Off-Policy Evaluation via the Regularized Lagrangian
NIPS 2020(2020)
摘要
The recently proposed distribution correction estimation (DICE) family of estimators has advanced the state of the art in off-policy evaluation from behavior-agnostic data. While these estimators all perform some form of stationary distribution correction, they arise from different derivations and objective functions. In this paper, we unify these estimators as regularized Lagrangians of the same linear program. The unification allows us to expand the space of DICE estimators to new alternatives that demonstrate improved performance. More importantly, by analyzing the expanded space of estimators both mathematically and empirically we find that dual solutions offer greater flexibility in navigating the tradeoff between optimization stability and estimation bias, and generally provide superior estimates in practice.
更多查看译文
关键词
evaluation,off-policy
AI 理解论文
溯源树
样例
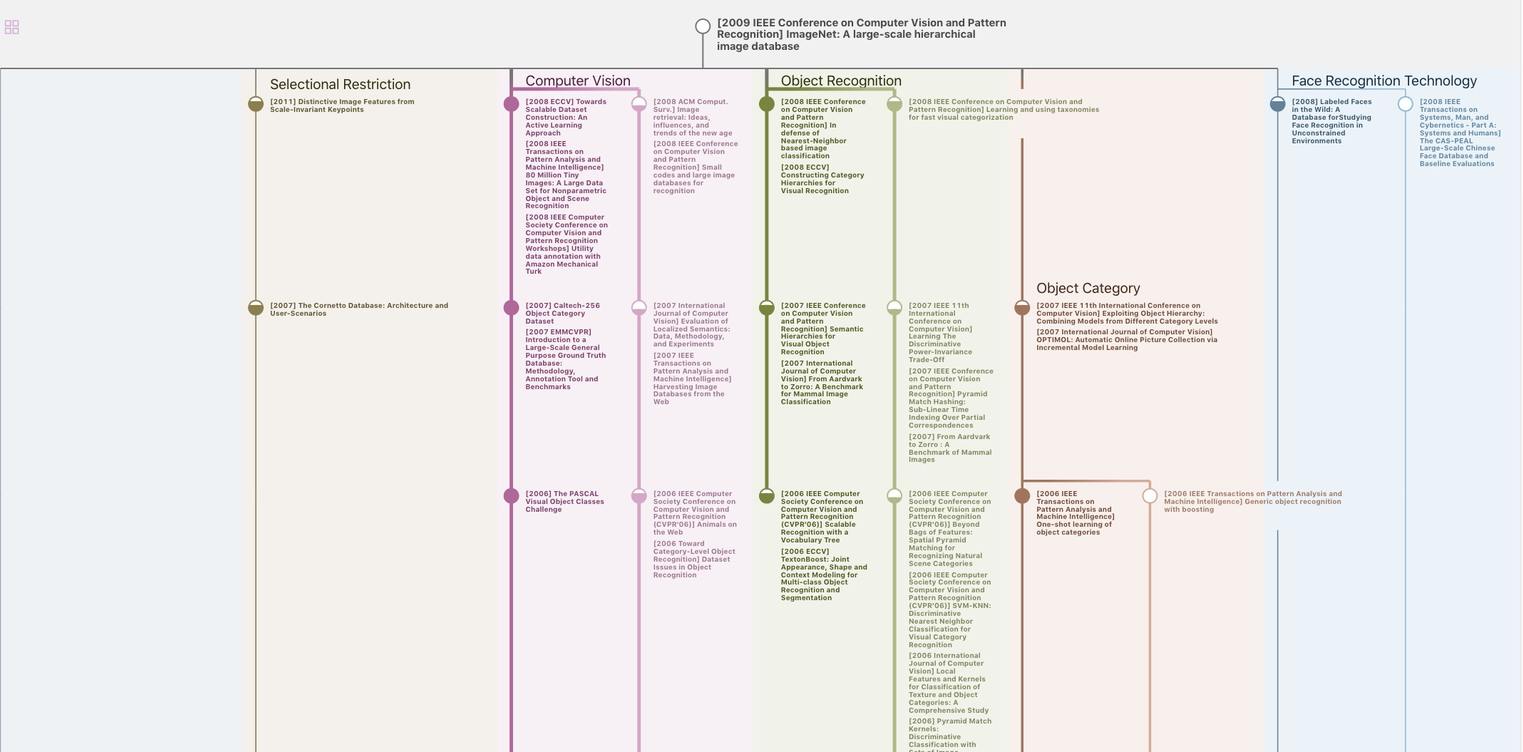
生成溯源树,研究论文发展脉络
Chat Paper
正在生成论文摘要