Training an Inverse Operator on Shingling Seismic Data based on cGANs
SEG 2018 Workshop: SEG Maximizing Asset Value Through Artificial Intelligence and Machine Learning, Beijing, China, 17-19 September 2018(2018)
摘要
We focus on a specific inverse problem, which is to work out the near-surface velocity model from shingling data that may be caused by reversed velocity sequences. The traditional inversion method first arrival traveltime tomography cannot handle such type of seismic data. A conditional generative adversarial network has the similar goal with an inverse problem. That is to generate model from given data, trying not to be distinguished from the true model given in training process. The network learns the relationship between data and model, especially the direction from data to model, and it can be seen as an inverse operator. We rebuild our network based on a well used general image-to-image cGANs framework, and create our datasets of shingling data through wave-equation based finite difference simulation method. After training 2D synthetic pairs of shingling seismic data and velocity model, we acquire a 100 …
更多查看译文
关键词
shingling seismic data,inverse operator
AI 理解论文
溯源树
样例
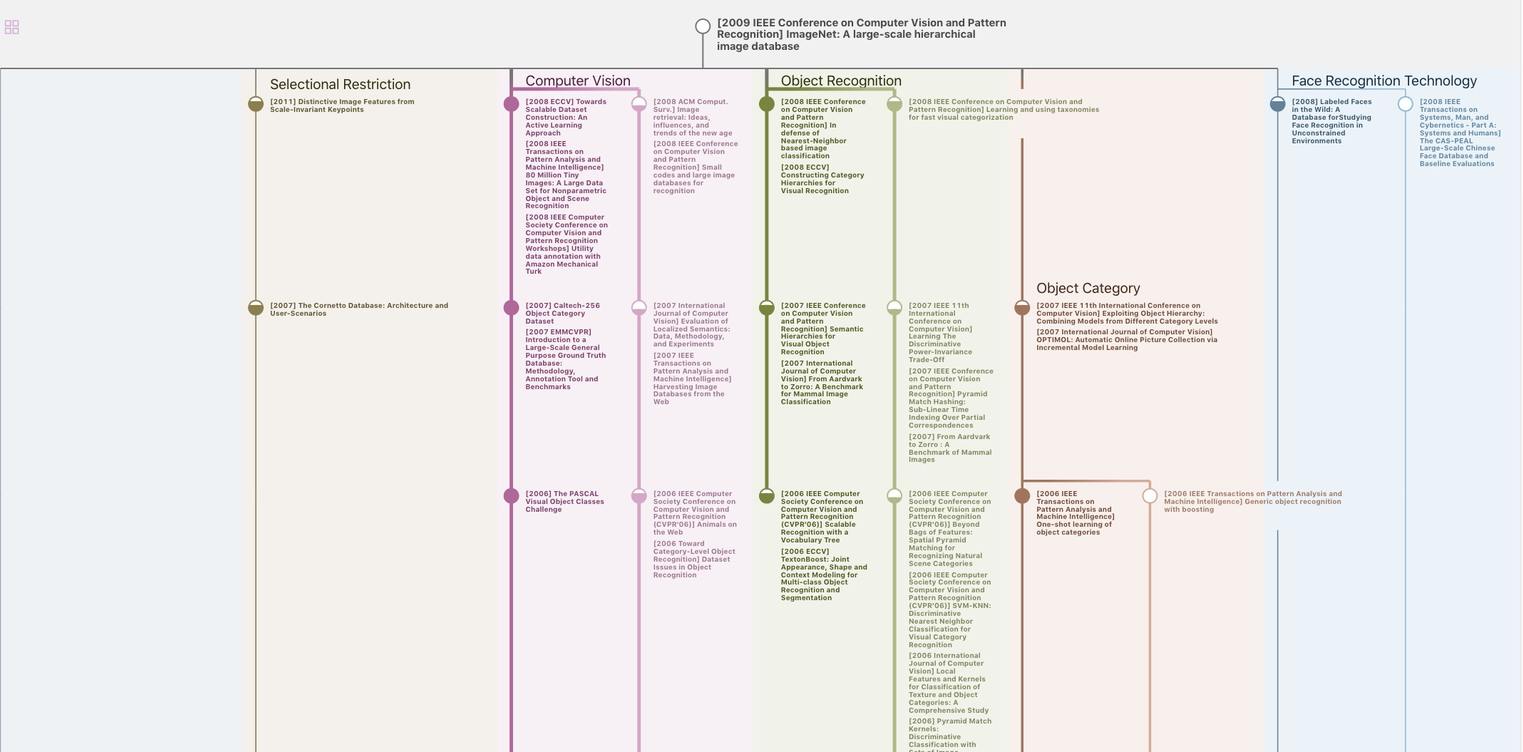
生成溯源树,研究论文发展脉络
Chat Paper
正在生成论文摘要