Hypothesis Testing With High-Dimensional Data
user-5f03edee4c775ed682ef5237(2017)
摘要
In the past two decades, vast high-dimensional biomedical datasets have become mainstay in various biomedical applications from genomics to neuroscience. These high-dimensional data enable researchers to answer scientific questions that are impossible to answer with classical, low-dimensional datasets. However, due to the “curse of dimensionality”, such high-dimensional datasets also pose serious statistical challenges. Motivated by these emerging applications, statisticians have devoted much effort to developing estimation methods for high-dimensional linear models and graphical models. However, there is still little progress on quantifying the uncertainty of the estimates, e.g., obtaining p-values and confidence intervals, which are crucial for drawing scientific conclusions. While encouraging advances have been made in this area over the past couple of years, the majority of existing high-dimensional hypothesis testing methods still suffer from low statistical power or high computational intensity. In this dissertation, we focus on developing hypothesis testing methods for high-dimensional linear and graphical models. In Chapter 2, we investigate a naive and simple two-step hypothesis testing procedure for linear models. We show that, under appropriate conditions, such a simple procedure controls type-I error rate, and is closely connected to more complicated alternatives. We also show in numerical studies that such a simple procedure achieves similar performance as procedures that are computationally more intense. In Chapter 3, we consider hypothesis testing for linear regression that incorporates external information about the …
更多查看译文
AI 理解论文
溯源树
样例
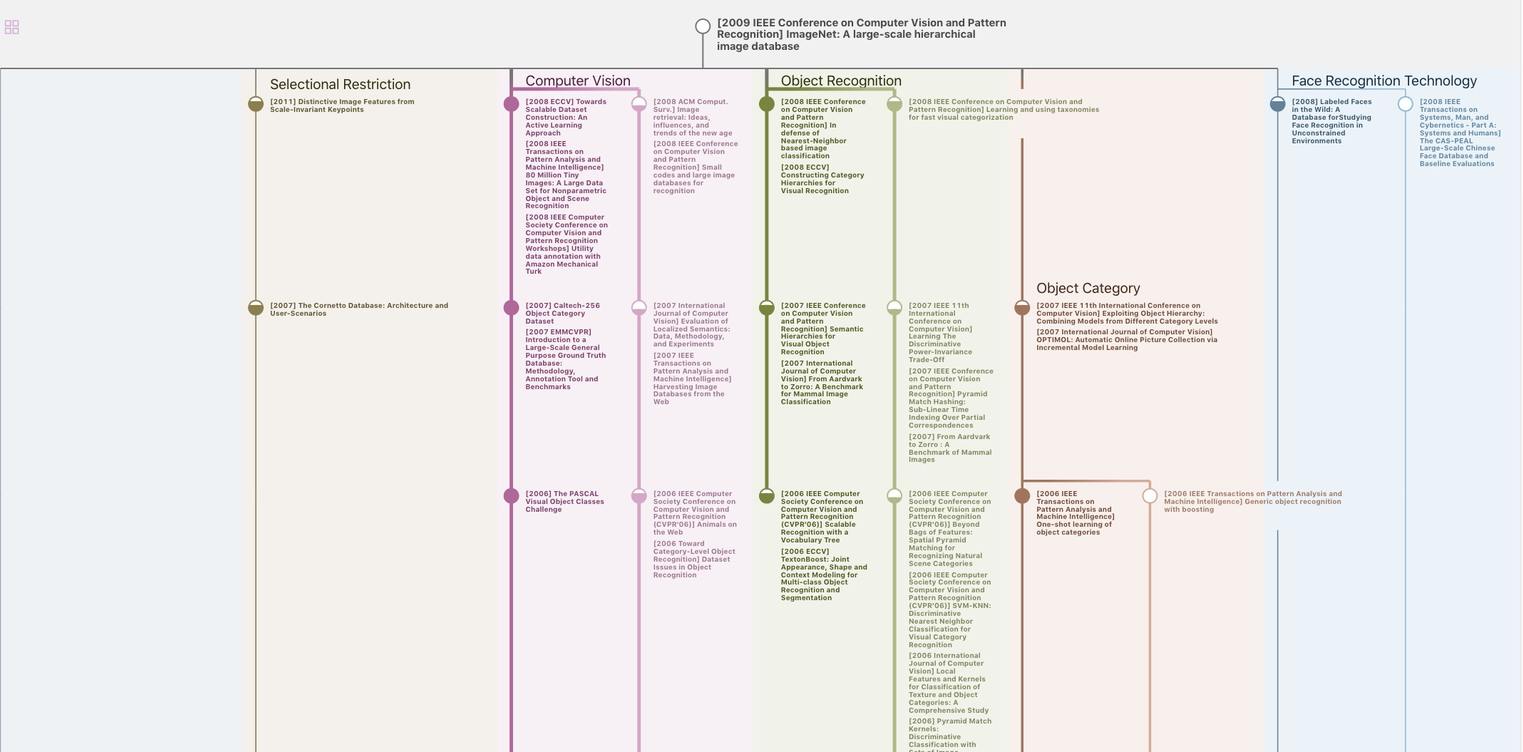
生成溯源树,研究论文发展脉络
Chat Paper
正在生成论文摘要