Model-based Learning in Multivariate Time Series
semanticscholar(2018)
摘要
Multivariate time series is a very active topic in the research community and many machine learning tasks are being used in order to extract information from this type of data. However, in real-world problems, the data has missing values and may lack prior information about the groups, which may difficult the application of machine learning techniques to extract information. Hence, this thesis will focus on the tasks of imputation and clustering. Many imputation methods of time series are based on regression methods; however, these type of methods cannot capture the information between the variables of multivariate categorical time series. As such this thesis proposes a new imputation method that uses the Dynamic Bayesian Networks. The task of clustering tries to group similar time series. State of the art methods can be divided in two types: the ones that use a custom distance and then use a traditional clustering method like k-means; and the ones that use models to cluster similar time series. However, most of these methods do not take into consideration the relationship between variables. As such, this thesis proposes a new clustering method based on Dynamic Bayesian Multinets to cluster similar time series. The devised methods are assessed in synthetic data, showing that it outperforms the state of the art methods, in both tasks. Further experimental tests are done using real datasets, where it is shown that the proposed methods are more consistent than the state of the art methods in their tasks.
更多查看译文
AI 理解论文
溯源树
样例
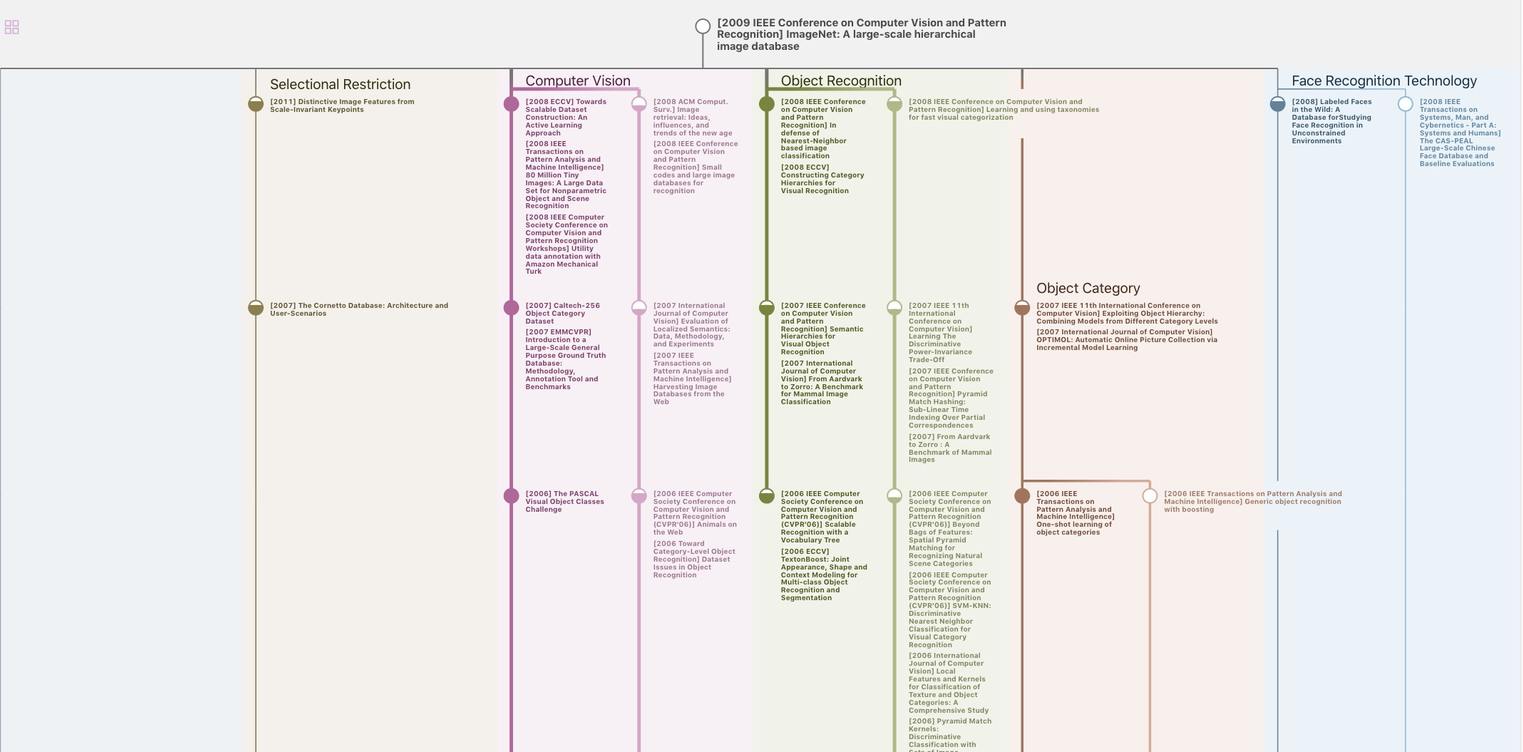
生成溯源树,研究论文发展脉络
Chat Paper
正在生成论文摘要