CPU vs GPU performance of deep learning based face detectors using resized images in forensic applications
9th International Conference on Imaging for Crime Detection and Prevention (ICDP-2019)(2019)
摘要
Accurate and fast face detection is a crucial step in forensic applications such as surveillance, facial fugitives recognition, and child sexual abuse detection. Several deep-learning-based methods addressed the face detection problem with high accuracy but they require a large computation power and processing time. Although GPUs have been used to speed-up computations, nowadays multiple generations of GPU architectures are available (e.g. Turing, Pascal or Keppler) making difficult to choose the most appropriate for face detection. In this work, we evaluate the speed-accuracy trade-off of three deep-learning-based face detectors in various CPUs/GPUs considering images reduced to different proportions as input. We successfully used this image resizing strategy in one previous work to improve the performance of face detection with Child Sexual Exploitation Material (CSEM). The results showed that the best speed-accuracy trade-off is achieved using the Pascal and Turing GPUs with images reduced to 50% of its original size.
更多查看译文
关键词
Face detection,GPU,CPU,Deep learning,CSEM
AI 理解论文
溯源树
样例
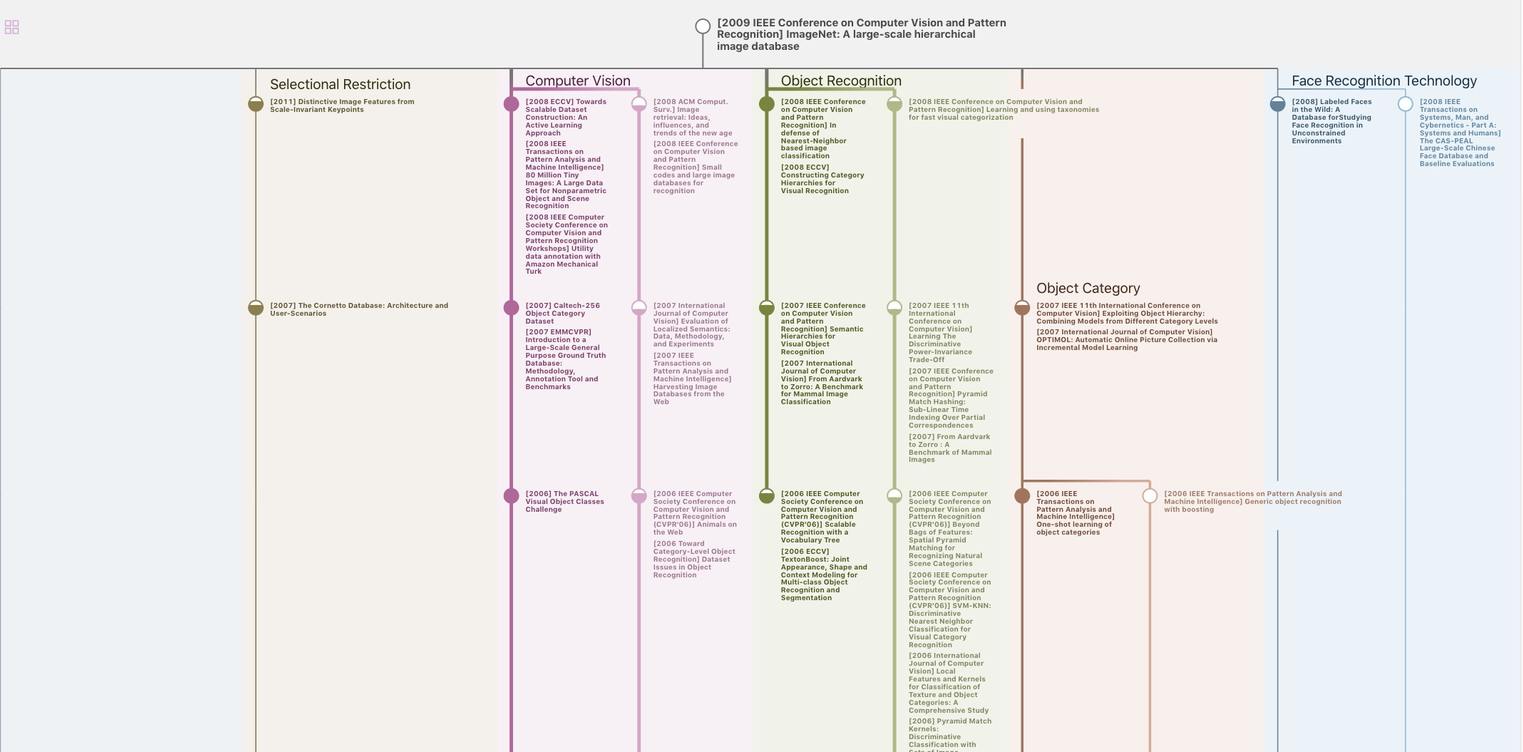
生成溯源树,研究论文发展脉络
Chat Paper
正在生成论文摘要