Controllable speech synthesis
semanticscholar(2020)
摘要
We present a novel generative model that combines state-of-the-art neural textto-speech (TTS) with semi-supervised probabilistic latent variable models. By providing partial supervision to some of the latent variables, we are able to force them to take on consistent and interpretable purposes, which previously hasn’t been possible with purely unsupervised TTS models. We demonstrate that our model is able to reliably discover and control important but rarely labelled attributes of speech, such as affect and speaking rate, with as little as 30 minutes supervision. Even at such low supervision levels we do not observe a degradation of synthesis quality compared to a state-of-the-art baseline. Audio samples are available on the web1.
更多查看译文
AI 理解论文
溯源树
样例
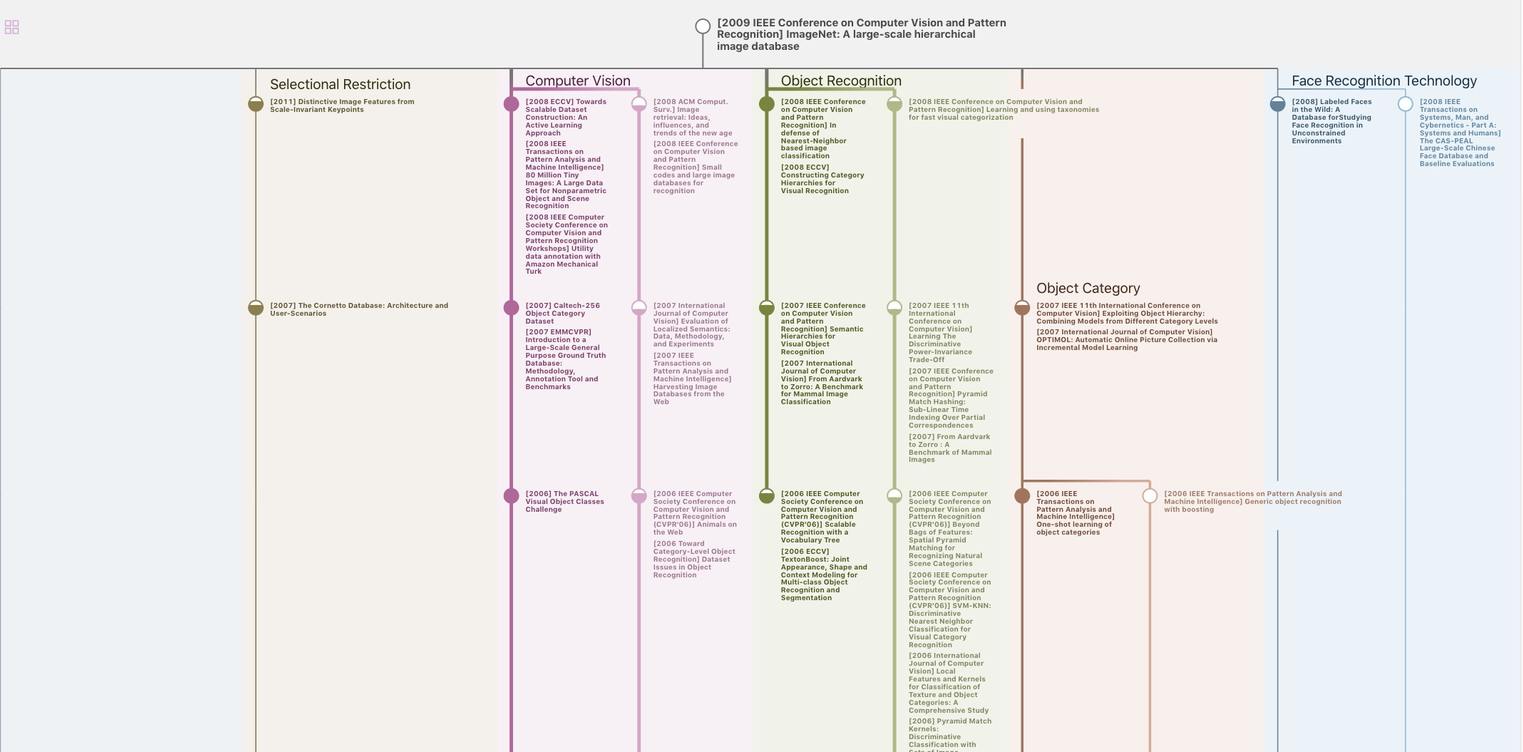
生成溯源树,研究论文发展脉络
Chat Paper
正在生成论文摘要