Boosting binary masks for multi-domain learning through affine transformations
Machine Vision and Applications(2020)
摘要
In this work, we present a new, algorithm for multi-domain learning. Given a pretrained architecture and a set of visual domains received sequentially, the goal of multi-domain learning is to produce a single model performing a task in all the domains together. Recent works showed how we can address this problem by masking the internal weights of a given original convnet through learned binary variables. In this work, we provide a general formulation of binary mask-based models for multi-domain learning by affine transformations of the original network parameters. Our formulation obtains significantly higher levels of adaptation to new domains, achieving performances comparable to domain-specific models while requiring slightly more than 1 bit per network parameter per additional domain. Experiments on two popular benchmarks showcase the power of our approach, achieving performances close to state-of-the-art methods on the Visual Decathlon Challenge.
更多查看译文
关键词
Multi-domain learning,Multi-task learning,Quantized neural networks
AI 理解论文
溯源树
样例
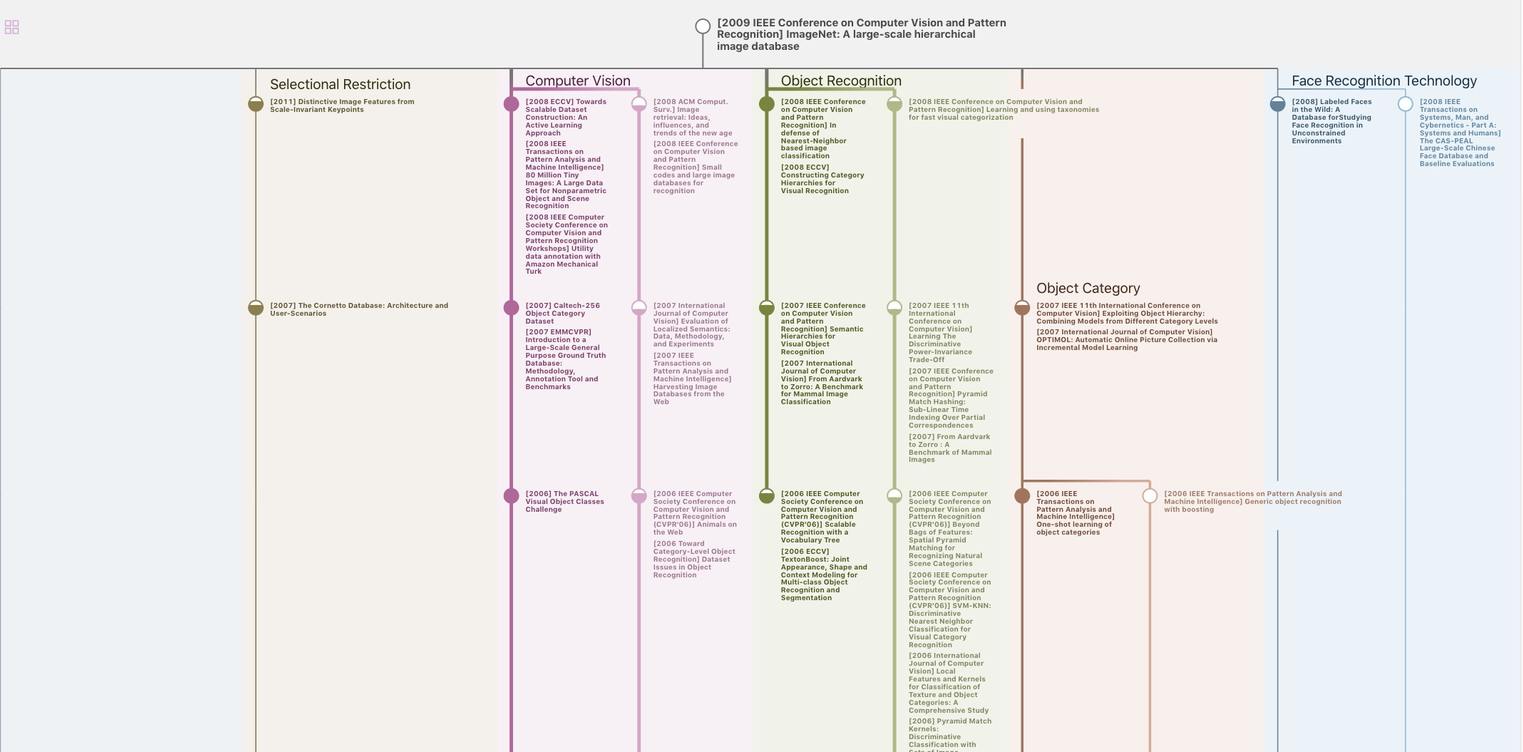
生成溯源树,研究论文发展脉络
Chat Paper
正在生成论文摘要