Unifying Sum-Product Networks and Submodular Fields
Proceedings of the Workshop on Principled Approaches to Deep Learning at ICML(2017)
摘要
Sum-product networks (SPNs) are a relatively new class of deep probabilistic models that are expressive but also tractable, due in part to their ability to exploit local structure in the form of context-specific independence and determinism. However, there remain many types of problems that are highly structured, but for which SPNs are intractable. In particular, the MAP solution of a Markov random field (MRF) with submodular energy can be computed in low-order polynomial time, but an SPN representing the same distribution would be intractable. In this work, we present submodular sum-product networks (SSPNs), a generalization of SPNs in which sumnode weights can be defined by a submodular energy function. SSPNs combine the expressivity and depth of SPNs with the ability to efficiently compute the MAP state of a combinatorial number of labelings afforded by submodularity, greatly increasing the expressivity of the SPN model class. We develop a movemaking algorithm for computing the (approximate) MAP state of an SSPN and analytically show that it is both efficient and convergent. Empirically, we show exponential improvements in inference time compared to traditional inference algorithms such as α-expansion and belief propagation, while returning comparable minima. Finally, we present promising empirical results when using SSPNs for scene understanding.
更多查看译文
AI 理解论文
溯源树
样例
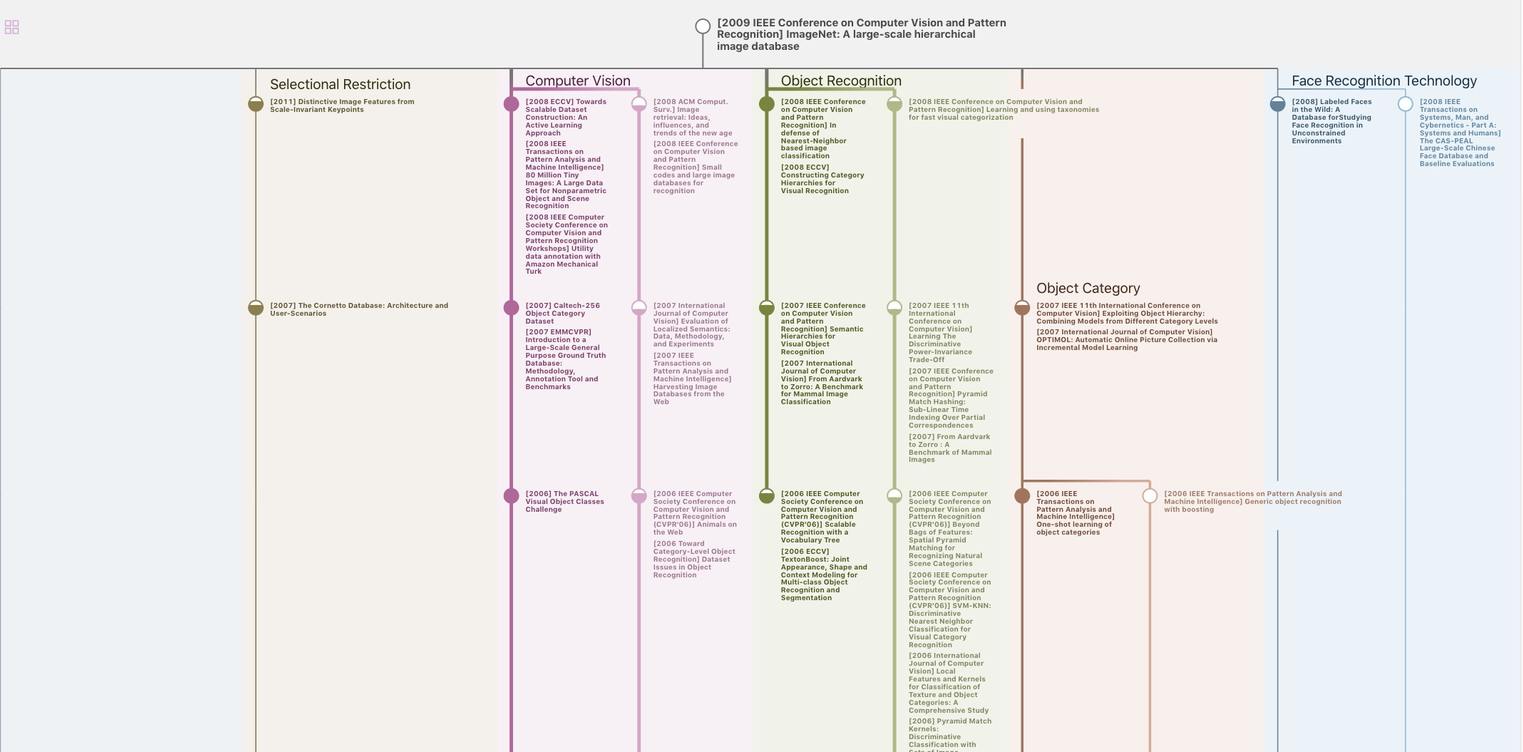
生成溯源树,研究论文发展脉络
Chat Paper
正在生成论文摘要