Activity recognition from body worn accelerometers-toward real-time event detection
semanticscholar(2017)
摘要
Inadequate physical activity is a leading risk factor in public health and inactive people are more vulnerable to having chronic diseases. In addition, levels of physical activity may be an indicator of health problems in elderly individuals, a particular problem in many societies where there is a growing ratio of elderly people. Identifying levels of physical activity may have a significant effect on reducing healthcare costs in the future. As a consequence, finding approaches for measuring the individuals’ activities is a persistent need, in order to provide a view about their quality life and to observe their current health status. This may be best achieved by using low-cost wearable technologies such as accelerometer based inertial sensors. In this work, accelerometer data was projected onto a sphere of radius g (magnitude of acceleration due to earth gravitate) and the angle between the posture of the individual’s trunk and the gravity vector was used together with the dot product of two successive accelerations to extract significant features from accelerometer data. Two datasets were used, the first had been collected for SPHERE project from individuals with Parkinson’s disease in their home and the second is a public domain benchmark data set. Decision Trees and Naïve Bayes classifiers were used on both datasets. The classification results of a small set of activities of single individual from first dataset show that Naïve Bayes have a higher overall accuracy rate, which is 85.41% compared with 78.56% for Decision Trees. The second dataset of daily household activities is used to provide a comparison with one state-of-the-art approach in the literature. The result shows that Decision Trees with the proposed features outperform the literature approach by having an overall accuracy rate exceeded 91%. 1 Biomedical Engineering group, School of Biological Sciences, University of Reading, Reading RG1 5AQ, UK 2 Department of Computer Systems, Babel Technical Institute, Al-Furat Al-Awsat Technical University, Babylon, Iraq 3 Faculty of Health Sciences, University of Southampton, Southampton SO17 1BJ, UK 4 NIHR CLAHRC Wessex, University of Southampton, Southampton SO17 1BJ, UK 5 Faculty of Engineering, University of Bristol, Bristol, BS8 1UB, UK
更多查看译文
AI 理解论文
溯源树
样例
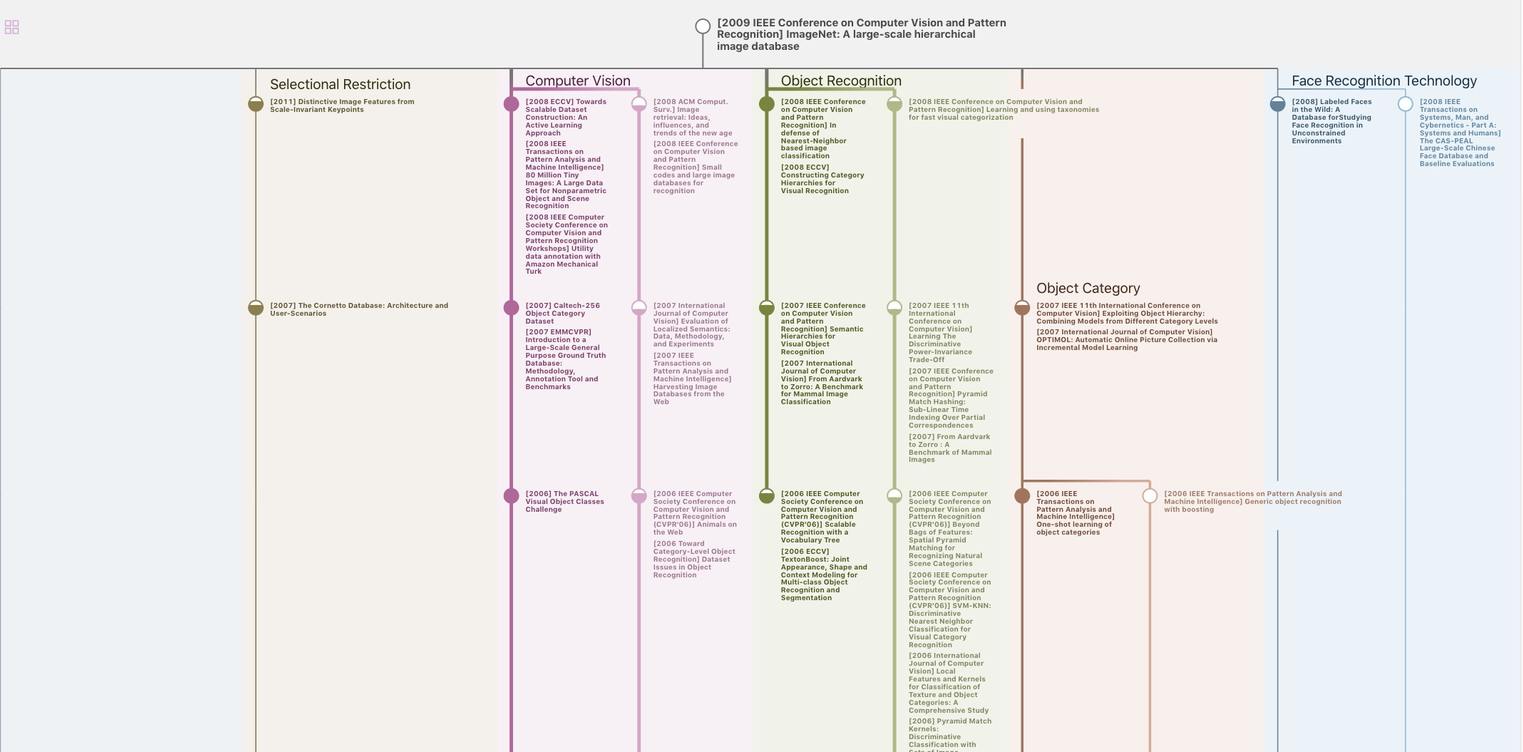
生成溯源树,研究论文发展脉络
Chat Paper
正在生成论文摘要