Improving movement prediction of traffic actors using off-road loss and bias mitigation
Workshop on’Machine Learning for Autonomous Driving’at Conference on Neural Information Processing Systems(2019)
摘要
There is a significant amount of recent literature in the domain of self-driving vehicles (SDVs), with researchers focusing on various components of the SDV system that have the potential to improve on-street performance. This includes work exploring improved perception of the SDV surroundings, or proposing algorithms providing better short-term prediction of nearby traffic actor behavior. However, in most cases, the authors report only aggregate metrics computed on the entire data, and often do not fully consider the bias inherent in the traffic data sets. We argue that this practice may not give a full picture of the actual performance of the prediction model, and in fact, may mask some of its problem areas (eg, handling turns). We analyze the amount of bias present in traffic data and explore the ways to address this issue. In particular, we propose to use a novel off-road loss and standard bias mitigation techniques that result in improved performance. We further propose to avoid aggregate metrics and instead analyze performance on relevant subsets of the data, thus better capturing actual model capabilities. Moreover, we propose to measure a novel off-road error to complement commonly used prediction metrics. Extensive analysis of real-world data suggests benefits of the proposed approach for improving the performance of SDV technology.
更多查看译文
AI 理解论文
溯源树
样例
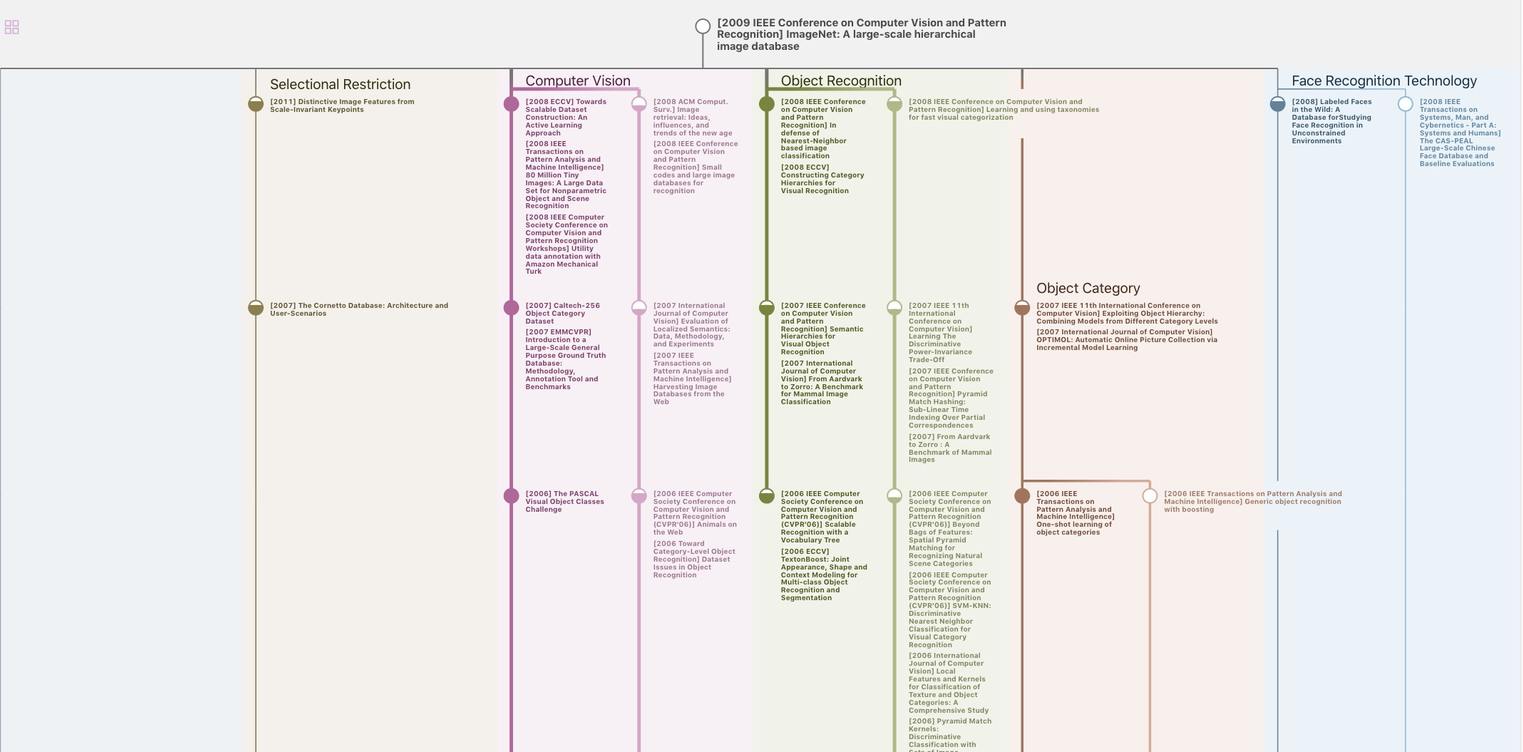
生成溯源树,研究论文发展脉络
Chat Paper
正在生成论文摘要