Towards High-Level Intrinsic Exploration in Reinforcement Learning.
IJCAI 2020(2020)
摘要
Deep reinforcement learning (DRL) methods traditionally struggle with tasks where environment rewards are sparse or delayed, which entails that exploration remains one of the key challenges of DRL. Instead of solely relying on extrinsic rewards, many state-of-the-art methods use intrinsic curiosity as exploration signal. While they hold promise of better local exploration, discovering global exploration strategies is beyond the reach of current methods. We propose a novel end-to-end intrinsic reward formulation that introduces high-level exploration in reinforcement learning. Our curiosity signal is driven by a fast reward that deals with local exploration and a slow reward that incentivizes long-time horizon exploration strategies. We formulate curiosity as the error in an agent’s ability to reconstruct the observations given their contexts. Experimental results show that this high-level exploration enables our agents to outperform prior work in several Atari games.
更多查看译文
关键词
Machine Learning: Deep Reinforcement Learning,Machine Learning: Reinforcement Learning,Agent-based and Multi-agent Systems: Other
AI 理解论文
溯源树
样例
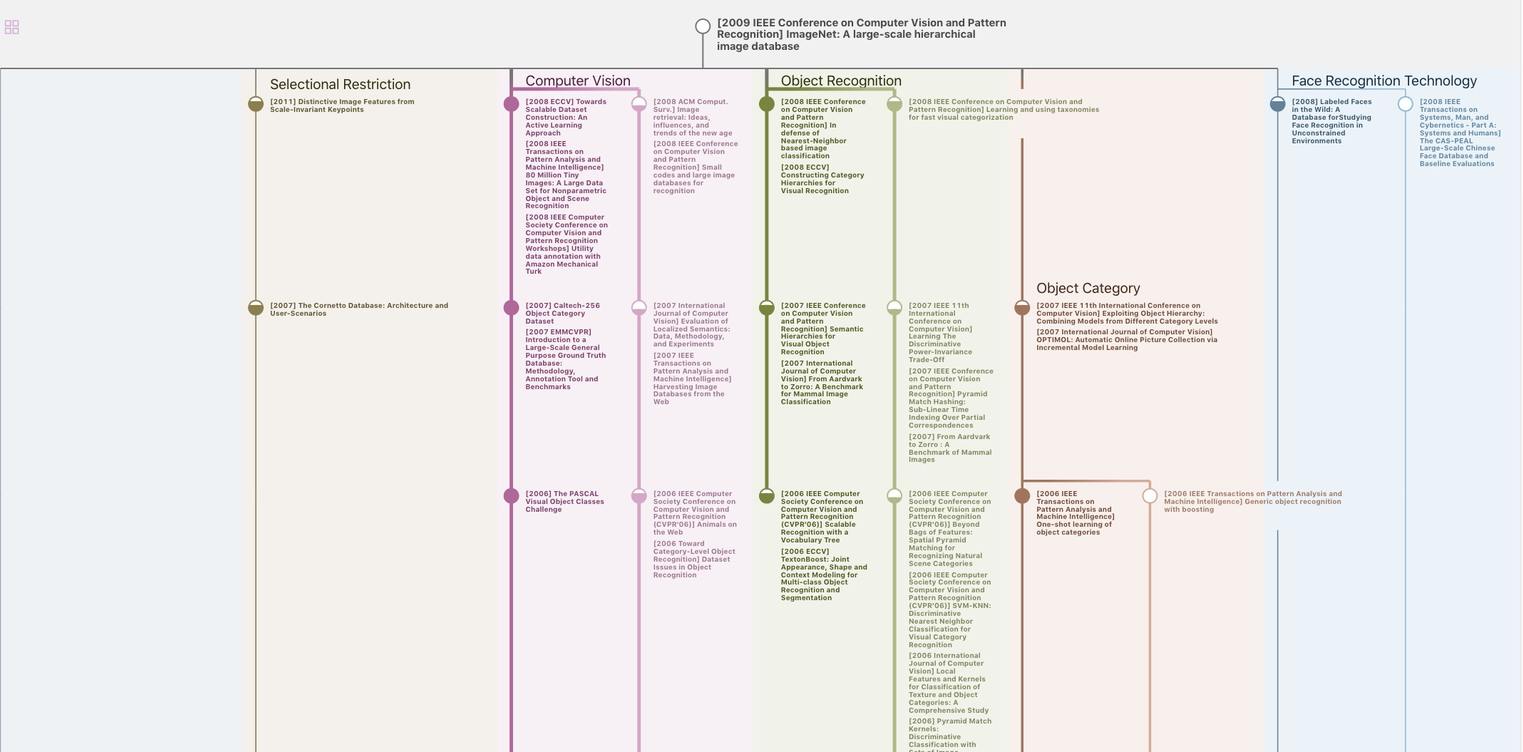
生成溯源树,研究论文发展脉络
Chat Paper
正在生成论文摘要