Turing learning with hybrid discriminators: combining the best of active and passive learning
GECCO '20: Genetic and Evolutionary Computation Conference Cancún Mexico July, 2020(2020)
摘要
We propose a hybrid formulation of Turing Learning and study its application in mobile robotics. Instead of using a single type of discriminator, in the hybrid formulation, both active and passive discriminators are used. Active discriminators come to their judgments while interacting with the system under investigation, which helps improve model accuracy. Passive discriminators come to their judgments while only observing the system, allowing the reuse of data samples, which for real robots would be costly to obtain. To validate these ideas, we present a case study where a simulated embodied robot is required to calibrate its distance sensor through a process of self-modeling, and without metric information of where it resides within the environment. The results show that the hybrid formulation achieves a good level of accuracy with significantly fewer data samples from the robot. The findings suggest that the self-modeling process could be realized on a mobile physical robot with a limited time and energy budget.
更多查看译文
AI 理解论文
溯源树
样例
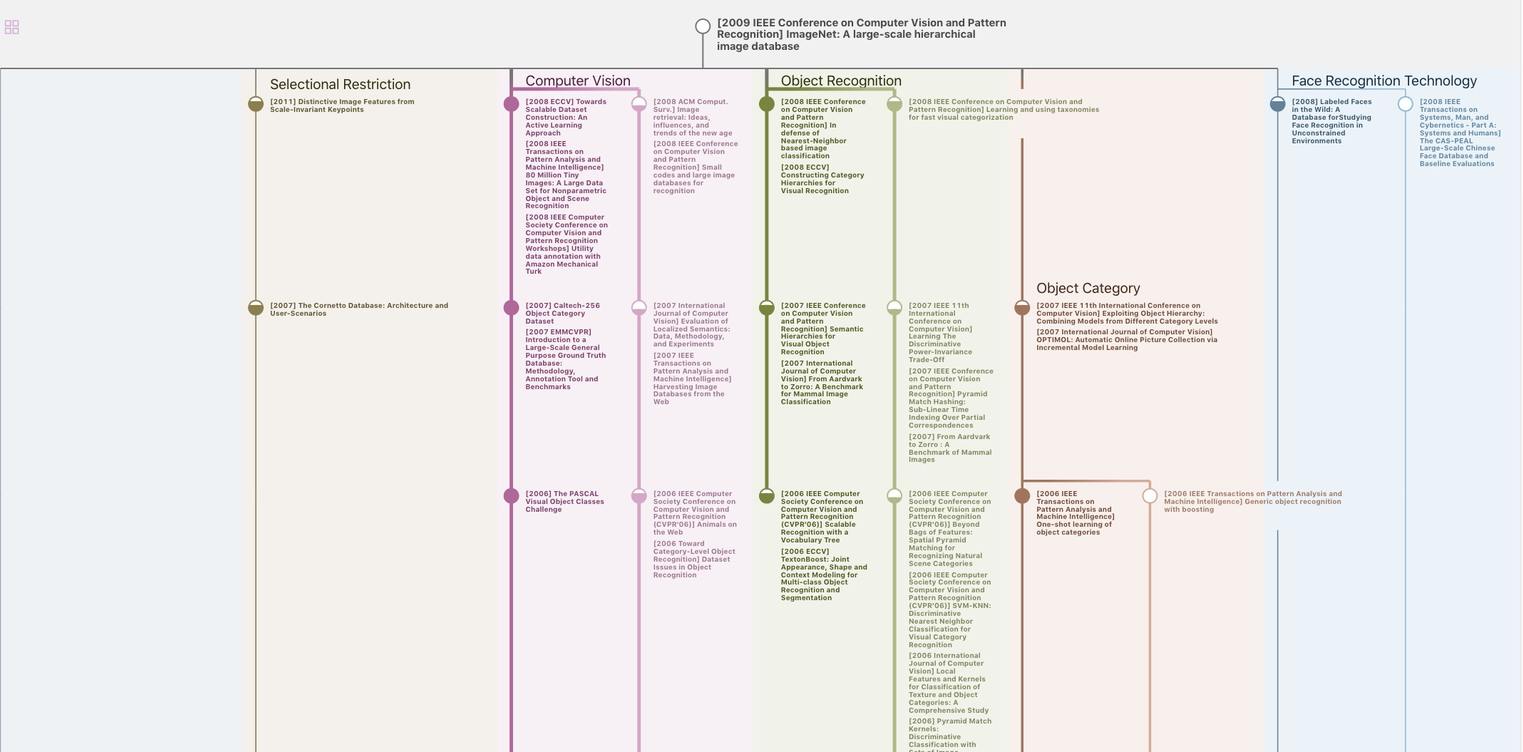
生成溯源树,研究论文发展脉络
Chat Paper
正在生成论文摘要