End-to-end COVID-19 screening with 3D deep learning on chest computed tomography
Research Square(2020)
摘要
Abstract The outbreak of an acute respiratory syndrome (called novel coronavirus pneumonia, NCP) caused by SARS-CoV-2 virus has now progressed to a pandemic, and became the most common threat to public death worldwide[i],[ii]. COVID-19 screening using computed tomography (CT) can perform a quick diagnosis and identify high-risk NCP patients[iii]. Automated screening using CT volumes is a challenging task owing to inter-grader variability and high false-positive and false-negative rates. We propose a three dimensional (3D) deep learning convolutional neural networks (CNN) that use a patient’s CT volume to predict the risk of COVID-19, trained end-to-end from CT volumes directly, using only images and disease labels as inputs. Our model achieves a state-of-the-art performance (95.78% overall accuracy, 99.4% area under the curve) on a dataset of 1,684 COVID-19 patients, nearly twice larger than previous datasets3, and performs similarly on an independent clinical validation set of 121 cases. We tested its performance against six radiologists on clinical confirmed patient’ CT volumes, our model outperformed all six radiologists with absolute reductions of 7% in false positives and 35.9% in false negatives, demonstrating artificial intelligence (AI) capable to optimize the COVID-19 screening process via computer assistance and automation with a level of competence comparable to radiologists. While the vast majority of patients remain unscreened, we show the potential for AI to increase the accuracy and consistency of COVID-19 screening with CT.
更多查看译文
关键词
3d deep learning,chest,screening,tomography,end-to-end
AI 理解论文
溯源树
样例
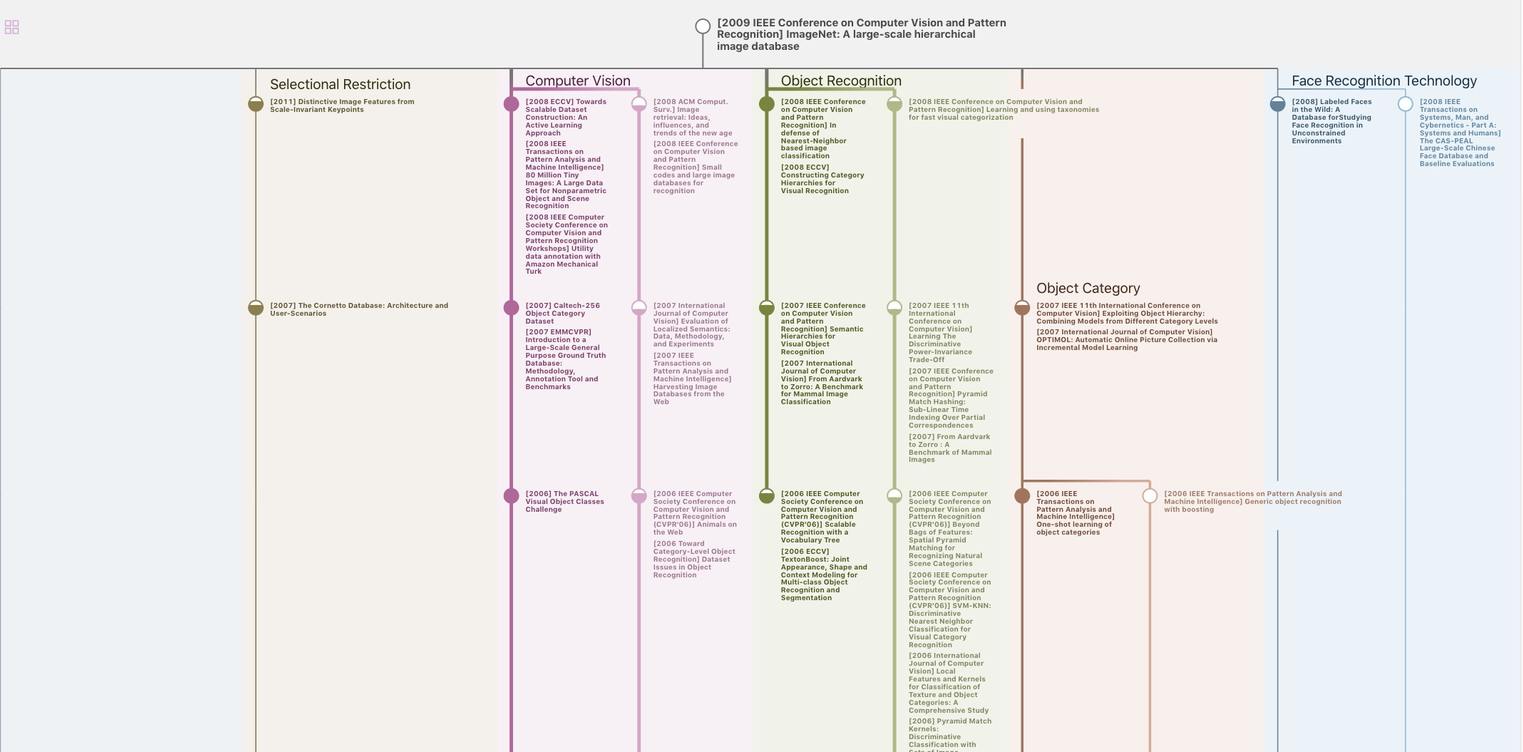
生成溯源树,研究论文发展脉络
Chat Paper
正在生成论文摘要