Bayesian deep learning for integrated intelligence : bridging the gap between perception and inference
user-5efd71244c775ed682ed8a03(2017)
摘要
Deep learning has achieved significant success in many perception tasks including seeing (visual object recognition), reading (text understanding), and hearing (speech recognition). These are undoubtedly fundamental tasks for a functioning comprehensive artificial intelligence (AI) system. However, in order to build a real AI system, simply being able to see, read, and hear is far from enough. It should, above all, possess the ability to think.Take medical diagnosis as an example. Besides seeing visible symptoms (or medical images from CT) and hearing descriptions from patients, a doctor has to look for relations among all the symptoms and infer the etiology. Only after that can the doctor provide medical advice for the patients. In this example, although the abilities of seeing and hearing allow the doctor to acquire information from the patients, it is the thinking part that defines a doctor. Specifically, the ability of thinking here could involve causal inference, logic deduction, and dealing with uncertainty, which is apparently beyond the capability of conventional deep learning methods. Fortunately, another type of models, probabilistic graphical models (PGM), excels at causal inference and dealing with uncertainty. The problem is that PGM is not as good as deep learning models at perception tasks. To address the problem, it is, therefore, a natural choice to tightly integrate deep learning and PGM within a principled probabilistic framework, which we call Bayesian deep learning (BDL) in this thesis.
更多查看译文
AI 理解论文
溯源树
样例
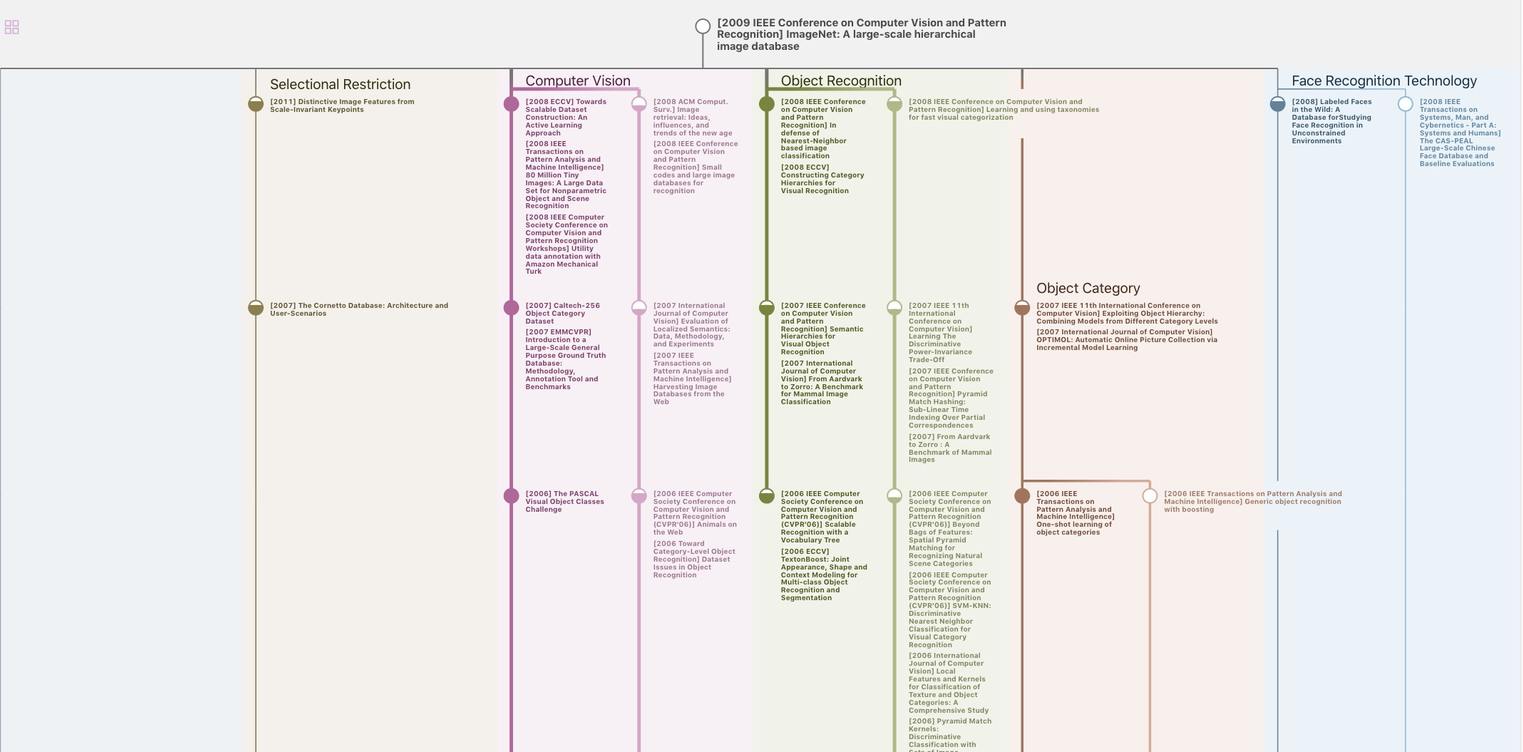
生成溯源树,研究论文发展脉络
Chat Paper
正在生成论文摘要