D S ] 1 5 M ar 2 01 9 Utility Preserving Secure Private Data Release
semanticscholar(2019)
摘要
Differential privacy mechanisms that also make reconstruction of the data impossible come at a cost a decrease in utility. In this paper, we tackle this problem by designing a private data release mechanism that makes reconstruction of the original data impossible and also preserves utility for a wide range of machine learning algorithms. We do so by combining the JohnsonLindenstrauss (JL) transform with noise generated from a Laplace distribution. While the JL transform can itself provide privacy guarantees (Blocki et al., 2012) and make reconstruction impossible, we do not rely on its differential privacy properties and only utilize its ability to make reconstruction impossible. We present novel proofs to show that our mechanism is differentially private under single element changes as well as single row changes to any database. In order to show utility, we prove that our mechanism maintains pairwise distances between points in expectation and also show that its variance is proportional to the dimensionality of the subspace we project the data into. Finally, we experimentally show the utility of our mechanism by deploying it on the task of clustering.
更多查看译文
AI 理解论文
溯源树
样例
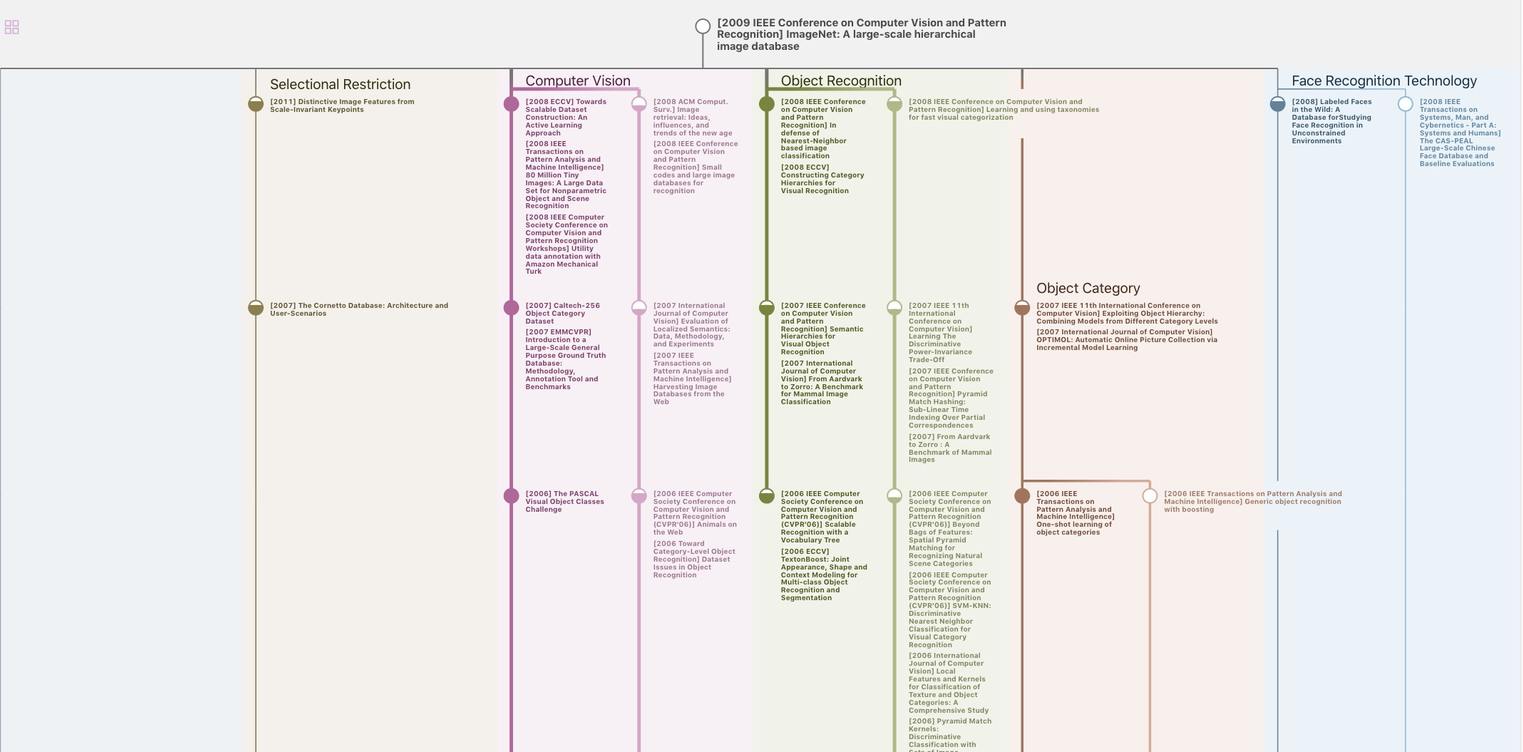
生成溯源树,研究论文发展脉络
Chat Paper
正在生成论文摘要