Nonexpansive Markov Operators and Random Function Iterations for Stochastic Fixed Point Problems
JOURNAL OF CONVEX ANALYSIS(2023)
摘要
We study the convergence of random function iterations for finding an invariant measure of the corresponding Markov operator. We call the problem of finding such an invariant measure the stochastic fixed point problem. This generalizes earlier work of the authors [Random function it-erations for consistent stochastic feasibility, Numer. Funct. Analysis Opt. 40/4 (2019) 386-420] studying the stochastic feasibility problem, namely, to find points that are, with probability 1, fixed points of the random functions. When no such points exist, the stochastic feasibility problem is called inconsistent, but still under certain assumptions, the more general stochastic fixed point problem has a solution and the random function iteration converges to an invariant measure for the corresponding Markov operator. We show how common structures in deterministic fixed point theory can be exploited to establish existence of invariant measures and convergence in distribution of the Markov chain. This framework specializes to many applications of current interest includ-ing, for instance, stochastic algorithms for large-scale distributed computation, and deterministic iterative procedures with computational error. The theory developed in this study provides a solid basis for describing the convergence of simple computational methods without the assumption of infinite precision arithmetic or vanishing computational errors.
更多查看译文
关键词
Averaged mappings,nonexpansive mappings,stochastic feasibility,inconsistent stochas-tic fixed point problem,iterated random functions,convergence of Markov chain
AI 理解论文
溯源树
样例
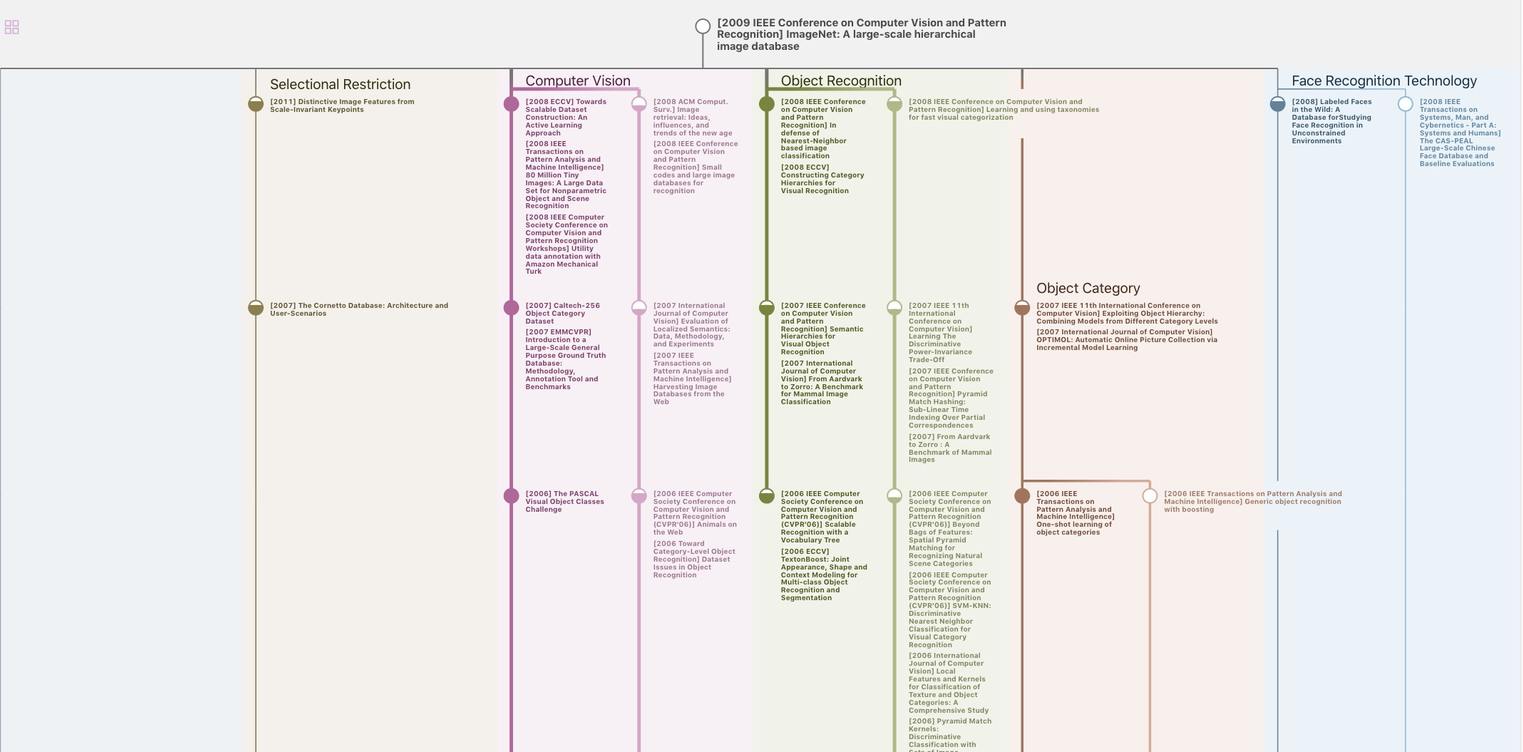
生成溯源树,研究论文发展脉络
Chat Paper
正在生成论文摘要