DC programming and DCA for parametric-margin ν -support vector machine
Applied Intelligence(2020)
摘要
As a development of ν -support vector machine ( ν -SVM), parametric-margin ν -support vector machine (Par- ν -SVM) can be useful in many cases, especially heteroscedastic noise classification problems. The present article proposes a novel and fast method to solve the primal problem of Par- ν -SVM (named as DC-Par- ν -SVM), while Par- ν -SVM maximizes the parametric-margin by solving a dual quadratic programming problem. In fact, the primal non-convex problem is converted into an unconstrained problem to express the objective function as the difference of convex functions (DC). The DC-Algorithm (DCA) based on generalized Newton’s method is proposed to solve the unconstrained problem cited. Numerical experiments performed on several artificial, real-life, UCI and NDC data sets showed the superiority of the DC-Par- ν -SVM in terms of both accuracy and learning speed.
更多查看译文
关键词
Support vector machine,Non-convex optimization,Generalized Newton’s method,DC programming,DCA
AI 理解论文
溯源树
样例
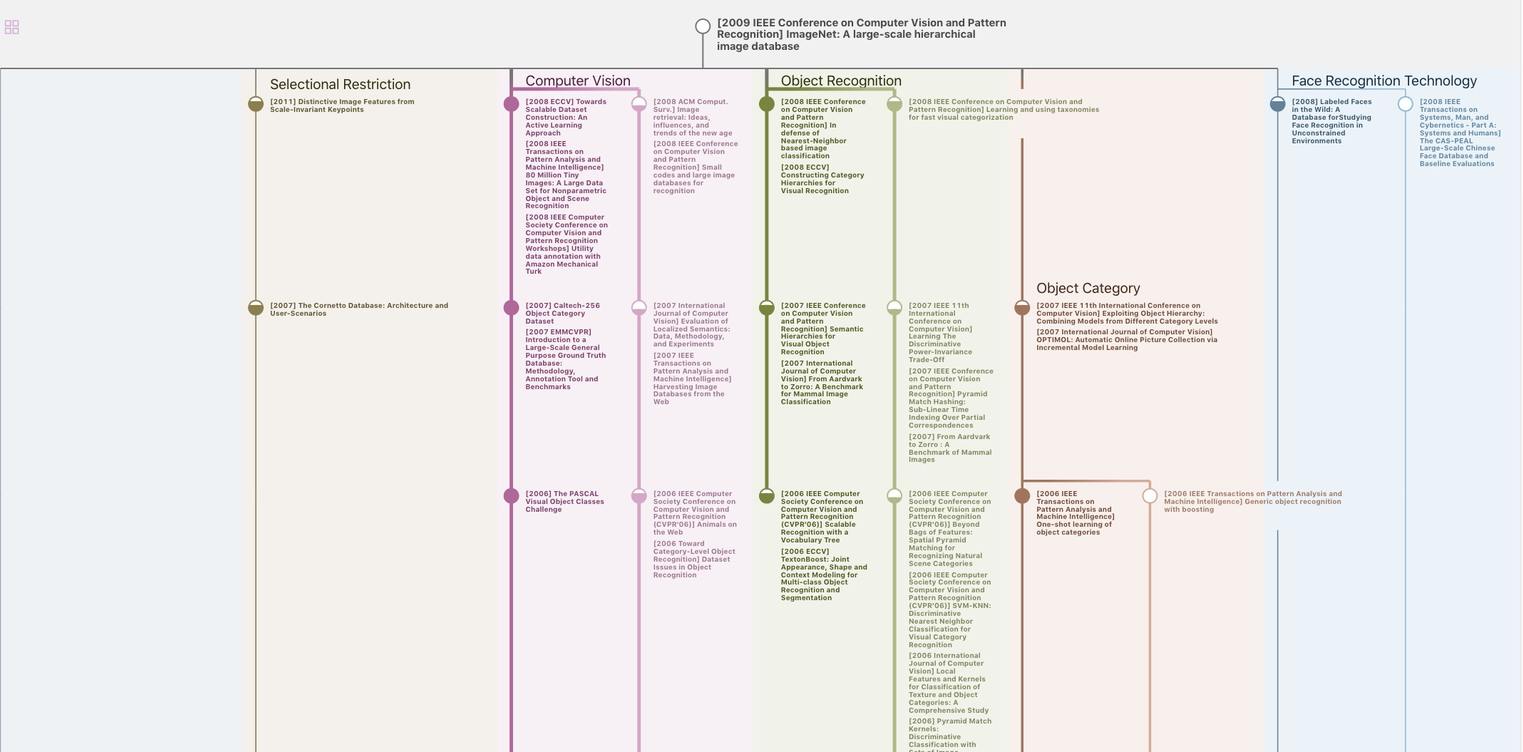
生成溯源树,研究论文发展脉络
Chat Paper
正在生成论文摘要