Quantitative Propagation of Chaos for SGD in Wide Neural Networks
NIPS 2020(2020)
摘要
In this paper, we investigate the limiting behavior of a continuous-time counterpartof the Stochastic Gradient Descent (SGD) algorithm applied to two-layer overpa-rameterized neural networks, as the number or neurons (i.e., the size of the hiddenlayer)N!+1. Following a probabilistic approach, we show ‘propagation ofchaos’ for the particle system defined by this continuous-time dynamics underdifferent scenarios, indicating that the statistical interaction between the particlesasymptotically vanishes. In particular, we establish quantitative convergence withrespect toNof any particle to a solution of a mean-field McKean-Vlasov equationin the metric space endowed with the Wasserstein distance. In comparison to previ-ous works on the subject, we consider settings in which the sequence of stepsizesin SGD can potentially depend on the number of neurons and the iterations. Wethen identify two regimes under which different mean-field limits are obtained,one of them corresponding to an implicitly regularized version of the minimizationproblem at hand. We perform various experiments on real datasets to validate ourtheoretical results, assessing the existence of these two regimes on classificationproblems and illustrating our convergence results.
更多查看译文
关键词
sgd,chaos,neural networks,propagation
AI 理解论文
溯源树
样例
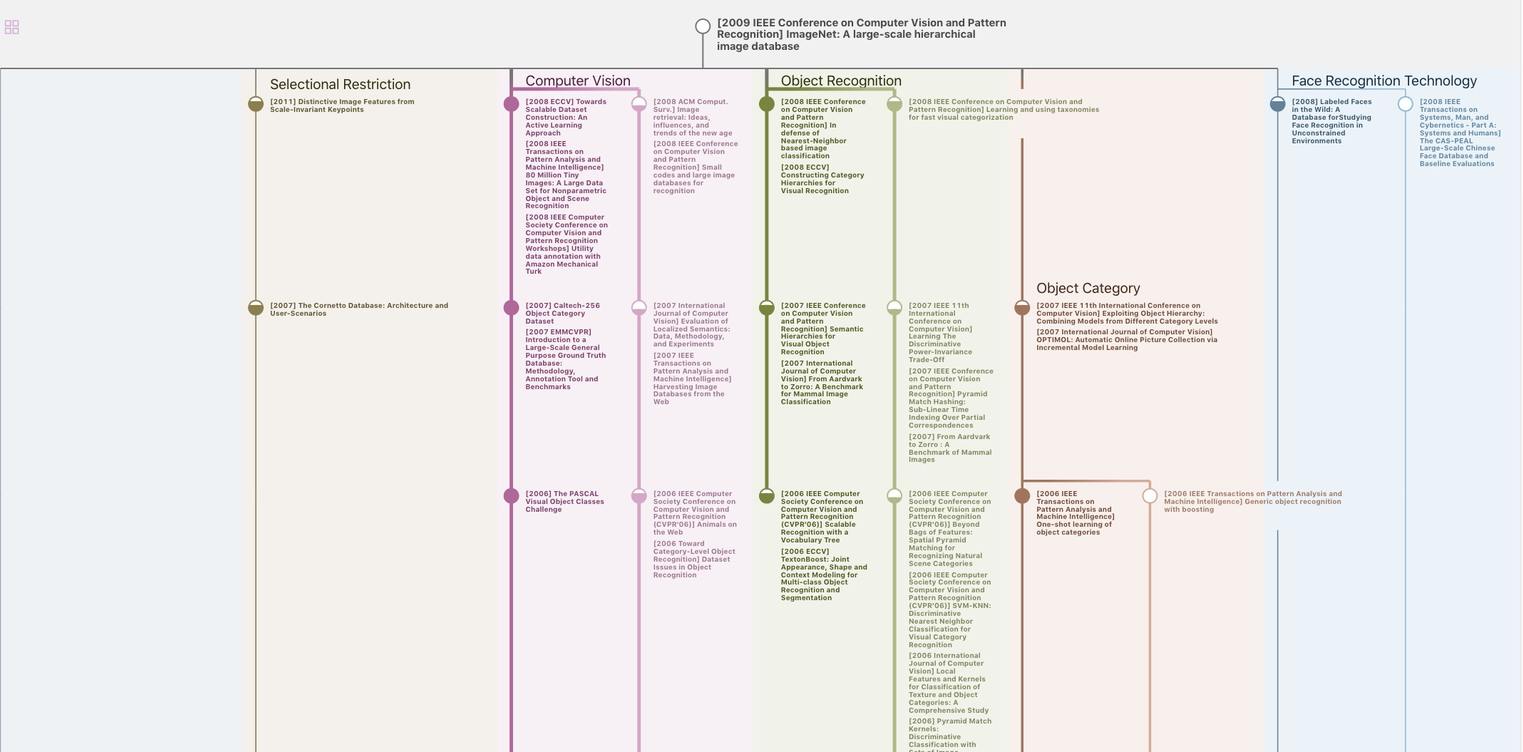
生成溯源树,研究论文发展脉络
Chat Paper
正在生成论文摘要