CrowdPic : A Flexible Approach for Optimized Data Selection and Aggregation in Mobile Crowd Photographing Applications
semanticscholar(2015)
摘要
Mobile Crowd Photographing (MCP) is attracting an emerging area of interest for researchers as cameras of mobile devices are becoming an indispensable visual logging device in people’s everyday lives. In order to meet diverse MCP application constraints/requirements (e.g. where and when to sense, sampling frequency, single or multiple shooting angles) to sensing targets, a multi-facet task model should be defined as a generic MCP data collection framework. Furthermore, MCP collects pictures in a distributed way in which a large number of contributors upload pictures whenever and wherever it is suitable. This inevitably leads to evolving picture streams. This paper investigates the multi-constraint-driven data selection problem in MCP picture aggregation, and propose a pyramid-tree (PTree) model which can efficiently select an optimal subset from the evolving picture streams based on varied coverage needs of MCP tasks. By utilizing the PTree model in a generic MCP data collection framework called CrowdPic, we test and evaluate the effectiveness, efficiency and flexibility of the proposed framework through both crowdsourcing-based and simulation-based experiments. Both the theoretical analysis and simulation results indicate that the PTree-based framework can effectively select a subset with high utility coverage and low redundancy ratio from the streaming data. The overall framework is also proved flexible and applicable to a wide range of MCP task scenarios.
更多查看译文
AI 理解论文
溯源树
样例
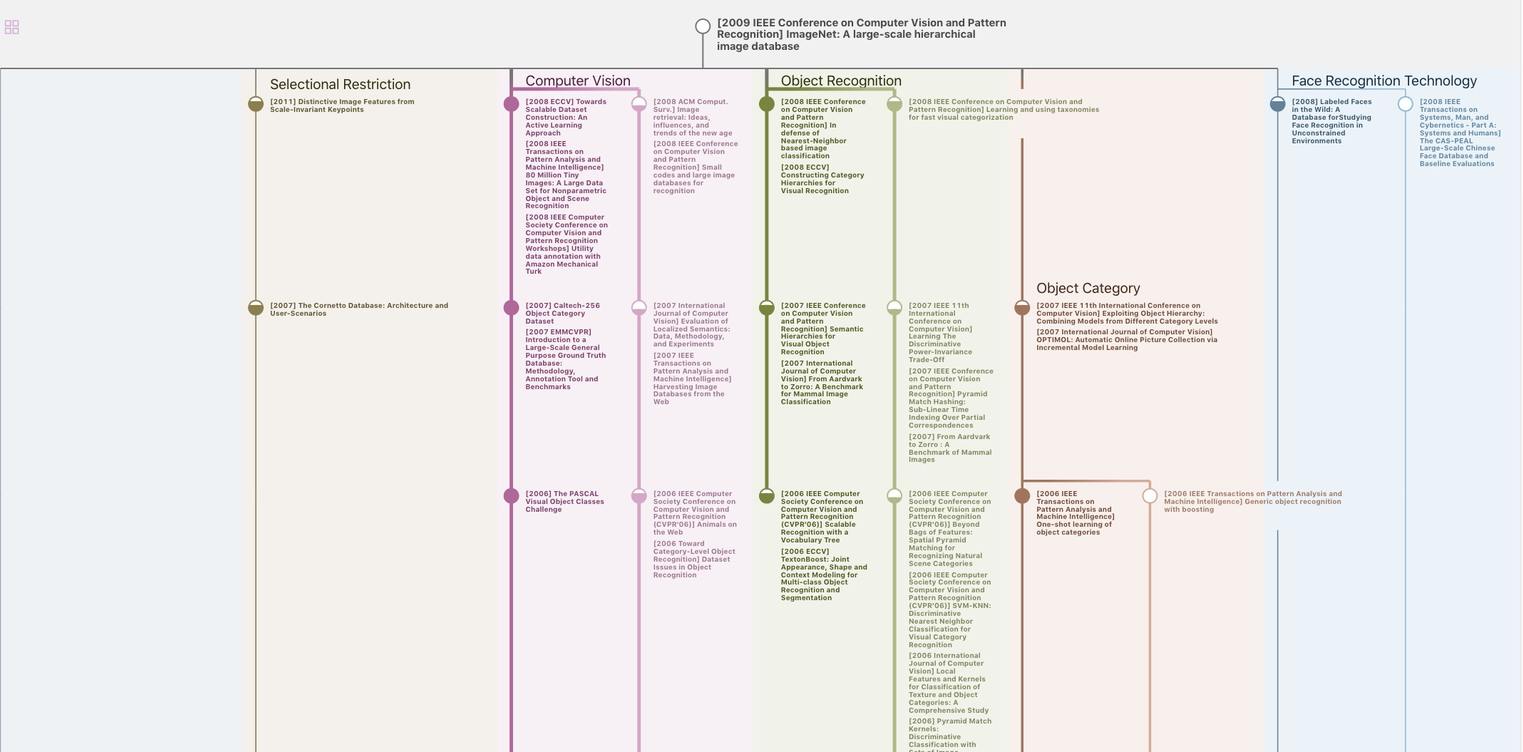
生成溯源树,研究论文发展脉络
Chat Paper
正在生成论文摘要