Exploring the BipedalWalker Benchmark with MAP-Elites and Curiosity-Driven A3C
Proceedings of the 2020 Genetic and Evolutionary Computation Conference Companion(2020)
摘要
The MAP-Elites algorithm can efficiently explore reinforcement learning problems and optimize collections of solutions over user-defined ranges of possible behaviors. However, MAP-Elites can be difficult to scale to highly dimensional problems, such as the optimization of large deep neural networks. Traditional deep reinforcement can train agents with a complex network model by relying on human-designed extrinsic rewards. In complex problems, this translates into reward landscapes that are extremely sparse and hard to explore. This has led to the development of curiosity-driven reinforcement learning algorithms that use intrinsic rewards to continuously optimize for novel policies. While this approach encourages exploration, it remains to be seen if it can be used similarly to MAP-Elites to search for a collection of highly diverse solutions.
更多查看译文
AI 理解论文
溯源树
样例
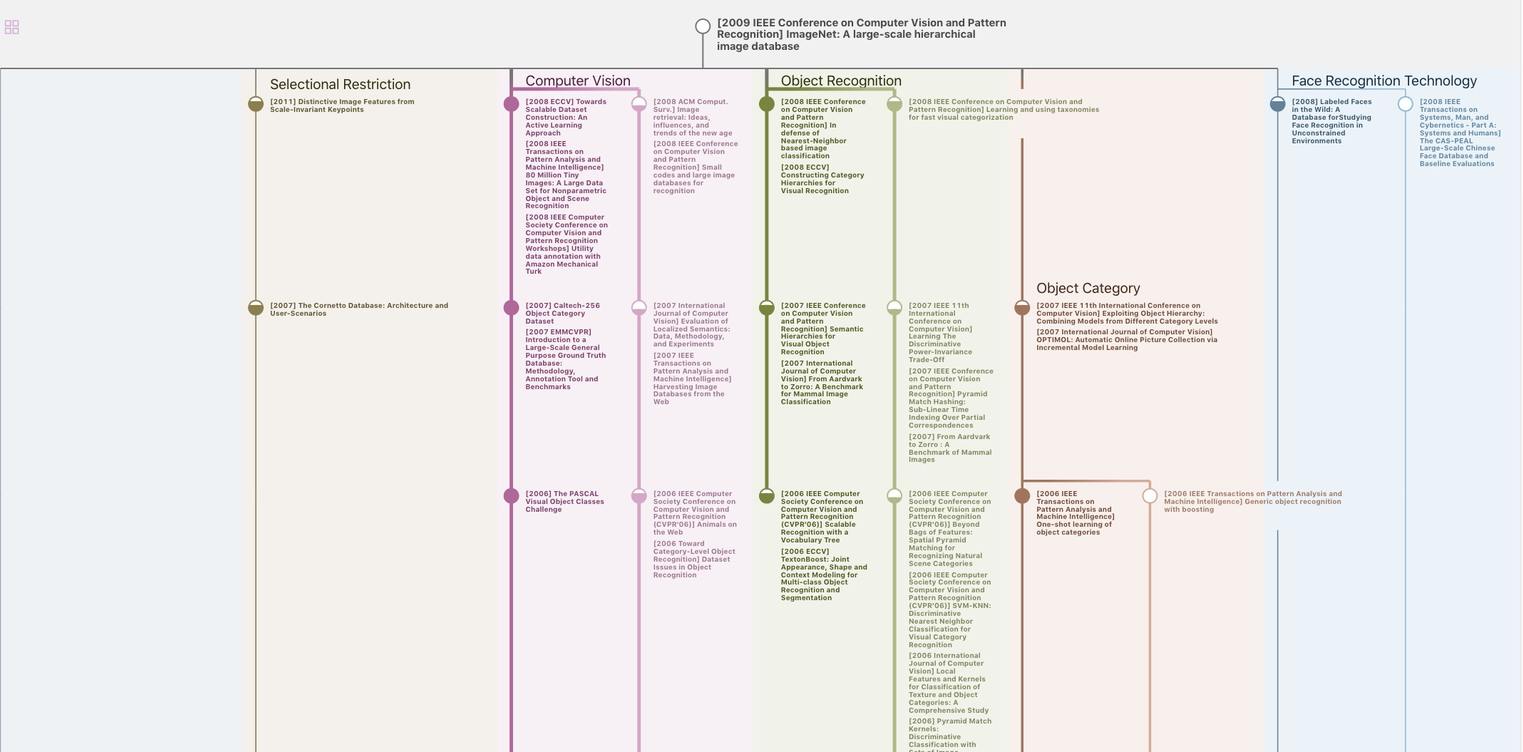
生成溯源树,研究论文发展脉络
Chat Paper
正在生成论文摘要