Pta-ftsm-2017-157 multi-level thresholding based on differential evolution-tsallis fuzzy entropy
semanticscholar(2018)
摘要
This paper presents a Multi level image Thresholding technique, using Tsallis entropy and fuzzy partition with a novel threshold selection technique. A global search algorithm is required for the calculation of optimal threshold values; a popular Meta heuristic algorithm Differential evolution is used successfully for solving this problem. One of the most critical tasks in image processing is Image segmentation; the proposed threshold selection technique is compared with Shannon entropy or fuzzy entropy , and Tsallis-Fuzzy entropy with existing threshold selection techniques, using over 10 images from popular image database of Berkeley as benchmark images, these images have been selected with each having a unique gray level histogram with important image quality metrics such as SSIM, PSNR, and SNR, furthermore for statistical analysis Friedman test values and average ranks have been calculated which establishes the superiority of the proposed threshold selection technique over other existing threshold selection techniques for image segmentation. A second group of dataset has been taken from [24] and comparison between the DE based proposed thresholding technique with patch-levy based bees algorithm based kapur entropy criterion and Otsu between-class variance criterion, on the basis of SSIM values has been conducted for the assessment of the image quality of the thresholds, also the CPU time elapsed for converging to the optimal threshold values has been compared to determine a faster algorithm. Standard deviation has been compared to give a measure of stability and robustness. For statistical significance Wilcoxon and Friedman tests have been conducted and p-value evaluated.
更多查看译文
AI 理解论文
溯源树
样例
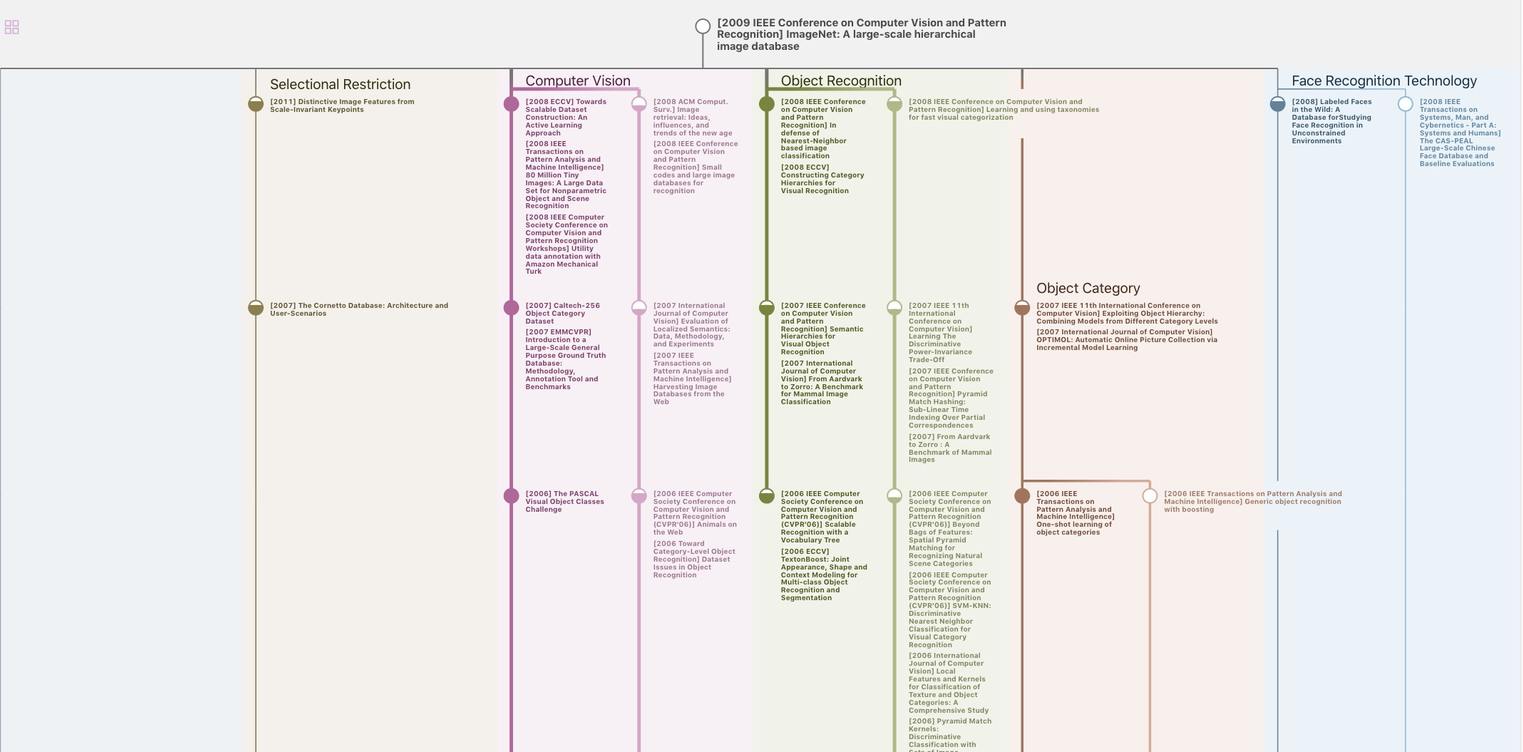
生成溯源树,研究论文发展脉络
Chat Paper
正在生成论文摘要