Title Low-Dimensional Models for Compressed Sensing andPrediction of Large-Scale Traffic Data
semanticscholar(2019)
Abstract
Advanced sensing and surveillance technologies often collect traffic information with high temporal and spatial resolutions. The volume of the collected data severely limits the scalability of online traffic operations. To overcome this issue, we propose a low-dimensional network representation where only a subset of road segments is explicitly monitored. Traffic information for the subset of roads is then used to estimate and predict conditions of the entire network. Numerical results show that such approach provides 10 times faster prediction at a loss of performance of 3% and 1% for 5 and 30 minutes prediction horizons, respectively.
MoreTranslated text
AI Read Science
Must-Reading Tree
Example
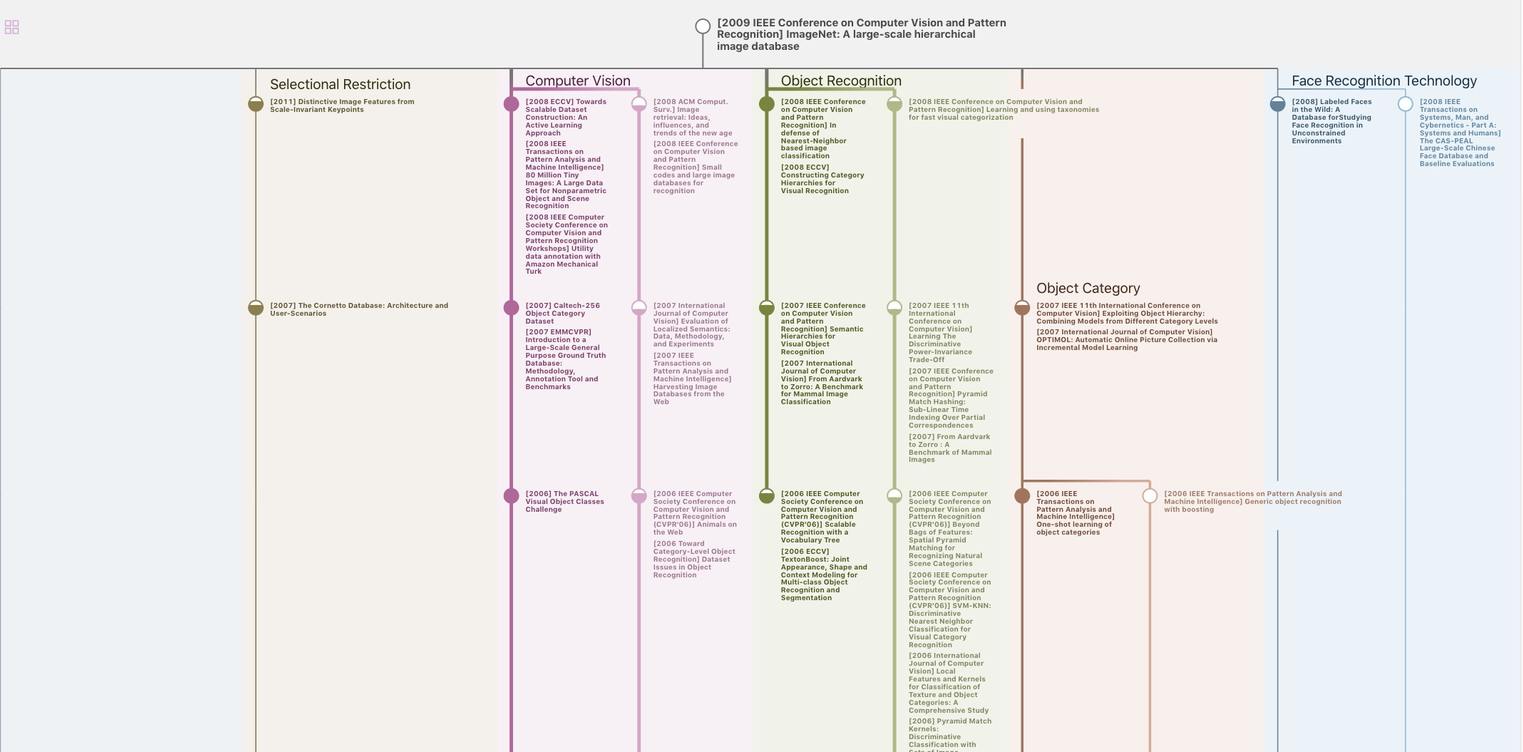
Generate MRT to find the research sequence of this paper
Chat Paper
Summary is being generated by the instructions you defined