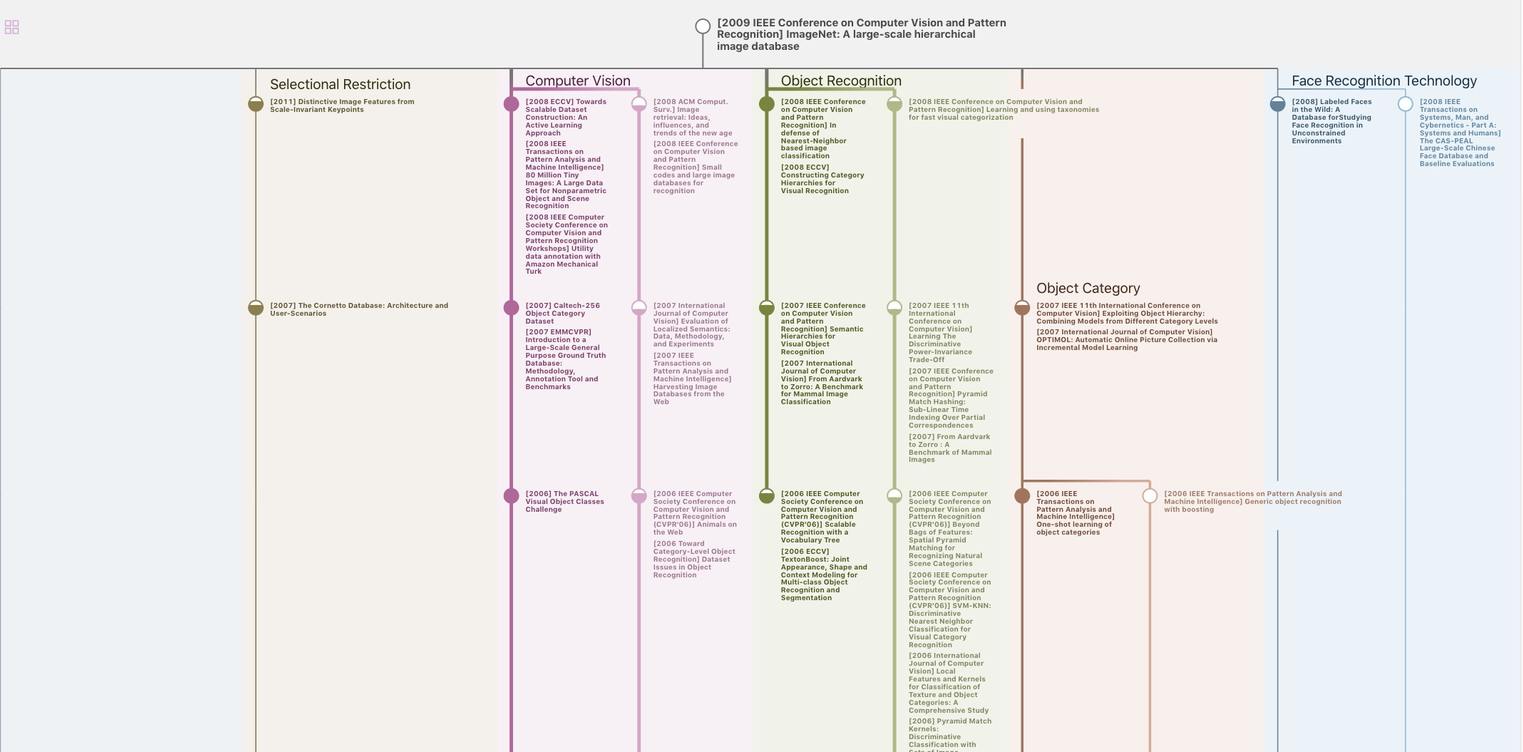
生成溯源树,研究论文发展脉络
Chat Paper
正在生成论文摘要