A Time-Dependent SEIRD Model for Forecasting the COVID-19 Transmission Dynamics
medRxiv(2020)
摘要
The spread of a disease caused by a virus can happen through human to human contact or could be from the environment. A mathematical model could be used to capture the dynamics of the disease spread to estimate the infections, recoveries, and fatalities that may result from the disease. An estimation is crucial to make policy decisions and for the alerts for the medical emergencies that may arise. Many epidemiological models are being used to make such an estimation. One major factor that is important in the forecasts using the models is the dynamic nature of the disease spread. Unless we can come up with a way of estimating the parameters that guide this dynamic spread, the models may not give accurate forecasts. The main principle is to keep the model generic while making minimal assumptions. In this work, we have derived a data-driven model from SEIRD, where we attempt to forecast Infected, Recovered and Deceased rates of COVID-19 up to a week. A method of estimating the parameters of the model is also discussed thoroughly in this work. The model is tested for India at a district level along with the most affected foreign cities like Lombardia from Italy and Moscow from Russia.
更多查看译文
关键词
forecasting,time-dependent
AI 理解论文
溯源树
样例
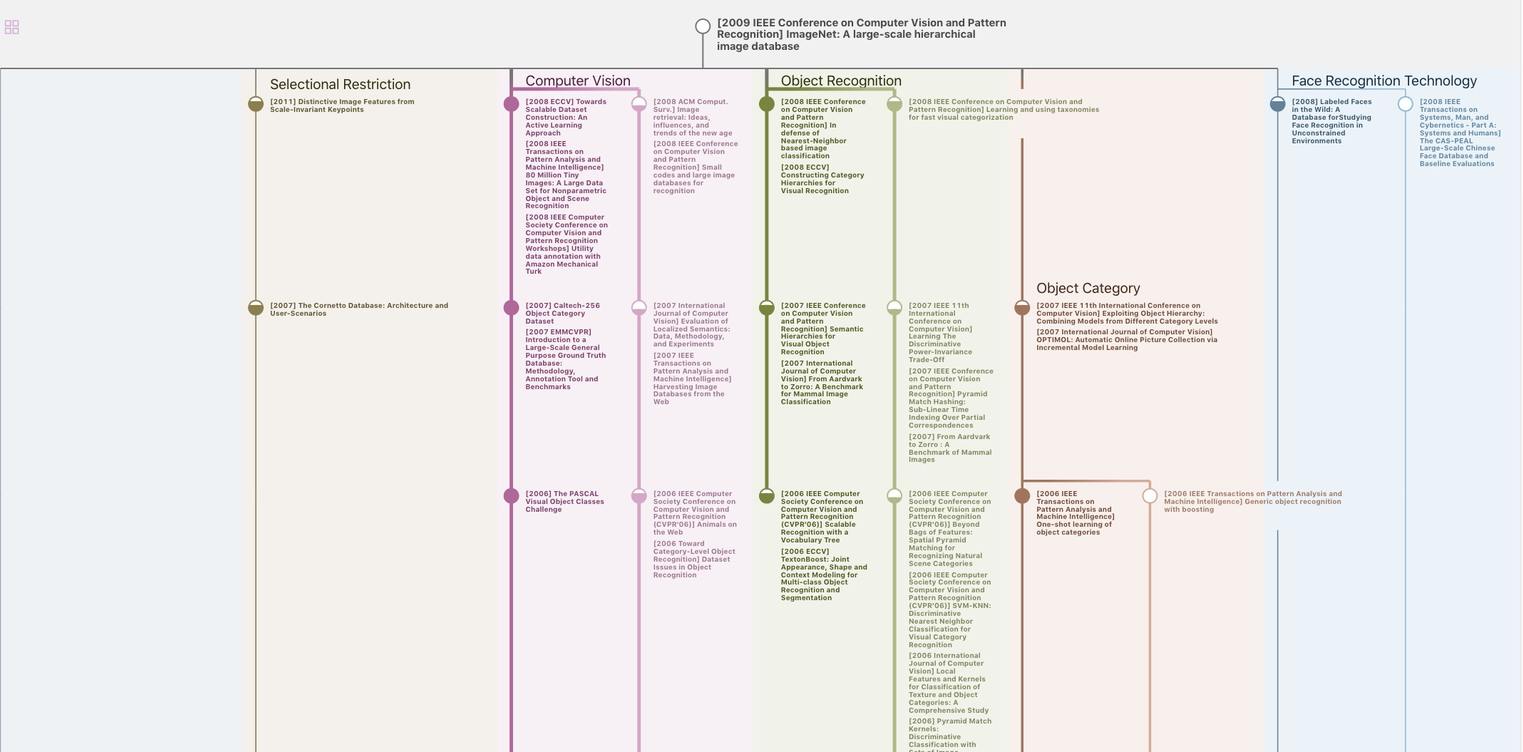
生成溯源树,研究论文发展脉络
Chat Paper
正在生成论文摘要