Neighbor Aggregative Network Embedding
user-5eddf84c4c775e09d87c9229(2018)
摘要
Network embedding is to transform nodes in a network into lowdimensional vector representations. Network embedding models need to answer two questions:(1) How to effectively utilize the information from a node’s neighborhood. We observe that current methods either do not use such information adequately, or use the information in problematic ways. We propose a neighborhood aggregative approach based on the theory of Graph Convolution. After demonstrating the equivalence between Graph Convolution and neighbor aggregation, we study different ways to perform such aggregation, and propose a specially designed aggregator called Weighted Row Laplacian, to suit the need of network embedding.(2) How to deal with the fact that real-world networks often exhibit great sparsity and highly skewed node degree distributions. In our model, higher-order neighborhood information is explored to enrich the amount of structural information contained in our embeddings. Instead of imposing a pre-specified definition of neighborhood, we propose a dynamic sampling algorithm. It gradually explores higher-order neighbors during training, and adaptively changes the exploration rate for nodes of different degrees. Experiments demonstrate the effectiveness of our model, NANE 1, through three network mining tasks, ie link prediction, node classification, and visualization.
更多查看译文
AI 理解论文
溯源树
样例
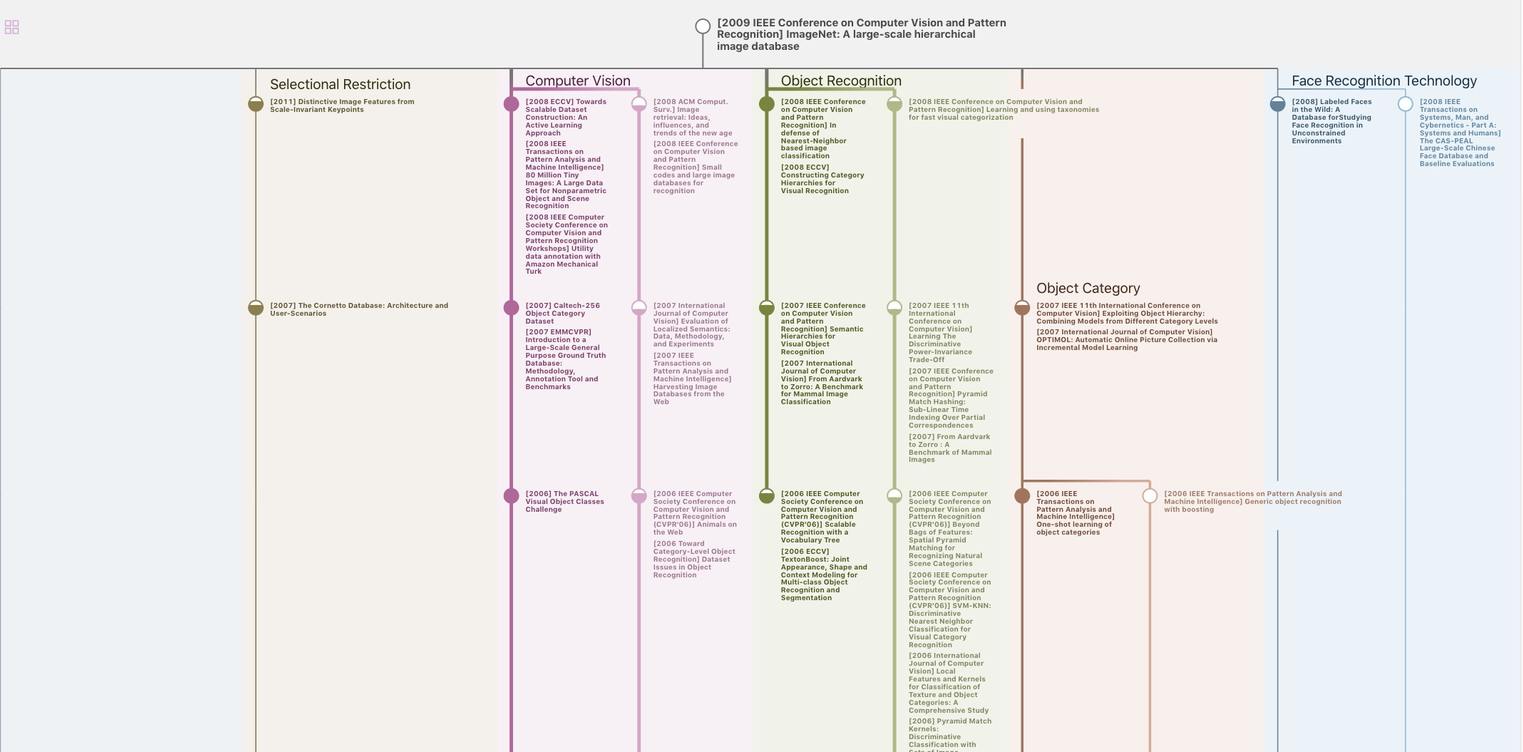
生成溯源树,研究论文发展脉络
Chat Paper
正在生成论文摘要