A method for extreme data reduction of Acoustic Emission ( AE ) data with application in machine failure diagnosis
semanticscholar(2013)
摘要
Abstract The use of AE in machine failure diagnosis has increased over the last years. Most AE-based failure diagnosis strategies use digital signal processing and thus require the sampling of AE signals. High sampling rates are required for this purpose (e.g. 2 MHz), which leads to streams of large amounts of data. This situation is aggravated if fine resolution and/or multiple sensors are required. These facts combine to produce bulky amounts of data –typically in the range of GBytes,– for which sufficient storage space and efficient signal processing algorithms are required. This situation probably explains why, in practice, AE-based methods consist mostly in the calculation of scalar quantities such as RMS, Kurtosis, etc., and the analysis of their evolution in time. While the scalar-based approach offers the advantage of maximum data reduction; it has the disadvantage that most part of the information contained in the raw AE signal is lost unrecoverably. This work presents a method offering extreme data reduction, while keeping the most important information conveyed by the raw AE signal, which is useful for failure diagnosis purposes. The proposed method is very simple: it consist on the construction of a synthetic, unevenly sampled signal which envelopes the AE bursts present on the raw AE signal in a triangular shape. The constructed signal –which we call TriSignal– also permits the estimation of most scalar quantities typically used. But more importantly, it contains the information of the time of occurrence of the bursts, which is key for failure diagnosis. Furthemore, we show how the TriSignal can be used to compute the TriSpectrum, an estimator of the frequency content of the AE envelope.
更多查看译文
AI 理解论文
溯源树
样例
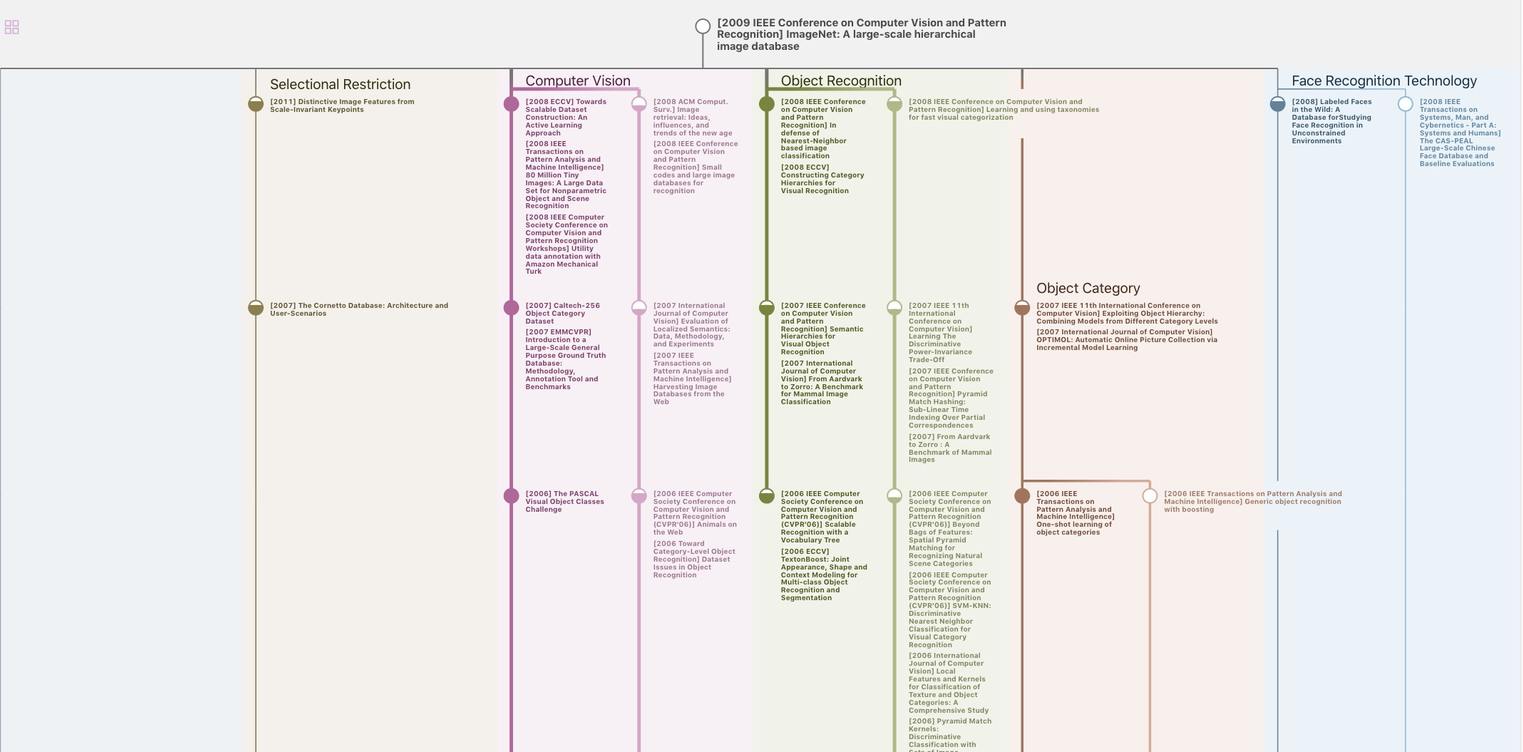
生成溯源树,研究论文发展脉络
Chat Paper
正在生成论文摘要