Exploring the evolution of GANs through quality diversity
GECCO(2020)
摘要
ABSTRACTGenerative adversarial networks (GANs) achieved relevant advances in the field of generative algorithms, presenting high-quality results mainly in the context of images. However, GANs are hard to train, and several aspects of the model should be previously designed by hand to ensure training success. In this context, evolutionary algorithms such as COEGAN were proposed to solve the challenges in GAN training. Nevertheless, the lack of diversity and premature optimization can be found in some of these solutions. We propose in this paper the application of a quality-diversity algorithm in the evolution of GANs. The solution is based on the Novelty Search with Local Competition (NSLC) algorithm, adapting the concepts used in COEGAN to this new proposal. We compare our proposal with the original COEGAN model and with an alternative version using a global competition approach. The experimental results evidenced that our proposal increases the diversity of the discovered solutions and leverage the performance of the models found by the algorithm. Furthermore, the global competition approach was able to consistently find better models for GANs.
更多查看译文
关键词
neuroevolution, coevolution, generative adversarial networks, quality diversity
AI 理解论文
溯源树
样例
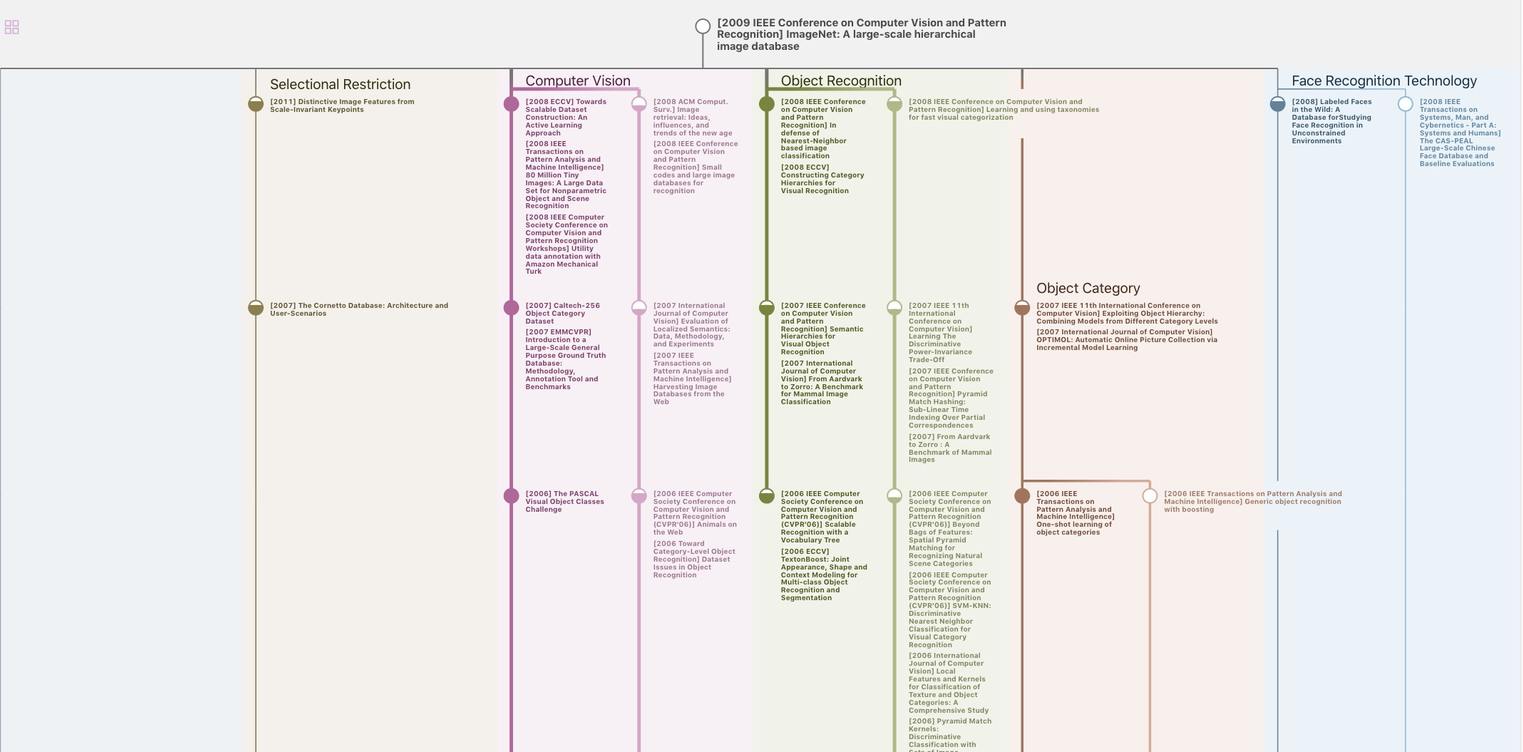
生成溯源树,研究论文发展脉络
Chat Paper
正在生成论文摘要