Classification Of Tumor Signatures From Electrosurgical Vapors Using Mass Spectrometry And Machine Learning: A Feasibility Study
MEDICAL IMAGING 2020: IMAGE-GUIDED PROCEDURES, ROBOTIC INTERVENTIONS, AND MODELING(2021)
摘要
PURPOSE: The iKnife is a new surgical tool designed to aid in tumor resection procedures by providing enriched chemical feedback about the tumor resection cavity from electrosurgical vapors. We build and compare machine learning classifiers that are capable of distinguishing primary cancer from surrounding tissue at different stages of tumor progression. In developing our classification framework, we implement feature reduction and recognition tools that will assist in the translation of xenograft studies to clinical application and compare these tools to standard linear methods that have been previously demonstrated.METHODS: Two cohorts (n=6 each) of 12 week old female immunocompromised (Rag2-/-;I12rg-/-) mice were injected with the same human breast adenocarcinoma (MDA-MB-231) cell line. At 4 and 6 weeks after cell injection, mice in each cohort were respectively euthanized, followed by iKnife burns performed on tumors and tissues prior to sample collection for future studies. A feature reduction technique that uses a neural network is compared to traditional linear analysis. For each method, we fit a classifier to distinguish primary cancer from surrounding tissue.RESULTS: Both classifiers can distinguish primary cancer from metastasis and surrounding tissue. The classifier that uses a neural network achieves an accuracy of 96.8% and the classifier without the neural network achieves an accuracy of 96%.CONCLUSIONS: The performance of these classifiers indicate that this device has the potential to offer real-time, intraoperative classification of tissue. This technology may be used to assist in intraoperative margin detection and inform surgical decisions to offer a better standard of care for cancer patients.
更多查看译文
AI 理解论文
溯源树
样例
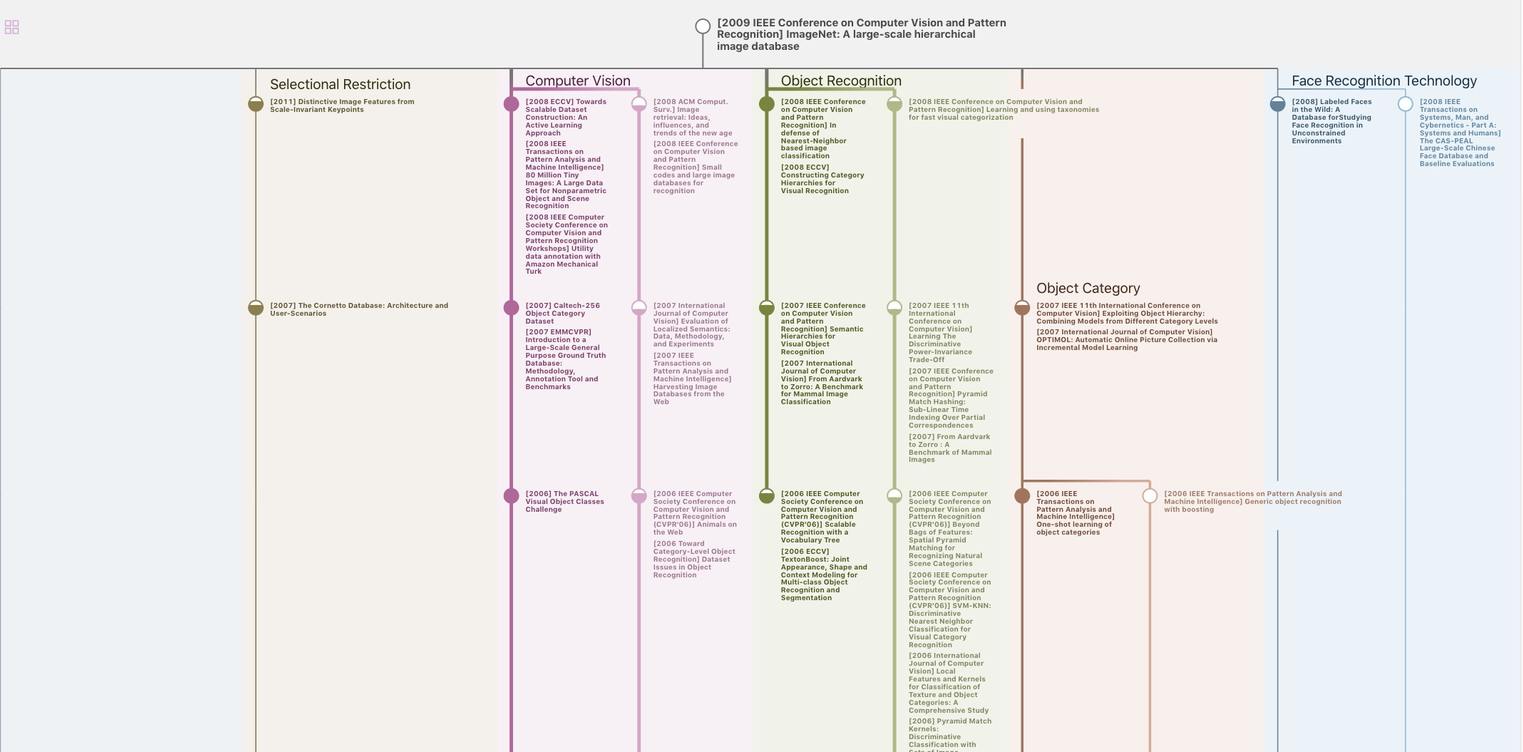
生成溯源树,研究论文发展脉络
Chat Paper
正在生成论文摘要