Bridging Causality and Learning - How Do They Benefit from Each Other?
IJCAI(2020)
摘要
Modern machine learning techniques can discover complicated statistical dependencies between random variables, usually in the form a statistical model, and make use of these dependencies to perform predictions on future observations. However, many real problems involve causal inference, which aims to infer how the data generating system should behave under changing conditions. To perform causal inference, we need not only statistical dependencies but also causal structures to determine the system's behavior under external interventions. In this paper, I will be focusing on two essential problems that bridge causality and learning and investigate how they can benefit from each other. On the one hand, since conducting randomized controlled experiments for causal structure discovery is often expensive or infeasible, it would be valuable to investigate how we can explore modern machine learning algorithms to search for causal structures from observational data. On the other hand, since causal structure provides information about the distribution change properties, it can be used as a fundamental tool to tackle a major challenge for machine learning: the capability of generalization to new distributions and prediction in nonstationary environment.
更多查看译文
AI 理解论文
溯源树
样例
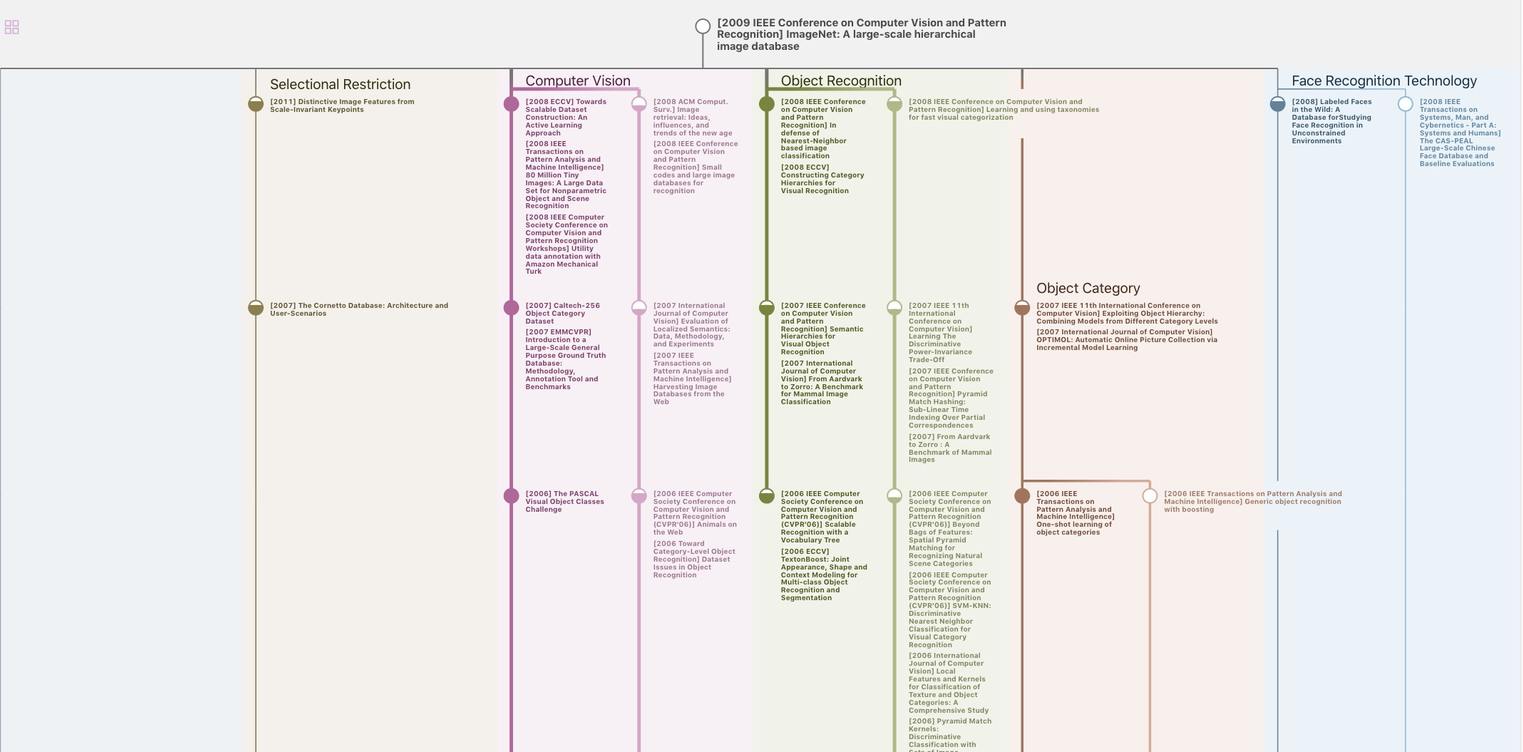
生成溯源树,研究论文发展脉络
Chat Paper
正在生成论文摘要