Clustering Longitudinal Data with Added Robustness through Cholesky-Decomposed Contaminated Gaussian Mixture Models
semanticscholar(2018)
摘要
In the last half century, advances in unsupervised machine learning approaches have made previously prohibitively computationally expensive classification problems rapidly solvable. When examining such a classification problem, many scientists will default to either a hierarchical clustering approach or the commonly applied k-means method (MacQueen, 1967). These approaches, based on distance-based measures of data similarity, frequently cannot differentiate irregularly shaped, sized, skewed, or intermixed groups within data. Unsupervised learning approaches based on distance alone may see no meaningful classification structure in such data, but in fact there may be a probabilistic or correlation-based model that can accurately sort these points; in such situations, an appropriate model-based clustering approach will prove far more effective. Mixture models assume that each group within the data is drawn from a underlying statistical distribution (i.e, they describe a mixture of models). The most frequent model used is a mixture of Gaussians, with the probability of a datapoint xi belonging to a specific Gaussian mixture model (GMM) having density
更多查看译文
AI 理解论文
溯源树
样例
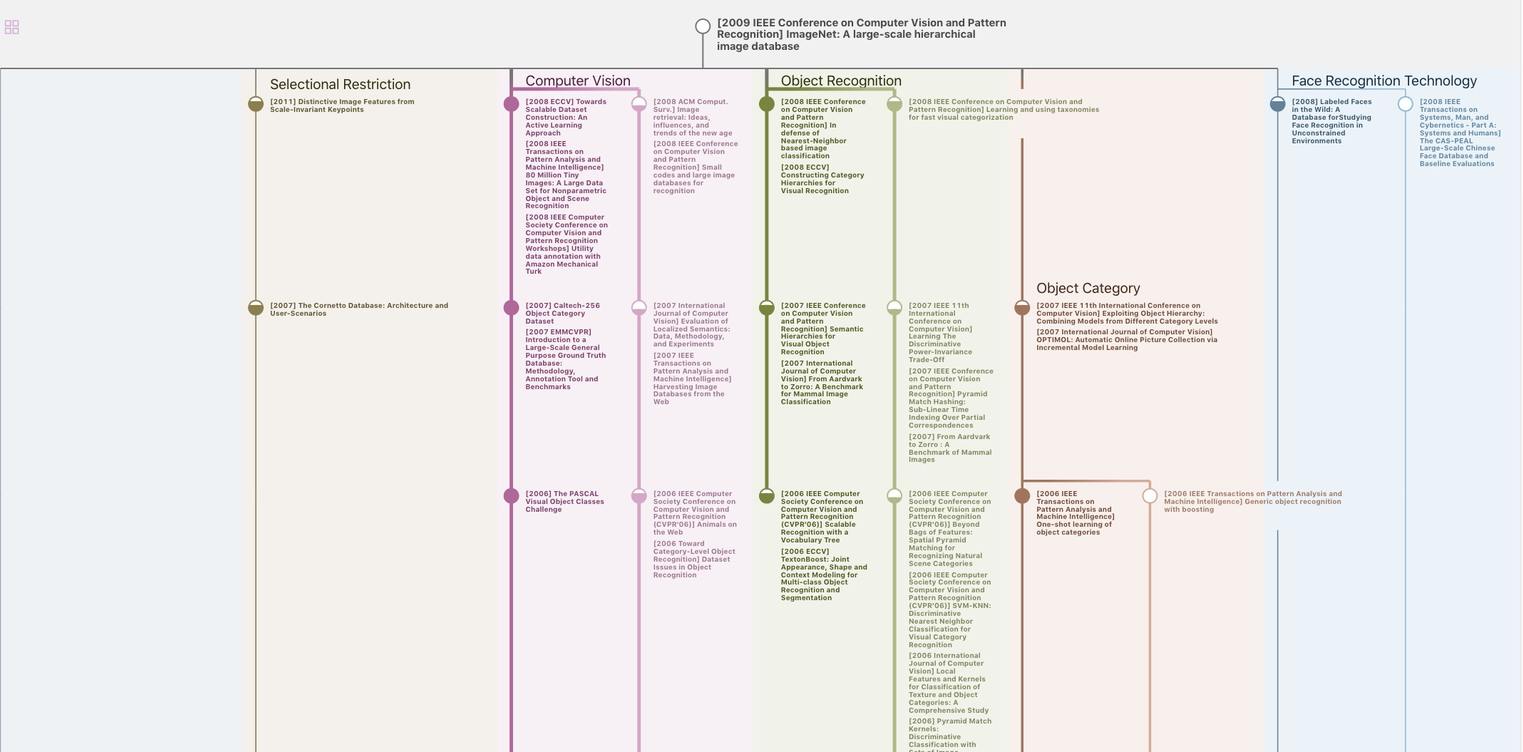
生成溯源树,研究论文发展脉络
Chat Paper
正在生成论文摘要